A. Seifan, R. Isaacson
Department of Neurology, Division of Memory Disorders, Weill Cornell Medical College / New York-Presbyterian Hospital, New York, NY, USA
Corresponding Author: R. Isaacson, MD, Weill Cornell Medical College, 525 E. 68th Street, F-610, New York, NY, 10065, USA. Phone 212-746-0226. Email: rii9004@med.cornell.edu
J Prev Alz Dis 2015;2(4):254-266
Published online October 1, 2015, http://dx.doi.org/10.14283/jpad.2015.81
Abstract
In July 2013, Weill Cornell Medical College founded the first Alzheimer’s Prevention Clinic (APC) in the United States, providing direct clinical care to family members of patients with Alzheimer’s disease (AD) as part of the Weill Cornell Memory Disorders Program. At the APC, patients seeking to lower their AD risk undergo a comprehensive assessment, receive a personalized plan based on rapidly evolving scientific evidence, and are followed over time using validated as well as emerging clinical and research technologies. The APC approach applies the principles of pharmacogenomics, nutrigenomics and clinical precision medicine, to tailor individualized therapies for patients. Longitudinal measures currently assessed in the clinic include anthropometrics, cognition, blood biomarkers (i.e., lipid, inflammatory, metabolic, nutritional) and genetics, as well as validated, self-reported measures that enable patients to track several aspects of health-related quality of life. Patients are educated on the fundamental concepts of AD prevention via an interactive online course hosted on Alzheimer’s Universe (www.AlzU.org), which also contains several activities including validated computer-based cognitive testing. The primary goal of the APC is to employ preventative measures that lower modifiable AD risk, possibly leading to a delay in onset of future symptoms. Our secondary goal is to establish a cohort of at-risk individuals who will be primed to participate in future AD prevention trials as disease-modifying agents emerge for testing at earlier stages of the AD process. The clinical services are intended to lower concern for future disease by giving patients a greater sense of control over their brain health.
Key words: Alzheimer’s prevention clinic, Alzheimer’s prevention education, Alzheimer’s prevention clinical research, personalized medicine, clinical precision medicine.
Introduction
Neuropathological brain changes related to Alzheimer’s Disease (AD) begin in healthy, community-dwelling individuals many years, if not decades, prior to symptom onset, a condition known as “Preclinical AD”, or “asymptomatic at risk for AD” (1). In such individuals, interventions delivered at midlife or other specific points in the lifespan could potentially slow or delay the cognitive changes which eventually affect a significant proportion of seniors across the globe and undermine their ability to live independently (2). A host of biomarkers to diagnose Preclinical AD are already available, at least on a research basis, and these biomarkers continue to improve (3). Also, several validated risk scores are available which enable cost-effective screening to identify individuals likely to show biomarker evidence of Preclinical AD (4).
Despite the increasing availability of sophisticated tools to diagnose Preclinical AD, and despite the growing evidence that the onset of dementia due to AD may be preventable, the clinical applicability to people at risk for AD remains fraught with challenges. Robust criteria for clinical diagnosis of Preclinical AD, using commonly available tools (without the use of research-based biomarkers), have yet to be developed. Also, determining the effects, ideal timing, costs and potential risks of behaviors and disease-modifying interventions throughout the lifespan represent important aims of current and future prevention research (5-7). The potential stress-inducing effects of receiving a diagnosis of Preclinical AD remain to be studied over the long-term (8). The natural history of Preclinical AD in individuals with atypical neurodevelopment remains uncharacterized. To answer these and other important questions, AD prevention trials will require large cohorts of asymptomatic subjects of various ages, followed over many years, assessed within multiple domains (9). Dementia prevention is indeed possible and has become a priority for nations across the globe, as evidenced by a recent report emanating from the G8 dementia summit in December 2013 which was supported by several international experts (2).
In July 2013, Weill Cornell Medical College founded the first Alzheimer’s Prevention Clinic (APC) in the United States (U.S.), providing direct clinical care to family members of patients with AD as part of the Weill Cornell Memory Disorders Program. At the APC, patients seeking to lower their AD risk undergo a comprehensive assessment, receive a personalized plan based on the rapidly evolving scientific evidence, and are followed over time using validated as well as emerging clinical and research technologies. The APC approach applies the principles of pharmacogenomics, nutrigenomics and clinical precision medicine, to tailor individualized therapies for patients. Longitudinal measures currently assessed in the clinic include anthropometrics, cognition, blood biomarkers (i.e., lipid, inflammatory, metabolic, nutritional) and genetics, as well as validated, self-reported measures that enable patients to track several aspects of health-related quality of life. The primary goal of the APC is to employ preventative measures that lower modifiable AD risk, possibly leading to a delay in onset of future symptoms. Our secondary goal is to establish a cohort of at-risk individuals who will be primed to participate in future AD prevention trials as disease-modifying agents emerge for testing at earlier stages of the AD process. The clinical services are intended to lower concern for future disease by giving patients a greater sense of control over their brain health.
The APC Conceptual Framework
In concordance with best practices for preventive care, the foundation of our approach is our educational program, which delivers both online and local educational interventions intended to maximize public awareness, engagement and adherence. Heightening of public awareness and improving access to resources are U.S. priorities for reducing the burden of AD (see The Healthy Brain Initiative: The Public Health Road Map for State and National Partnerships 2013–2018 and the National Plan to Address Alzheimer’s Disease). Raising public awareness and enhancing public engagement could lead to earlier identification of at-risk individuals, faster diagnoses, better stratification or higher enrollment in dementia prevention trials, and ultimately to more effective preventative treatments (10). Patient education not only promotes earlier identification of at-risk individuals, but also forms the foundation by which preventive health behaviors later ensue (11, 12). In turn, health behaviors influence biomarkers, brain structure, brain function and quality of life. Interrelationships between modifiable and non-modifiable factors during early-brain development, and throughout the lifecourse, are essential to consider. See Figure 1 for the Weill Cornell APC Conceptual Framework.
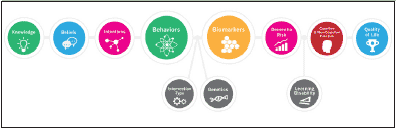
Figure 1. The Weill Cornell Medical College / NewYork-Presbyterian Hospital Alzheimer’s Prevention Clinic Conceptual Framework
Applying the Conceptual Framework
For each patient, each factor in the continuum from education to behavior to quality of life is systematically assessed at baseline and longitudinally using validated, standardized measures. Table 1 lists each domain of assessment with the corresponding measures by which each factor is assessed. All measures were chosen based on extensive literature review of best available measures. In choosing the measures, we prioritized those which were the most practical to deliver in a clinical setting and those which had the most evidence.
*The majority of these are measured routinely, with a minority of biomarkers being tested per individual case.
Figure 2 summarizes the cycle of assessment from the patient’s perspective. Prior to the initial visit, patients are emailed a comprehensive self-assessment which aids the physician to tailor the evaluation to the patient’s individual values. This assessment uses a customized collection of validated measures to assess many of the domains listed in the conceptual framework, including early life educational history, general knowledge about AD, willingness to engage in specific behaviors to prevent AD (including genetic testing preferences and lifestyle behaviors), concern and fear about AD, awareness of personal AD risk factor status, as well as measures to longitudinally assess quality of life in the domains of cognition, mood, motor, sleep, work and social structure. Prior to the first visit, patients are also encouraged to complete an interactive course about Alzheimer’s prevention which is hosted on Alzheimer’s Universe (www.AlzU.org). This website provides a technological platform to offer current and comprehensive evidence-based educational content, while also providing the tools necessary to study their effectiveness, such as the Alzheimer Prevention Knowledge Scale (APKS) (13), and AD-Nutrition Tracking System (AD-NTS) (14), which records longitudinal lifestyle patterns and can be used to evaluate outcomes. AlzU.org has been shown to significantly improve medical knowledge about AD prevention and improve willingness to participate in an AD prevention clinical trial (15). AlzU.org also contains a number of activities, including the option to take online cognitive tests which may be particularly useful for detecting and longitudinally assessing prodromal pathological changes due to AD (i.e., Cognitive Function Test (16) and Neurotrack (17)) in an easily accessible online format. Currently, AlzU.org provides 6 evidence-based interactive lessons (~10 minutes each) and 12 activities (e.g. referral to other online AD initiatives such as endalznow.org and brainhealthregistry.org; clinical trial referrals including A4; US Against Alzheimer’s referral; and user discussion forums). A token economy system was employed to promote lesson completion, which includes periodic awards for the completion of core milestones, as well as “brain-health” points awarded for each task finished. The completion of comprehensive, self-reported assessments prior to the physician visit enables better personalization of the visit to the patient’s unique background; online education prior to the visit enables a richer discussion during the counseling portion of the visit.
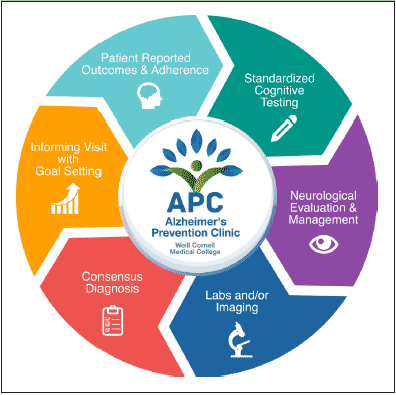
Figure 2. Summary of the cycle of longitudinal patient assessment in the Alzheimer’s Prevention Clinic
Upon arriving to the clinic, patients complete approximately 1 hour of standardized cognitive testing, which includes relevant elements from the NIH Toolbox Cognition Battery, the CogState Brief Battery, and traditional neuropsychological tests sensitive to early stages of dementia (language fluency, Trails B). Patients also undergo standardized smell testing using the NIH Toolbox Brief Odor Identification Test (18). After cognitive testing, patients undergo neurological evaluation and clinical management, including a thorough neurological exam, medical history, review of potential prodromal neurodegenerative symptoms, and review of medications. During the visit, patients’ survey answers are reviewed and confirmed in person by the physician (appointment time up to 90 minutes).
After the visit, patients identify their personal goals, using willingness scales that incorporate Stages of Change theory (19). Prior to their next follow-up, patients report their adherence to their stated goals using an adapted version of a previously validated adherence scale used in the Medical Outcomes Study (20). In some cases where medication is prescribed, adherence is also assessed using prescription refill rates via the electronic medical record (EMR).
The Consensus Diagnostic Process
Although biomarker-based criteria are available for diagnosing Preclinical AD, no criteria have been formally developed to clinically diagnose individuals who are in prodromal stages of AD or other dementias. At the APC, we have drafted a preliminary diagnostic schema to aid clinicians in detecting patients with no or minor memory complaints who may be at higher risk for neurodegenerative disease, or already in Preclinical AD (See Table 2 and 3). These criteria highlight more refined patient classifications that otherwise would be classified as “normal”. We classify patients who have no significant cognitive symptoms/findings as Normal with Low, Moderate or High Risk, using commonly available risk indices for cerebrovascular disease and AD. Specifically, Normal-High Risk patients are those who we predict to already be biomarker positive (i.e., Stage 1/Stage 2 Preclinical AD). Normal-Moderate Risk patients are those with a significant family history of AD with any number of modifiable risk factors (122), yet we predict that they are AD biomarker negative. Normal-Low Risk patients are those with no significant family history of AD with no or few modifiable risk factors.
AD = Alzheimer’s Disease; PD = Parkinson’s Disease; ND = Neurodegenerative Dementia; DCI = Detectable Cognitive Impairment; SN = Synucleinopathy; DLB = Dementia with Lewy Bodies; Atypical ND = Primary Progressive Aphasia, Posterior Cortical Atrophy, Dysexecutive Predominant AD, Frontotemporal Dementia, and CBD/PSP; SCI = Subjective Cognitive Impairment
After Normal, the next diagnostic category is termed detectable cognitive impairment (DCI), which is modeled after the criteria used to diagnose MCI-AD (68). The category of DCI helps to identify and categorize patients with objective cognitive deficits but who do not meet criteria for MCI. They may or may not have associated risk factors or subjective cognitive complaints. We then attempt to classify DCI patients as either DCI due to neurodegenerative disease (ND) or DCI not otherwise specified (DCI-NOS), based solely on the presence or absence of non-cognitive symptoms which have been robustly identified in the literature to be associated with any ND (70).
Once classified as DCI-ND, we attempt to predict the underlying pathology. We stratify DCI-ND as DCI due to typical AD pathology (DCI-AD), DCI due to synucleinopathy (DCI-SN) and DCI due to Atypical ND (DCI-AND). This last category includes patients with expected prodromal Posterior Cortical Atrophy, Primary Progressive Aphasia, Frontotemporal Lobar Degeneration, or other tauopathies). To distinguish among different predicted pathologies, we integrate the evidence regarding cognitive, mood, motor, sleep, and family history features that are specific to different ND. For example, distinguishing features in DCI-AD include presence of amnestic-type memory changes, sleep inefficiency, late-life mood changes (i.e., irritability, depression), and slowed walking (without Parkinsonism). Distinguishing features in DCI-SN (such as from Parkinson’s disease or Dementia with Lewy Bodies) are primarily non-amnestic cognitive deficits (e.g., bradyphrenia, poor learning, logopenia), REM sleep behavior disorder, features of autonomic disturbance (e.g., constipation, orthostatic hypotension) and early-life anxiety disorders. DCI-AND features include non-amnestic cognitive changes, history of atypical development (dyslexia, dyscalculia, dysgraphia, stuttering, etc.) and/or other atypical factors (muscle atrophy, myoclonus, dystonia, gaze paralysis).
While preliminary, these draft criteria allow our multidisciplinary group of practicing dementia clinicians to classify patients at various levels of risk for ND based purely on clinical history, examination and freely available, standardized measures. Further research will be aimed at identifying the sensitivity and specificity of these consensus diagnoses with respect to verified AD and other ND biomarkers.
Individuals who are already in MCI or dementia stages are diagnosed using the latest consensus criteria for Mild Cognitive Impairment (17), Alzheimer’s Disease (19), Dementia with Lewy Bodies / Parkinson’s Disease Dementia (71), Corticobasal Degeneration (72), Primary Progressive Aphasia (20), and Frontotemporal Dementia (73).
Evidence-Based Interventions
The APC approach is to individualize all patient recommendations such that individual patient values, preferences, and genetics are taken into account. Precision medicine is a medical model that proposes the customization of healthcare—with decisions tailored to individual patients. In this model, diagnostic testing is often employed for selecting appropriate and optimal therapies based on the context of a patient’s genetic risk or other molecular or cellular analysis (78).
A principal hypothesis underlying our approach toward AD risk reduction is that glucose hypometabolism, especially in the context of modifiable AD risk factors, promotes further AD brain pathology. As such, targeted interventions to address distinctive nutritional, and/or metabolic requirements in the AD brain should support mitochondrial efficiency and reduce oxidative damage, thereby contributing to neuroprotection (79, 80). At the first follow-up visit, the treating physician and patient collaboratively review their diagnostic workup, including labs and imaging (appointment time approximately 60 minutes). Any potentially modifiable risk factors are identified, and targeted interventions to address those risk factors are discussed.
Table 4 summarizes potential interventions to address risk factors which are carefully tailored for each individual patient. All patients receive nutritional counseling for lowering cardiovascular and AD risk. For patients with any evidence of adipose-tissue insulin resistance who are already living a healthy lifestyle, aggressive treatment with medication is discussed. This is important because in our healthy adults at higher genetic risk for AD, traditional risk factors might otherwise not be identified or treated as aggressively during midlife. Also, for patients who are already taking hypertensive medications, consideration is given to switching to medications with the most evidence for potential to delay cognitive decline (angiotensin receptor blockers and calcium channel blockers). Other lifestyle interventions include suggestions to boost cognitive reserve (i.e., musical training (81)), to promote self-management of cardiovascular health (at-home anthropomorphic testing (82)), increase social engagement (83), and to teach stress reduction techniques (i.e., meditation (84) and cognitive behavioral therapy (85)).
CCB = calcium channel blocker; ARB = angiotensin receptor blocker
APC Specific Research Programs
The Comparative Effectiveness Dementia & Alzheimer’s Registry (CEDAR) Project
The CEDAR Project is the primary registry for all patients presenting for memory loss prevention or treatment due to AD, other neurodegenerative dementias, or other causes. All patients presenting to the clinic have the opportunity to donate their de-identified clinical data to the registry. The primary aim of the registry is to establish a well-characterized cohort of individuals with or at risk for AD or other dementias who will be primed to benefit from preventative strategies and disease-modifying interventions expected to continue to emerge in the coming decade.
The Dietary Intervention to Slow Memory Loss due to Alzheimer’s (DIPLOMA) Study
This study is a prospective, observational feasibility and pilot study of patients receiving nutritional and lifestyle counseling as part of routine clinical care at the APC. Nutritional and other lifestyle interventions have been shown to delay the onset of cognitive decline in controlled trials. These interventions may be most effective in patients with preclinical AD, prior to the onset of neuronal loss. However, no formal clinical criteria currently exist to classify individuals with preclinical AD without the use of expensive biomarker testing. Moreover, the effectiveness of nutritional interventions in real-world clinical, as opposed to controlled settings, has not been demonstrated.
The overall, long-term objective of the DIPLOMA study is to better identify and predict individuals most likely to benefit from nutritional interventions. The short-term objectives are to validate a clinical diagnostic algorithm and assess the comparative effectiveness of the DIPLOMA intervention in subjects with unique medical and genetic backgrounds. We hypothesize that adherence to the APC intervention improves brain metabolism and slows cognitive decline via changes in metabolic risk factors of AD. Eligible patients will include persons aged 30 to 85 who are enrolled in CEDAR, have at least one genetic factor consistent with Alzheimer’s risk (either APOE4 or first degree family history), and have at least one modifiable nutritional risk factor (adipose tissue peripheral insulin resistance, low B12, low vitamin D, low omega 3, poor diet, overweight, or elevated homocysteine). In the future, nutritional and lifestyle interventions could form the basis for disease prevention counseling in early to midlife for adults at risk for AD.
Development and Validation of Alzheimer’s Universe (www.AlzU.org), a Web-based Program for Alzheimer’s Disease Education and Prevention
In this study, we are developing and validating scales that measure the key knowledge and belief constructs known to influence care-seeking behaviors related to Alzheimer’s prevention. There is a lack of knowledge about the comparative effectiveness of different dissemination strategies to facilitate the use of health-related evidence (108). This is driven largely by a lack of tools to accurately measure knowledge and beliefs about neurodegenerative diseases. The Center for Disease Control plans to identify validated survey questions that can be used at the national, state, and local levels to track awareness and perceptions about cognitive health (109). Using AlzU.org, we will test whether these scales can predict behavioral intent, as measured by actual behaviors such as scheduling an appointment to see an Alzheimer prevention doctor, adhering to doctor recommendations, or participating in a prevention trial. Although prior studies have addressed differences in motivating factors for behavioral intent related to AD, studies have not attempted to elucidate the differences in the belief mechanisms responsible for behavioral change. Ultimately, accurate measurement of knowledge and health belief constructs may enable more accurate public health surveillance of AD awareness and attitudes. Validated, online tools such as these may also play a role in better defining strategies to increase recruitment into Alzheimer’s prevention research trials. Our ultimate goal is to improve patient outcomes through education about risk factor modification and resultant health-promoting behavioral change.
Specific Learning Disabilities and Atypical Alzheimer’s Disease
This is an observational, prospective study of clinical care delivered to learning-disabled adults who present for dementia prevention or treatment. The reasons why a subset of individuals with AD presents with atypical symptoms at onset (vision or language, rather than memory) are unclear, but may relate to presence of a childhood learning disability. This is a critical research gap because a better understanding of clinical heterogeneity in AD could lead to clues related to the primary pathogenesis of AD. Strategies for early detection, prevention or treatment may need to be different for people with Atypical AD (110). The overall, long-term goal of this project is to further understand the differences in risk factors, outcomes and effectiveness of health care for memory loss patients with and without specific learning disabilities (LD). Primary aims include validation of a patient-reported questionnaire, adapted from prior validated questionnaires (64-66), to detect history of childhood LD in adults presenting for dementia prevention or treatment, as well as characterization of differences in cognition and disease progression in patients with vs. without LD who present for dementia prevention. We hypothesize that areas of the brain that are last to connect during early life, as affected in LD, could be the first to degenerate when amyloid accumulation begins in the brain, leading to Atypical phenotypes of AD. This group of adults represents a significant proportion of the population (5-10%) who has thus far been underrepresented in dementia research. Ultimately, this line of research could identify a new risk factor for Atypical AD, which might lead to clues about the cause of AD (which remains unknown) and to the development of preventative interventions for atypical dementias, which are currently unavailable.
Ethical Considerations
Clinical implementation of precision medicine for Alzheimer prevention is complicated by several ethical, legal and psychosocial implications (111). These include potential for adverse psychological impact related to risk disclosure, and potential for social stigma or discrimination by employers or long-term care or life insurance companies. Despite these concerns, the best available evidence suggests that blinded enrollment of patients into an Alzheimer prevention study (preventing disclosure of Alzheimer’s risk), at least from an ethical standpoint, is not necessary (112) and also that disclosure of genetic status is without significant adverse psychological impact (113). Regarding potential for discrimination, in the United States, the Genetic Information Nondiscrimination Act (GINA) prevents health insurance companies from considering genetic information when setting premiums; however, GINA does not prevent life, disability or long-term care insurances from using genetic testing information. In the context of clinical care, physicians and patients may decide together to keep genetic data separate from a patient’s medical record (for example if the patient perceives potential risks of breach of confidentiality to outweigh the benefits of complete documentation) (114). Also of note is the fact that risk disclosure may facilitate adherence to physician recommendations related to lifestyle but may also cause unnecessary or undesirable behavior change such as increased likelihood of taking supplements with no clear beneficial effect (115, 116).
To mitigate against these concerns, and in accordance with best practices for testing in prodromal neurological disease (117), the APC implements the following policies: All patients’ genetic test results inform the pharmacogenomics and/or nutrigenomics care plan (118), including recommendations related to lifestyle changes, medications, long-term care planning and participation in research trials. All patients are provided extensive pre-visit educational tools, and undergo routine psychological screening to identify patients at high risk of adverse psychological outcomes. All patients also receive pre-test genetic counseling and have access to post-test counseling. Patients are encouraged to consider long-term care, disability and life insurance prior to obtaining genetic testing. Although there is no definitive prohibition, most insurers do not use APOE genotyping to determine long-term care insurance eligibility or to set premiums (119). In some states, insurers are actually required to obtain consent prior to accessing genetic data (120).
A Global Effort
As of mid-2015, only a few clinical programs exist worldwide which offer dementia prevention services outside of a strictly research setting. To our knowledge, in the United States, these programs include the Weill Cornell Medical College / NewYork-Presbyterian Hospital APC, the University of Alabama – Birmingham Alzheimer’s Risk Assessment and Intervention Clinic, and the Cedars-Sinai Medical Center Alzheimer’s Prevention Program. The information for making an appointment at any one of these programs is listed on www.AlzU.org (under the ‘See a Doctor’ activity). The APC, via AlzU.org, has also established collaborations with multiple institutions involved in Alzheimer prevention research, including the UCSF Brain Health Registry and the Banner Institute Alzheimer’s Prevention Initiative. We invite interested collaborators to reach out as we continue to build a network of health professionals who can provide the latest, safest, evidence-based preventative interventions for at-risk individuals, families and communities. We host a weekly consensus conference to discuss Alzheimer prevention cases, which is available for viewing and participation online every Wednesday morning (EST). Collaborators interested in attending the conference either in person or via web-based video conferencing are encouraged to contact us.
Conclusion
The first goal of the U.S. National Plan to Address Alzheimer’s Disease is to prevent and effectively treat AD by 2025. Until that time, a variety of evidence-based, low-risk clinical interventions may be instituted by primary care physicians, cardiologists, nutritionists and other healthcare professionals, in an effort to (in the least) lower validated risk scores. Besides clinical interventions, scores of interventional research trials are already available for individuals with prodromal Alzheimer disease or mild cognitive impairment (121). With continued engagement of healthy, community-dwelling adults at various levels of risk for Alzheimer’s or dementia, and with earlier interventions specifically targeting the biological mechanisms of disease at key stages in the life course, prior to neuronal loss, a major breakthrough in preventing AD may be finally within our reach. The APC will continue to work with collaborators worldwide to establish well-characterized populations of individuals who, either through clinical trial participation or evidence-based clinical care, can benefit from the disease-modifying interventions expected to arise in the coming decade.
Acknowledgments: The Authors would like to thank Dr. Matthew Fink, Dr. Laurie Glimcher, Dr. Robert Krikorian, Dr. Arthur Agatston, Dr. Islon Woolf, Dr. Randy Cohen, Yaa Obeng-Aduasare, Ciara Gaglio, Max Pensack, Jaclyn Chen, Mark McInnis, Dr. Roberta Marongiu, Dr. Josefina Meléndez-Cabrero, Dana Ergas, Dr. Gloria Chiang, Dr. Milos Ivkovic and Max Lugavere.
Conflict of interests: Dr. Isaacson has received research support from the American Academy of Neurology, student loan payments from the National Institutes of Health Clinical Research LRP, and an unrestricted educational research grant support from Accera, Inc. He has served as a scientific advisor/consultant to Novartis and Accera. Dr. Seifan has no financial interests, relationships or affiliations relevant to the subject of this manuscript.
Funding: Provided via philanthropic support (proceeds from the Annual Memories for Mary fundraiser, organized by Mr. David Twardock, and contributions from grateful patients of the Alzheimer’s Prevention Clinic, Weill Cornell Memory Disorders Program), and grant support by the Leon Levy Foundation and the Weill Cornell Medical College Clinical and Translational Science Center. The sponsors had no role in the preparation of the manuscript; or in the review or approval of the manuscript.
Ethical standard: The clinical and education research trials described have been reviewed and approved by the Weill Cornell Medical College IRB.
References
1. Sperling R, Mormino E, Johnson K. The evolution of preclinical Alzheimer’s disease: implications for prevention trials. Neuron 2014;84:608-622.
2. Smith AD, Yaffe K. Dementia (including Alzheimer’s disease) can be prevented: statement supported by international experts. J Alzheimers Dis 2014;38:699-703.
3. Tan CC, Yu JT, Tan L. Biomarkers for preclinical Alzheimer’s disease. J Alzheimers Dis 2014;42:1051-1069.
4. Anstey KJ, Cherbuin N, Herath PM, et al. A self-report risk index to predict occurrence of dementia in three independent cohorts of older adults: the ANU-ADRI. PLoS One 2014;9:e86141.
5. Sperling RA, Aisen PS, Beckett LA, et al. Toward defining the preclinical stages of Alzheimer’s disease: recommendations from the National Institute on Aging-Alzheimer’s Association workgroups on diagnostic guidelines for Alzheimer’s disease. Alzheimers Dement 2011;7:280-292.
6. Daviglus ML, Plassman BL, Pirzada A, et al. Risk factors and preventive interventions for Alzheimer disease: state of the science. Arch Neurol 2011;68:1185-1190.
7. International AsD. World Alzheimer Report 2011 The benefits of early diagnosis and intervention. 2011.
8. Christensen KD, Roberts JS, Royal CD, et al. Incorporating ethnicity into genetic risk assessment for Alzheimer disease: the REVEAL study experience. Genetics in medicine : official journal of the American College of Medical Genetics 2008;10:207-214.
9. Sano M, Egelko S, Donohue M, et al. Developing Dementia Prevention Trials: Baseline Report of the Home-Based Assessment Study. Alzheimer Dis Assoc Disord 2012.
10. Wagner SL, Tanzi RE, Mobley WC, Galasko D. Potential Use of gamma-Secretase Modulators in the Treatment of Alzheimer Disease. Arch Neurol 2012:1-4.
11. Godin G, Kok G. The theory of planned behavior: a review of its applications to health-related behaviors. American journal of health promotion : AJHP 1996;11:87-98.
12. Janz NK, Becker MH. The Health Belief Model: a decade later. Health Educ Q 1984;11:1-47.
13. Isaacson RS GJ, Seifan A. Validation of the Alzheimer’s Prevention and Risk Knowledge Score. Neurology 2015;77 (suppl.).
14. Isaacson RS KR, Ochner CN. Alzheimer’s Diet Modification: A Web-based Nutrition Tracking System For Patient Management and Outcomes Research. J Nutrition Health & Aging 2012;16.
15. Isaacson RS HN, Seifan A, Sherry Christiansen S, Berger JC, Larsen D, Safdieh J, Lunde AM, Luo A, Kramps M, McInnis M, Ochner CN. Alzheimer’s Prevention Education: If We Build It, Will They Come? . Journal of Prevention of Alzheimer’s disease September 2014.;1.
16. Trustram Eve C, de Jager CA. Piloting and validation of a novel self-administered online cognitive screening tool in normal older persons: the Cognitive Function Test. Int J Geriatr Psychiatry 2014;29:198-206.
17. Zola SM, Manzanares CM, Clopton P, Lah JJ, Levey AI. A behavioral task predicts conversion to mild cognitive impairment and Alzheimer’s disease. American journal of Alzheimer’s disease and other dementias 2013;28:179-184.
18. Dalton P, Doty RL, Murphy C, et al. Olfactory assessment using the NIH Toolbox. Neurology 2013;80:S32-36.
19. Di Noia J, Prochaska JO. Dietary stages of change and decisional balance: a meta-analytic review. American journal of health behavior 2010;34:618-632.
20. Nguyen TM, La Caze A, Cottrell N. What are validated self-report adherence scales really measuring?: a systematic review. British journal of clinical pharmacology 2014;77:427-445.
21. Carpenter BD, Balsis S, Otilingam PG, Hanson PK, Gatz M. The Alzheimer’s Disease Knowledge Scale: development and psychometric properties. Gerontologist 2009;49:236-247.
22. French SL, Floyd M, Wilkins S, Osato S. The Fear of Alzheimer’s Disease Scale: a new measure designed to assess anticipatory dementia in older adults. Int J Geriatr Psychiatry 2012;27:521-528.
23. Kim S, Sargent-Cox K, Cherbuin N, Anstey KJ. Development of the motivation to change lifestyle and health behaviours for dementia risk reduction scale. Dement Geriatr Cogn Dis Extra 2014;4:172-183.
24. Topolski TD, LoGerfo J, Patrick DL, Williams B, Walwick J, Patrick MB. The Rapid Assessment of Physical Activity (RAPA) among older adults. Preventing chronic disease 2006;3:A118.
25. Gonzalez-Escamilla G, Atienza M, Garcia-Solis D, Cantero JL. Cerebral and blood correlates of reduced functional connectivity in mild cognitive impairment. Brain structure & function 2014.
26. Cagnin A, Zambon A, Zarantonello G, et al. Serum lipoprotein profile and APOE genotype in Alzheimer’s disease. Journal of neural transmission Supplementum 2007:175-179.
27. Hottman DA, Chernick D, Cheng S, Wang Z, Li L. HDL and cognition in neurodegenerative disorders. Neurobiology of disease 2014;72 Pt A:22-36.
28. Sato Y, Suzuki I, Nakamura T, Bernier F, Aoshima K, Oda Y. Identification of a new plasma biomarker of Alzheimer’s disease using metabolomics technology. Journal of lipid research 2012;53:567-576.
29. Hostage CA, Choudhury KR, Murali Doraiswamy P, Petrella JR. Mapping the effect of the apolipoprotein E genotype on 4-year atrophy rates in an Alzheimer disease-related brain network. Radiology 2014;271:211-219.
30. Quinn TJ, Gallacher J, Deary IJ, Lowe GD, Fenton C, Stott DJ. Association between circulating hemostatic measures and dementia or cognitive impairment: systematic review and meta-analyzes. Journal of thrombosis and haemostasis : JTH 2011;9:1475-1482.
31. Tzikas S, Schlak D, Sopova K, et al. Increased myeloperoxidase plasma levels in patients with Alzheimer’s disease. J Alzheimers Dis 2014;39:557-564.
32. Fitzpatrick AL, Irizarry MC, Cushman M, Jenny NS, Chi GC, Koro C. Lipoprotein-associated phospholipase A2 and risk of dementia in the Cardiovascular Health Study. Atherosclerosis 2014;235:384-391.
33. Vidal JS, Sigurdsson S, Jonsdottir MK, et al. Coronary artery calcium, brain function and structure: the AGES-Reykjavik Study. Stroke 2010;41:891-897.
34. Zhong XM, Hou L, Luo XN, et al. Alterations of CSF cystatin C levels and their correlations with CSF Alphabeta40 and Alphabeta42 levels in patients with Alzheimer’s disease, dementia with lewy bodies and the atrophic form of general paresis. PLoS One 2013;8:e55328.
35. Tynkkynen J, Laatikainen T, Salomaa V, et al. NT-proBNP and the risk of dementia: a prospective cohort study with 14 years of follow-up. J Alzheimers Dis 2015;44:1007-1013.
36. Kiddle SJ, Thambisetty M, Simmons A, et al. Plasma based markers of [11C] PiB-PET brain amyloid burden. PLoS One 2012;7:e44260.
37. Ahn HJ, Glickman JF, Poon KL, et al. A novel Abeta-fibrinogen interaction inhibitor rescues altered thrombosis and cognitive decline in Alzheimer’s disease mice. The Journal of experimental medicine 2014;211:1049-1062.
38. Chen H, Liu S, Ji L, et al. Associations between Alzheimer’s disease and blood homocysteine, vitamin B12, and folate: a case-control study. Curr Alzheimer Res 2015;12:88-94.
39. Peng Q, Lao X, Huang X, Qin X, Li S, Zeng Z. The MTHFR C677T polymorphism contributes to increased risk of Alzheimer’s disease: evidence based on 40 case-control studies. Neurosci Lett 2015;586:36-42.
40. Yu Z, Li W, Hou D, et al. Relationship between Adiponectin Gene Polymorphisms and Late-Onset Alzheimer’s Disease. PLoS One 2015;10:e0125186.
41. Luciano R, Barraco GM, Muraca M, et al. Biomarkers of Alzheimer Disease, Insulin Resistance, and Obesity in Childhood. Pediatrics 2015.
42. Tan ZS, Harris WS, Beiser AS, et al. Red blood cell omega-3 fatty acid levels and markers of accelerated brain aging. Neurology 2012;78:658-664.
43. Berti V, Murray J, Davies M, et al. Nutrient patterns and brain biomarkers of Alzheimer’s disease in cognitively normal individuals. J Nutr Health Aging 2015;19:413-423.
44. Raj A, LoCastro E, Kuceyeski A, Tosun D, Relkin N, Weiner M. Network Diffusion Model of Progression Predicts Longitudinal Patterns of Atrophy and Metabolism in Alzheimer’s Disease. Cell reports 2015.
45. Kim HJ, Kim C, Jeon S, et al. Association of Body Fat Percentage and Waist-hip Ratio With Brain Cortical Thickness: A Study Among 1777 Cognitively Normal Subjects. Alzheimer Dis Assoc Disord 2015.
46. Debette S, Beiser A, Hoffmann U, et al. Visceral fat is associated with lower brain volume in healthy middle-aged adults. Ann Neurol 2010;68:136-144.
47. Kivipelto M, Ngandu T, Laatikainen T, Winblad B, Soininen H, Tuomilehto J. Risk score for the prediction of dementia risk in 20 years among middle aged people: a longitudinal, population-based study. Lancet neurology 2006;5:735-741.
48. Barnes DE, Covinsky KE, Whitmer RA, Kuller LH, Lopez OL, Yaffe K. Predicting risk of dementia in older adults: The late-life dementia risk index. Neurology 2009;73:173-179.
49. Nettiksimmons J, Ayonayon H, Harris T, et al. Development and validation of risk index for cognitive decline using blood-derived markers. Neurology 2015;84:696-702.
50. Hye A, Riddoch-Contreras J, Baird AL, et al. Plasma proteins predict conversion to dementia from prodromal disease. Alzheimers Dement 2014;10:799-807.e792.
51. Pankratz VS, Roberts RO, Mielke MM, et al. Predicting the risk of mild cognitive impairment in the Mayo Clinic Study of Aging. Neurology 2015;84:1433-1442.
52. Saffer BY, Lanting SC, Koehle MS, Klonsky ED, Iverson GL. Assessing cognitive impairment using PROMIS((R)) applied cognition-abilities scales in a medical outpatient sample. Psychiatry Res 2015;226:169-172.
53. Becker H, Stuifbergen A, Lee H, Kullberg V. Reliability and Validity of PROMIS Cognitive Abilities and Cognitive Concerns Scales Among People with Multiple Sclerosis. International journal of MS care 2014;16:1-8.
54. Lim YY, Pietrzak RH, Bourgeat P, et al. Relationships between performance on the Cogstate Brief Battery, neurodegeneration, and Abeta accumulation in cognitively normal older adults and adults with MCI. Archives of clinical neuropsychology : the official journal of the National Academy of Neuropsychologists 2015;30:49-58.
55. Jones S, Laukka EJ, Backman L. Differential verbal fluency deficits in the preclinical stages of Alzheimer’s disease and vascular dementia. Cortex 2006;42:347-355.
56. Mueller KD, Koscik RL, LaRue A, et al. Verbal Fluency and Early Memory Decline: Results from the Wisconsin Registry for Alzheimer’s Prevention. Archives of clinical neuropsychology : the official journal of the National Academy of Neuropsychologists 2015.
57. Pilkonis PA, Yu L, Dodds NE, Johnston KL, Maihoefer CC, Lawrence SM. Validation of the depression item bank from the Patient-Reported Outcomes Measurement Information System (PROMIS) in a three-month observational study. Journal of psychiatric research 2014;56:112-119.
58. Schalet BD, Cook KF, Choi SW, Cella D. Establishing a common metric for self-reported anxiety: linking the MASQ, PANAS, and GAD-7 to PROMIS Anxiety. Journal of anxiety disorders 2014;28:88-96.
59. Buysse DJ, Yu L, Moul DE, et al. Development and validation of patient-reported outcome measures for sleep disturbance and sleep-related impairments. Sleep 2010;33:781-792.
60. Cohen S, Kamarck T, Mermelstein R. A global measure of perceived stress. Journal of health and social behavior 1983;24:385-396.
61. Kaufer DI, Cummings JL, Ketchel P, et al. Validation of the NPI-Q, a brief clinical form of the Neuropsychiatric Inventory. The Journal of neuropsychiatry and clinical neurosciences 2000;12:233-239.
62. Castel LD, Williams KA, Bosworth HB, et al. Content validity in the PROMIS social-health domain: a qualitative analysis of focus-group data. Quality of life research : an international journal of quality of life aspects of treatment, care and rehabilitation 2008;17:737-749.
63. Lawton MP, Brody EM. Assessment of older people: self-maintaining and instrumental activities of daily living. Gerontologist 1969;9:179-186.
64. Patrick KE, McCurdy MD, Chute DL, Mahone EM, Zabel TA, Jacobson LA. Clinical utility of the Colorado Learning Difficulties Questionnaire. Pediatrics 2013;132:e1257-1264.
65. Tamboer P, Vorst HC, Oort FJ. Identifying dyslexia in adults: an iterative method using the predictive value of item scores and self-report questions. Annals of dyslexia 2014;64:34-56.
66. Nergard-Nilssen T, Hulme C. Developmental dyslexia in adults: behavioural manifestations and cognitive correlates. Dyslexia (Chichester, England) 2014;20:191-207.
67. Russ TC, Kivimaki M, Starr JM, Stamatakis E, Batty GD. Height in relation to dementia death: individual participant meta-analysis of 18 UK prospective cohort studies. Br J Psychiatry 2014;205:348-354.
68. Albert MS, DeKosky ST, Dickson D, et al. The diagnosis of mild cognitive impairment due to Alzheimer’s disease: recommendations from the National Institute on Aging-Alzheimer’s Association workgroups on diagnostic guidelines for Alzheimer’s disease. Alzheimers Dement 2011;7:270-279.
69. Jessen F, Amariglio RE, van Boxtel M, et al. A conceptual framework for research on subjective cognitive decline in preclinical Alzheimer’s disease. Alzheimers Dement 2014;10:844-852.
70. Ferini-Strambi L, Marelli S, Galbiati A, Rinaldi F, Giora E. REM Sleep Behavior Disorder (RBD) as a marker of neurodegenerative disorders. Archives italiennes de biologie 2014;152:129-146.
71. McKeith IG, Dickson DW, Lowe J, et al. Diagnosis and management of dementia with Lewy bodies: third report of the DLB Consortium. Neurology 2005;65:1863-1872.
72. Armstrong MJ, Litvan I, Lang AE, et al. Criteria for the diagnosis of corticobasal degeneration. Neurology 2013;80:496-503.
73. Rascovsky K, Hodges JR, Knopman D, et al. Sensitivity of revised diagnostic criteria for the behavioural variant of frontotemporal dementia. Brain 2011;134:2456-2477.
74. Rogalski E, Johnson N, Weintraub S, Mesulam M. Increased frequency of learning disability in patients with primary progressive aphasia and their first-degree relatives. Arch Neurol 2008;65:244-248.
75. Ju YE, McLeland JS, Toedebusch CD, et al. Sleep quality and preclinical Alzheimer disease. JAMA Neurol 2013;70:587-593.
76. Postuma RB, Iranzo A, Hogl B, et al. Risk factors for neurodegeneration in idiopathic rapid eye movement sleep behavior disorder: A multicenter study. Ann Neurol 2015;77:830-839.
77. Dodge HH, Mattek NC, Austin D, Hayes TL, Kaye JA. In-home walking speeds and variability trajectories associated with mild cognitive impairment. Neurology 2012;78:1946-1952.
78. Gourraud PA, Henry RG, Cree BA, et al. Precision medicine in chronic disease management: The multiple sclerosis BioScreen. Ann Neurol 2014;76:633-642.
79. Tamashiro-Duran JH, Squarzoni P, de Souza Duran FL, et al. Cardiovascular risk in cognitively preserved elderlies is associated with glucose hypometabolism in the posterior cingulate cortex and precuneus regardless of brain atrophy and apolipoprotein gene variations. Age (Dordrecht, Netherlands) 2013;35:777-792.
80. Cummings JL, Isaacson RS, Schmitt FA, Velting DM. A practical algorithm for managing Alzheimer’s disease: what, when, and why? Annals of clinical and translational neurology 2015;2:307-323.
81. Sarkamo T, Tervaniemi M, Laitinen S, et al. Cognitive, emotional, and social benefits of regular musical activities in early dementia: randomized controlled study. Gerontologist 2014;54:634-650.
82. Marquez-Contreras E, Martell-Claros N, Gil-Guillen V, et al. Efficacy of a home blood pressure monitoring programme on therapeutic compliance in hypertension: the EAPACUM-HTA study. J Hypertens 2006;24:169-175.
83. Paganini-Hill A, Kawas CH, Corrada MM. Lifestyle Factors and Dementia in the Oldest-old: The 90+ Study. Alzheimer Dis Assoc Disord 2015.
84. Wells RE, Yeh GY, Kerr CE, et al. Meditation’s impact on default mode network and hippocampus in mild cognitive impairment: a pilot study. Neurosci Lett 2013;556:15-19.
85. Olatunji BO, Kauffman BY, Meltzer S, Davis ML, Smits JA, Powers MB. Cognitive-behavioral therapy for hypochondriasis/health anxiety: a meta-analysis of treatment outcome and moderators. Behaviour research and therapy 2014;58:65-74.
86. Gliebus G, Lippa CF. The influence of beta-blockers on delayed memory function in people with cognitive impairment. American journal of Alzheimer’s disease and other dementias 2007;22:57-61.
87. Rouch L, Cestac P, Hanon O, et al. Antihypertensive drugs, prevention of cognitive decline and dementia: a systematic review of observational studies, randomized controlled trials and meta-analyses, with discussion of potential mechanisms. CNS drugs 2015;29:113-130.
88. Read S, Wu P, Biscow M. Sustained 4-year cognitive and functional response in early Alzheimer’s disease with pioglitazone. J Am Geriatr Soc 2014;62:584-586.
89. Unfer V, Carlomagno G, Dante G, Facchinetti F. Effects of myo-inositol in women with PCOS: a systematic review of randomized controlled trials. Gynecological endocrinology : the official journal of the International Society of Gynecological Endocrinology 2012;28:509-515.
90. Singh RB, Niaz MA, Rastogi SS, Shukla PK, Thakur AS. Effect of hydrosoluble coenzyme Q10 on blood pressures and insulin resistance in hypertensive patients with coronary artery disease. Journal of human hypertension 1999;13:203-208.
91. Mastroiacovo D, Kwik-Uribe C, Grassi D, et al. Cocoa flavanol consumption improves cognitive function, blood pressure control, and metabolic profile in elderly subjects: the Cocoa, Cognition, and Aging (CoCoA) Study–a randomized controlled trial. Am J Clin Nutr 2015;101:538-548.
92. Chapman MJ, Orsoni A, Robillard P, Hounslow N, Sponseller CA, Giral P. Effect of high-dose pitavastatin on glucose homeostasis in patients at elevated risk of new-onset diabetes: insights from the CAPITAIN and PREVAIL-US studies. Curr Med Res Opin 2014;30:775-784.
93. de Jager CA, Oulhaj A, Jacoby R, Refsum H, Smith AD. Cognitive and clinical outcomes of homocysteine-lowering B-vitamin treatment in mild cognitive impairment: a randomized controlled trial. Int J Geriatr Psychiatry 2012;27:592-600.
94. Smith AD, Smith SM, de Jager CA, et al. Homocysteine-lowering by B vitamins slows the rate of accelerated brain atrophy in mild cognitive impairment: a randomized controlled trial. PLoS One 2010;5:e12244.
95. Annweiler C, Llewellyn DJ, Beauchet O. Low serum vitamin D concentrations in Alzheimer’s disease: a systematic review and meta-analysis. J Alzheimers Dis 2013;33:659-674.
96. Annweiler C, Montero-Odasso M, Llewellyn DJ, Richard-Devantoy S, Duque G, Beauchet O. Meta-analysis of memory and executive dysfunctions in relation to vitamin D. J Alzheimers Dis 2013;37:147-171.
97. Jerneren F, Elshorbagy AK, Oulhaj A, Smith SM, Refsum H, Smith AD. Brain atrophy in cognitively impaired elderly: the importance of long-chain omega-3 fatty acids and B vitamin status in a randomized controlled trial. Am J Clin Nutr 2015.
98. Safouris A, Hambye AS, Sculier C, et al. Chronic brain hypoperfusion due to multi-vessel extracranial atherosclerotic disease: a potentially reversible cause of cognitive impairment. J Alzheimers Dis 2015;43:23-27.
99. Ishihara H, Oka F, Shirao S, et al. Cognitive outcome differences on the side of carotid artery stenting. Journal of vascular surgery 2013;57:125-130.
100. de la Serna G, Cadarso C. Fenofibrate decreases plasma fibrinogen, improves lipid profile, and reduces uricemia. Clinical pharmacology and therapeutics 1999;66:166-172.
101. Danesh J, Lewington S, Thompson SG, et al. Plasma fibrinogen level and the risk of major cardiovascular diseases and nonvascular mortality: an individual participant meta-analysis. Jama 2005;294:1799-1809.
102. Smith JC, Nielson KA, Woodard JL, et al. Physical activity reduces hippocampal atrophy in elders at genetic risk for Alzheimer’s disease. Front Aging Neurosci 2014;6:61.
103. Kivipelto M, Solomon A, Ahtiluoto S, et al. The Finnish Geriatric Intervention Study to Prevent Cognitive Impairment and Disability (FINGER): study design and progress. Alzheimers Dement 2013;9:657-665.
104. Smart NA, Titus TT. Outcomes of early versus late nephrology referral in chronic kidney disease: a systematic review. The American journal of medicine 2011;124:1073-1080.e1072.
105. Ihle A, Oris M, Fagot D, Baeriswyl M, Guichard E, Kliegel M. The Association of Leisure Activities in Middle Adulthood with Cognitive Performance in Old Age: The Moderating Role of Educational Level. Gerontology 2015.
106. Wegner M, Helmich I, Machado S, Nardi AE, Arias-Carrion O, Budde H. Effects of exercise on anxiety and depression disorders: review of meta- analyses and neurobiological mechanisms. CNS & neurological disorders drug targets 2014;13:1002-1014.
107. Rasgon NL, Geist CL, Kenna HA, Wroolie TE, Williams KE, Silverman DH. Prospective randomized trial to assess effects of continuing hormone therapy on cerebral function in postmenopausal women at risk for dementia. PLoS One 2014;9:e89095.
108. McCormack L, Sheridan S, Lewis M, et al. Communication and dissemination strategies to facilitate the use of health-related evidence. Evid Rep Technol Assess (Full Rep) 2013:1-520.
109. National Plan to Address Alzheimer’s Disease 2013 :PDATEU.S. Department of Health & Human Services, 2013.
110. Douglas JT. Adaptation to Early-Stage Nonfluent/Agrammatic Variant Primary Progressive Aphasia: A First-Person Account. American journal of Alzheimer’s disease and other dementias 2014;29:289-292.
111. Goldman JS, Hahn SE, Catania JW, et al. Genetic counseling and testing for Alzheimer disease: joint practice guidelines of the American College of Medical Genetics and the National Society of Genetic Counselors. Genetics in medicine : official journal of the American College of Medical Genetics 2011;13:597-605.
112. Kim SY, Karlawish J, Berkman BE. Ethics of genetic and biomarker test disclosures in neurodegenerative disease prevention trials. Neurology 2015;84:1488-1494.
113. Green RC, Roberts JS, Cupples LA, et al. Disclosure of APOE genotype for risk of Alzheimer’s disease. N Engl J Med 2009;361:245-254.
114. Klitzman R. Exclusion of genetic information from the medical record: ethical and medical dilemmas. Jama 2010;304:1120-1121.
115. Vernarelli JA. Impact of genetic risk assessment on nutrition-related lifestyle behaviours. The Proceedings of the Nutrition Society 2013;72:153-159.
116. Vernarelli JA, Roberts JS, Hiraki S, Chen CA, Cupples LA, Green RC. Effect of Alzheimer disease genetic risk disclosure on dietary supplement use. Am J Clin Nutr 2010;91:1402-1407.
117. Caselli RJ, Langbaum J, Marchant GE, et al. Public perceptions of presymptomatic testing for Alzheimer disease. Mayo Clin Proc 2014;89:1389-1396.
118. Padilla C, Isaacson RS. Genetics of dementia. Continuum (Minneapolis, Minn) 2011;17:326-342.
119. Taylor DH, Jr., Cook-Deegan RM, Hiraki S, Roberts JS, Blazer DG, Green RC. Genetic testing for Alzheimer’s and long-term care insurance. Health affairs (Project Hope) 2010;29:102-108.
120. Service CR. Genetics and Life, Disability and Long-Term Care Insurance2008.
121. Available at: https://clinicaltrials.gov/ct2/results?term=alzheimers& Search=Search.
122. Norton S, et al. Potential for primary prevention of Alzheimer’s disease: an analysis of population based data. Lancet Neurology 2014;13:788-794.