A.M. Fosnacht1, S. Patel1, C. Yucus1, A. Pham1, E. Rasmussen1, R. Frigerio1, S. Walters2, D. Maraganore1
1. NorthShore Neurological Institute, NorthShore University HealthSystem, Evanston, IL, USA; 2. Research Institute, NorthShore University HealthSystem, Evanston, IL, USA
Corresponding Author: Demetrius M. Maraganore, MD, Ruth Cain Ruggles Chairman, Department of Neurology, Medical Director, NorthShore Neurological Institute, NorthShore University Health System, 2650 Ridge Ave., Evanston, IL, 60201, USA, Tel: 1-847-570-1678, Fax: 1-847-733-5565, Email: dmaraganore@northshore.org
J Prev Alz Dis 2017;4(3):157-164
Published online January 31, 2017, http://dx.doi.org/10.14283/jpad.2017.3
Abstract
Background: Alzheimer’s disease and aging brain disorders are progressive, often fatal neurodegenerative diseases. Successful aging, modern lifestyles and behaviors have combined to result in an expected epidemic. Risks for these diseases include genetic, medical, and lifestyle factors; over 20 modifiable risks have been reported.
Objectives: We aim to primarily prevent Alzheimer’s disease and related disorders through electronic medical record (EMR)-based screening, risk assessments, interventions, and surveillance.
Design: We identified modifiable risks; developed human, systems and infrastructural resources; developed interventions; and targeted at-risk groups for the intervention.
Setting: A Community Based Health System.
Participants: In year one (June 2015 to May 2016), 133 at-risk patients received brain health services with the goal of delaying or preventing Alzheimer’s disease and related disorders.
Measurements: We created mechanisms to identify patients at high risk of neurodegenerative disease; EMR-based structured clinical documentation support tools to evaluate risk factors and history; evidence-based interventions to modify risk; and the capacity for annual surveillance, pragmatic trials, and practice-based and genomic research using the EMR.
Results: This paper describes our Center for Brain Health, our EMR tools, and our first year of healthy but at-risk patients.
Conclusion: We are translating research into primary prevention of Alzheimer’s disease and related disorders in our health system and aim to shift the paradigm in Neurology from brain disease to brain health.
Key words: Neurodegeneration, brain health, primary prevention, risk assessments, surveillance, electronic medical record.
Introduction
Alzheimer’s disease (AD) is an aging-related neurodegenerative disorder characterized by progressive accumulation of beta-amyloid protein plaques and tangles of the protein tau in and around neurons the brain. It is the most common type of dementia, accounting for well over half of cases (1). Early presentation includes lapses in memory regarding conversations, recent events and names, followed by psychological manifestations and then by impaired behavioral and motor functions. It is the 6th leading cause of death in the US (1).
Social phenomena such as the aging of Baby Boomers, “successful aging”, and growth of the oldest-old segment of the population have forecasted epidemics of aging-related disorders (1). Alzheimer’s disease is of particular concern due to high prevalence, limitedly efficacious pharmaceuticals, and disappointing clinical trials (2). Today, 5.4 million Americans live with AD and prevalence expectations for the year 2050 range from 13 to 16 million (1). Prevalence patterns are mirrored in developed nations and AD has been identified as a priority by the G8 nation’s recently formed World Dementia Council (3).
Alzheimer’s disease is expensive. The combined costs of AD total $236 billion per year (1), fueled by a number of factors including extraordinary cost of nursing homes, which can exceed $92,378 per year (4), and hospital stays, which are longer for patients with AD regardless of reason for hospitalization (1). By mid-century, costs are expected to exceed $1 trillion. Survey research reveals heavy burden to unpaid/family caregivers, who report serious work and career-related sacrifices, emotional stress, physical pain, and financial struggle (1).
The lifetime risk for AD is approximately 1 in 5 for women and 1 in 10 for men (1); however, knowledge of a variety of health and genetic factors allows personalization of risk estimates. In 1993, the Duke Alzheimer’s Disease Research Center Group published 3 papers describing their discovery that variations in the gene Apolipoprotein E (APOE) are associated with different risks of non-familial AD (5). Depending on one’s APOE genotype, risk may be 40% less than referent or fifteen times more. In 2010, the US National Institutes of Health issued an Independent State-Of-The-Science Report that named diabetes, smoking, and depression as having reliable evidence for increasing risk for AD. Evidence for other factors was lacking, and the authors called for more rigorous and higher quality research (6). Five years later, a meta-analysis by the Alzheimer’s Association reported a robustly stronger body of literature supporting modifiable risk factors for cognitive decline and dementia (3). Research also supports a variety of APOE gene-environment interactions and highlights the particularly beneficial implications of risk mitigation for APOE ε4 carriers (7-9).
In 2015 the results of the first randomized-controlled trial of a multi-domain intervention among at-risk community-dwelling elderly were published, which demonstrated that multi-modal lifestyle intervention could improve or maintain cognitive function even in elderly at-risk adults (10). Accordingly, calls for action concerning models and initiatives in primary prevention of AD have been published. Statistical estimates of what is possible for prevalence reduction in the US range from 30% to 50% (11, 12).
Based on the ability to risk-stratify, the weight of the evidence on modifiable risk factors, and available resources, the Neurology Department at NorthShore University HealthSystem aimed to build mechanisms to identify cognitively healthy but at-risk individuals years before a possible diagnosis of AD, Parkinson’s disease (PD) or chronic traumatic encephalopathy (CTE), to build practice-based interventions to manage risk factors, and surveillance mechanisms to monitor brain health with the goal of primary prevention of neurodegenerative disease. Uniquely, we have built in the capacity for quality improvement and practice-based research using the electronic medical record (EMR), including creation of a biobank (“clinomics”), a Neurology Practice Based Research Network (NPBRN), and informatics tools to conduct pragmatic trials using subgroup based adaptive designs (13). This paper describes how we built the Center for Brain Health, describes our EMR tools and first year of patients, and characterizes our opportunity for risk mitigation and prevention.
Methods
Step 1: Identification of risk and protective factors
We identified as having strong evidence in the literature the following factors that increase risk for AD and related disorders: genetic (family history, APOE ε4, other susceptibility genes) (14-17), metabolic (cholesterol, diabetes, midlife obesity) (3, 5, 6, 11, 12, 17), vascular (cardiovascular disease, midlife hypertension, stroke) (3, 12), infectious and inflammatory (periodontitis, others) (18), head trauma (3), diet (homocysteine, standard American diet, nutrient deficiencies) (17, 19), habits (smoking, alcohol abuse) (3,6,11,12,17,20), sleep (poor quality, disorders) (3, 7, 8), depression (3, 6, 10, 16), early menopause (natural or surgical) (21), sedentary lifestyle (11, 12) and certain medications (22, 23) (Table 1). We identified as having strong evidence in the literature as decreasing risk for AD and related disorders the following factors: genetic (APOE ε2) (5, 15), social (education, income, engagement) (3, 24), lifestyle (physical, mental exercise) (3, 6, 17, 25), diet (Mediterranean) (3, 17), vitamins (B6/B12/Folate, A, C, D, E) (17, 26), medications (NSAIDS, statins, early hormone replacement therapy, antihypertensives, antidiabetics) (17, 27, 28). (Table 1)
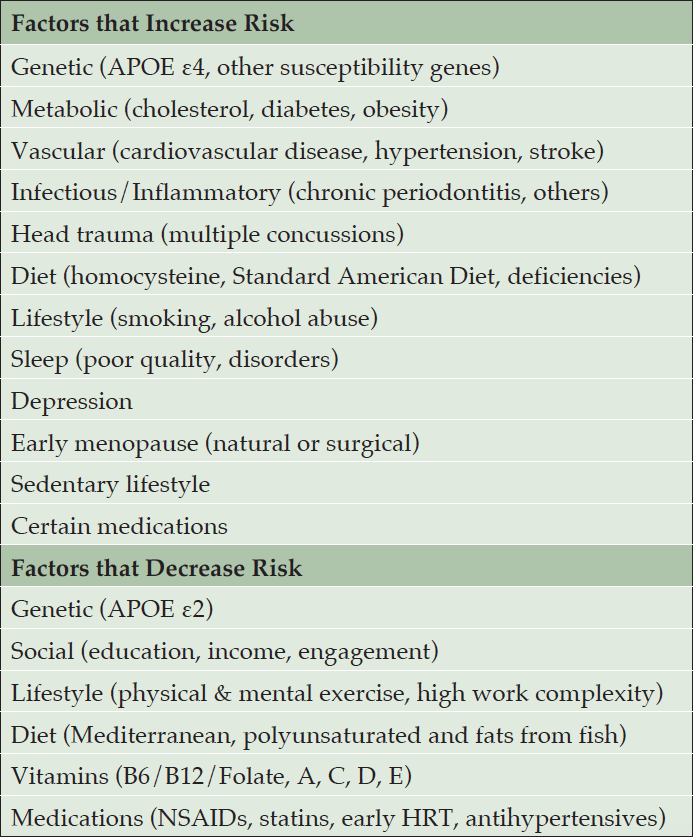
Table 1. Survey of the literature reveals at least 20 modifiable risk factors for Alzheimer’s disease and related disorders
Step 2: Resource mobilization
The Center for Brain Health exists within the NorthShore Neurological Institute of NorthShore University HealthSystem and utilizes its staff, offices, equipment and infrastructure. NorthShore University HealthSystem is a comprehensive, fully integrated healthcare delivery system serving the North Chicago region and includes 4 hospitals and 2,100 affiliated/employed physicians (29). It was amongst the first health systems to adopt the EMR in the US and was amongst the first to demonstrate “meaningful use” of EMR technology in ways that translate to improved quality, safety and efficiency for patients. Downstream from its EMR, NorthShore maintains an enterprise data warehouse (EDW) that fosters health-related data analytics and enables broad capacity for increasingly longitudinal health-related research and informatics.
Concept development and planning began via the invitation of physicians and internal professionals to join one of five working groups that met monthly for up to 24 months to develop the concept vis-à-vis their areas of expertise. All groups were lead by the Chairman of Neurology (Director, Center for Brain Health).
The Research and Development Working Group consisted of experts in biomedical research informatics, epidemiology, genomics, molecular medicine, neurology, neuropathology, neuroradiology, nutrition, primary care, and animal models of neurodegeneration. They were tasked with deliberating over gaps in knowledge regarding at-risk populations, risk assessments, interventions, and addressing these gaps through point-of-care research utilizing the EMR.
The Community Engagement Working Group consisted of experts in community relations, marketing, patient engagement, philanthropy, and public health. They were tasked with building strategic community partnerships, community resource leveraging, and identifying events for participation to expand the reach of screening.
The Targeted Populations Working Group consisted of experts in genomics, healthcare administration, health information technology (HIT), marketing, neurology (memory disorders and movement disorders), public health, primary care, reproductive endocrinology, and sports concussion. They were charged with describing high-risk populations and recruitment mechanisms.
The Risk Assessments and Surveillance Working Group consisted of experts in healthcare administration, neurology (memory disorders and movement disorders), neuropsychology, neuroradiology, nuclear medicine, primary care, and public health. Their goal was to identify reliable, valid and pragmatic measures of brain health for patient assessments, and mechanisms for surveillance and capturing outcomes.
The Interventions Working Group consisted of experts in cognitive and physical therapy, healthcare administration, HIT, integrative medicine, medical social work, neurology (memory disorders and movement disorders), nutrition, and public health. Their goal was to develop a suite of evidence-based interventions for modifiable risk factors for AD, PD and CTE and strategize how to operationalize these within the framework of the health system.
Key practitioners recruited for Center for Brain Health include three neurologists (PD, memory, sleep and integrative), a registered and research dietitian, a medical social worker, a physician assistant, and therapists from cognitive and physical therapy. Non-clinical staff includes a practice manager, a senior clinical research associate, research assistants, a statistician, and HIT programmer analysts.
Step 3: Build Structured Clinical Documentation Support (SCDS) Toolkits into the Electronic Medical Record
As part of an initiative funded by the Agency for Healthcare Research and Quality to improve the quality of neurology clinical practice and facilitate point-of-care practice-based research using the EMR, the Center for Brain Health utilizes a SCDS toolkit in the EMR for all patient encounters (13). Center for Brain Health neurologists met biweekly for three months to standardize Brain Health office visit types according to evidence-based medicine (toward Best Practices). We developed consensus on: definitions of AD, PD and CTE; outcomes of interest to clinicians and patients; valid and feasible outcome measures for point-of-care assessments; and factors known to influence the outcomes and measures. The neurologists met with members of NorthShore’s EMR optimization team biweekly over three months to develop and test the SCDS toolkit which navigates care, writes progress notes, provides clinical decision support, and electronically captures structured data.
The brain health SCDS toolkit is utilized by the care team (medical assistant, nurse, neurologist, research assistant when appropriate). The tools include a custom navigator (index of electronic forms), electronic forms (documentation flow sheets, including cascading data elements, auto-scoring and interpreting and other “smart form” features), Best Practice Advisories (pop-up alerts), and order sets. The content of the electronic forms includes several score test measures (Appendix A) and customized fields that discretely document: chief complaints, patient information (ancestry, special diets, caffeine use, exercise habits, health maintenance, toxin and medication exposures), past medical history (specific to aging brain disorders), family history (specific to aging brain disorders), traumatic brain injury, prior treatments (nootropic, dopaminergic, nutraceutical), prior diagnostics (brain imaging modalities, electroencephalography, polysomnography, neuropsychological, genetic, and cerebrospinal fluid testing), and blood tests. These tools electronically capture 400+ fields of data. Some examples of screenshots of the toolkit are shown in Figure 1, with additional screenshots shown in Appendix B.
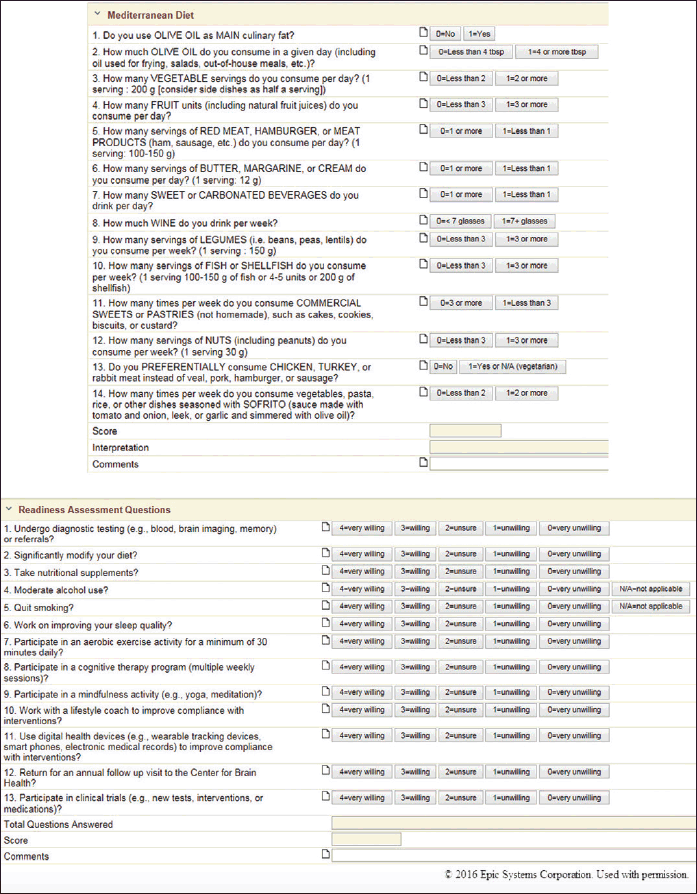
Figure 1. Screenshots of structured clinical documentation support tools that we have built into the electronic medical record (EPIC) that assess known risks to Alzheimer’s and related disorders. These tools electronically capture large amounts of clinical and diagnostic data. Data capture includes: Mediterranean diet and Readiness Assessment (both shown below). Additional screenshots are shown in Appendix A. © 2015 EPIC Systems, used with permission
Step 4: Identification of at-risk patients
To identify at-risk patients currently engaged with our health system, we sent mailings to Primary Care Providers (PCPs), posted articles to internal websites and newsletters, and gave presentations to NorthShore committees (Council of Chairmen, Medical Group Primary Care Committee, and Medical Group Specialty Care Committee) and departments (Internal Medicine, Neurology, Obstetrics and Gynecology).
To identify at-risk individuals in the community, we sponsored activities and gave presentations at community events. We authored blogs and webinars for local chapters of aging and dementia-related national organizations. The Center for Brain Health initiative has been represented at national scientific conferences and featured in media interviews. We advertised on television, radio, the internet, billboards, newspapers, and magazines.
We developed informatics tools to identify targeted populations: a web- and paper-based Brain Health Quiz (Appendix C), an EMR-based Alzheimer’s Risk Score Algorithm, and EMR-based flags. The Brain Health Quiz is an un-scored self-screening tool of evidence-based risk factors for AD, PD, and CTE by which individuals can learn about their risk factors and self-refer. We distribute the paper-based quiz at events and post it electronically on NorthShore intranet and internet sites.
The Alzheimer’s Risk Score is an algorithm-based clinical decision support tool that predicts mild cognitive impairment (MCI), dementia, or AD in the next five years for patients aged 60+. It will be built into the EMR as a widget (on-demand score) and as a Best Practice Advisory (pop-up notification for high-risk patients). To construct the algorithm: we utilized data stored in the EDW for patients ages 60+ who had visited their primary care physician in 2009 and again in 2014. We excluded patients who in 2009 had a diagnosis of MCI, dementia, or AD. We included as independent variables any of the 23 factors listed in the Brain Health Quiz that were captured by the EMR by 2009. We included as dependent variables diagnosis of MCI, dementia, or AD by 2014. Using stepwise statistical model building, we identified variables in the patients’electronic records that contributed to higher risk. Details regarding the model will be published separately. Additionally, we will flag patients in the EMR with a documented family history of AD or PD, multiple concussions or more-severe brain injuries, rapid eye movement sleep behavior disorder, or early-unopposed menopause, for primary care physicians to consider referral to the Center for Brain Health.
Step 5: Develop personalized medicine and interventions
We implement personalized, evidence-based interventions to mitigate risk factors and maximize protective factors, and evidence-based interventions with the potential to benefit all patients. These interventions included lifestyle and behavioral changes, medications, and management of diseases associated with increased risk of AD. We maintain compliance-focused follow-up via interval visits with a physician assistant. Annual follow-up visits with the neurologist focus on updating risk profiles and modifying interventions according to proximal outcomes and the latest evidence. In the event that patients develop aging brain disorders despite our best efforts, they are transitioned at the earliest point to relevant neurology subspecialty practices.
The diagnostic testing and interventions are defined by an order set built into the EMR. The “smart set” includes frequently ordered labs tests (e.g., metabolic panel, complete blood count with differential, cardiac risk, and vitamin D-25, Vitamin B12, Folic acid blood levels), imaging tests (MRI, CT), additional lab tests (e.g., glucose test, homocysteine level), genetic tests (e.g., APOE, early onset Alzheimer’s evaluation), additional procedures (e.g., cerebrospinal fluid examination), frequently ordered medications (e.g., Folic Acid-Pyridoxine-Cyancobalamin), consults (e.g. dietitian, physical therapy), diagnoses, and billing codes.
We built into the EMR Best Practice Advisories that prompt neurologists to enroll patients into a DNA biobank (each is genotyped for one million single nucleotide polymorphism markers), or to complete a mental health order set when patients were severely anxious or depressed and not taking an anxiolytic or an antidepressant and without documentation of a visit with a mental health practitioner in the prior year.
Step 6: Community Engagement
To include our community in defining our clinical services, we created a Community Advisory Council whose membership consisted of patients with aging brain disorders, caregivers, municipal leaders, public health experts, and professionals representing our partnering organizations. We met bimonthly to provide progress updates and receive feedback.
Statistical Methods
We generated from the Center-for-Brain-Health-specific data mart in the enterprise data warehouse a descriptive cohort report (medians and ranges, means and standard deviations, frequencies, overall and in men and women separately), visualized the data using box plots, bar graphs, and normal Q-Q plots, and performed pairwise correlations (without and with adjustments) and principal component (PC) analysis of the scored tests (without and with Varimax rotations). A statistician (SW) performed the analyses using SAS 9.3 (Cary, NC) and R software.
Results
We created mechanisms to identify patients at high risk of AD, PD, and CTE; the SCDS tools to evaluate risk factors and history; the evidence-based interventions to modify risk; and the capacity for annual surveillance, pragmatic trials, and practice-based and genomic research using the EMR.
In our first year (June 2015 to May 2016), we saw 133 patients. Fifteen were found to have MCI and were referred to the Memory Disorders Clinic for care. The remaining 118 patients were cognitively normal; median age was 59.5 (range 31-81); 81 were women. The median number of risk factors reported via the Brain Health Quiz was 5 (range 1-10). The median body mass index (BMI) for women was 26 and for men 27. The median PREDIMED score (a validated 14-item Mediterranean diet questionnaire) was 7 for both genders (range, 1-12); only 3.4% of patients had “strongly adherent to the Mediterranean diet” scores. Our patients were highly educated with 100% having finished high school, 83% completing 4 years college, 51% completing 2 years graduate school and 21% receiving a post-graduate education. A complete descriptive cohort report is provided in Appendix D.
Women reported with higher frequencies family histories of dementia, PD, depression, sleep apnea, prior head injuries, prior use of NSAIDs, exposure to pesticides, and alcohol use. Women scored more often in the clinical insomnia and depression ranges. Men were more likely to report caffeine use and sedentary activity. Men scored more often in the moderate to severe anxiety range. None of these gender differences reached statistical significance. Men were more likely to report statin use, the only gender difference reaching statistical significance (p <0.05).
Table 2 provides results of pairwise correlations; Figure 2 illustrates results of the PC analysis. The analyses included 118 patients with complete data for a Mediterranean diet questionnaire (PREDIMED); Center for Epidemiological Studies-Depression scale (CED-D); Generalized Anxiety Disorder 7-item scale (GAD-7); Insomnia Severity Index (ISI); 9-hole peg test, dominant hand (9-hole dom); 9-hole peg test, non-dominant hand (9-hole non-dom); 25-foot walking test (25ft walk); Short Test of Mental Status (STMS); Unified Parkinson’s Disease Rating Scale-Motor scale (UPDRS); The Eight-Item Informant Interview to Differentiate Aging and Dementia (AD8); a Parkinsonism screening questionnaire (PARK); Body-mass index (BMI); and a Brain Health Readiness Assessment (Readiness) which measures patients’ willingness to engage in behavior change specific to risk and protective factors. Several pairwise correlations were statistically significant even accounting for multiple comparisons. None of the measures were over-correlated (rho < 0.8 or > -0.8). We performed PC analyses restricting to validated score test measures (9-hole peg tests dominant and non-dominant, 25-ft walk, AD-8, CES-D, GAD-7, ISI, PARK, PREDIMED, STMS, UPDRS), and also including additional continuous trait measures (age at study, BMI, Readiness). For the PC analyses that included all measures, the 9-hole peg test (dominant hand) loaded to the first PC most heavily. Two-factor maps (PCs 1 and 2), without and with Varimax rotation (Figure 2), revealed clustering of ISI, BMI, AD-8, PREDIMED, and Readiness. Another cluster included STMS, 9-hole peg test (dominant, non-dominant), 25-ft walk, age at study, PARK, and UPDRS. GAD-7 and CES-D formed a 3rd distal cluster. Inspection of the PCs after Varimax rotation and restricting to factors with eigen values >1 revealed a three factor solution, where the score tests with component loadings >0.40 on PC1 included the 9-hole peg test non-dominant and the 9-hole peg test dominant and age at study, and the score tests with component loadings >0.40 on PC2 included the GAD-7 and CES-D, and the score tests with component loadings >0.40 on PC3 included AD-8 and Readiness. See Appendix E for a complete score test analytic report.
56 patients were referred to the dietitian. 28 patients opted for APOE genotype analysis. Of the 118 in our cohort, 101 were eligible for enrollment in our blood DNA and plasma biobank, and 88 (87.1%) of the eligible subjects participated.
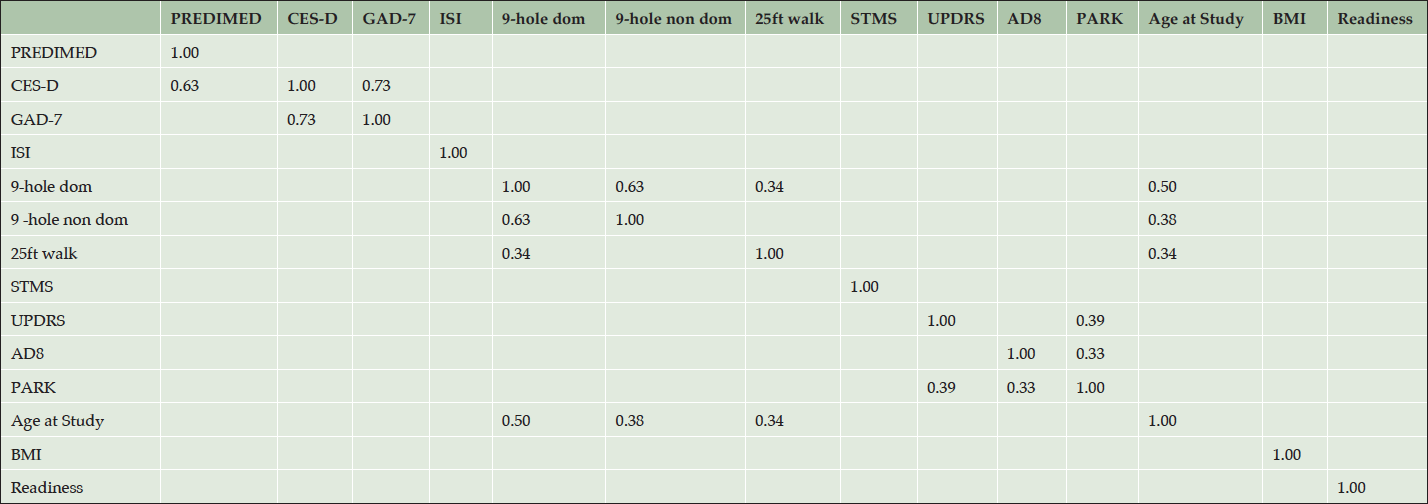
Table 2. Pairwise correlations of score test measures at initial visits for 118 patients in the cohort. Table shows pairwise correlations adjusted for age and gender. Correlation coefficients (rho) are shown in the cells only for significant correlations, p-value < .05/n where n = 91, the number of tests. Thus significance is established for p-value < 5.5 x 10-4 (after Bonferroni correction for multiple testing). Note that score tests compared to themselves (x and y axis of the table) are perfectly correlated (rho = 1.0)
PREDIMED (Mediterranean diet questionnaire); CES-D (Center for Epidemiological Studies-Depression); GAD-7 (Generalized Anxiety Disorder 7-item scale); ISI (Insomnia Severity Index); 9-hole dom (9-hole peg test, dominant hand); 9-hole non-dom (9-hole peg test, non-dominant hand); 25ft walk (25-foot walking test); STMS (Short Test of Mental Status); UPDRS (Unified Parkinson’s Disease Rating Scale-Motor); AD8 (The Eight-Item Informant Interview to Differentiate Aging and Dementia); PARK (Parkinsonism screening questionnaire); BMI (Body-mass index); Readiness (Brain Health Readiness Assessment).
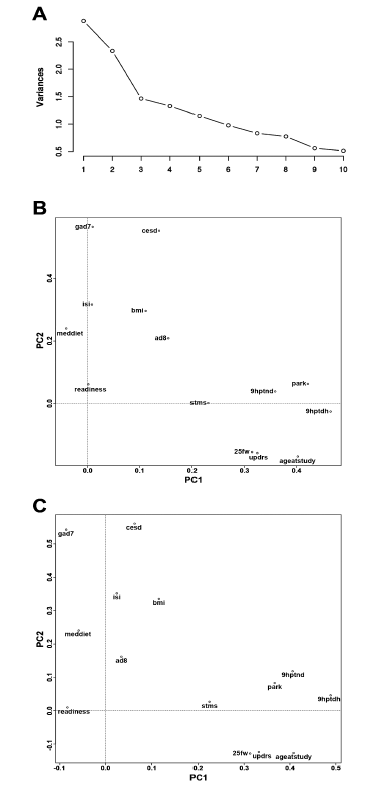
Figure 2. Principal component analyses for score test measures at initial visits for 118 patients in the cohort
A) Cascade figure, demonstrating the proportion of the variance between the measures explained stepwise by each of the principal components. B) Illustrates the principal component mappings of first principal component (PC1) versus second principle component (PC2) loadings for each of the score tests and their spatial relationships. C) Illustrates the principal component mappings of PC1 and PC2 loadings for each of the score tests, after Varimax rotation (to maximize the distance between measures). PREDIMED (Mediterranean diet questionnaire); CES-D (Center for Epidemiological Studies-Depression); GAD-7 (Generalized Anxiety Disorder 7-item scale); ISI (Insomnia Severity Index); 9-hole dom (9-hole peg test, dominant hand); 9-hole non-dom (9-hole peg test, non-dominant hand); 25ft walk (25-foot walking test); STMS (Short Test of Mental Status); UPDRS (Unified Parkinson’s Disease Rating Scale-Motor); AD8 (The Eight-Item Informant Interview to Differentiate Aging and Dementia); PARK (Parkinsonism screening questionnaire); BMI (Body-mass index); Readiness (Brain Health Readiness Assessment)
Discussion
In its first year, the Center for Brain Health initiative identified and engaged individuals at increased risk for Alzheimer’s disease and related disorders, explored gender differences, and identified opportunities for mitigation of risk at the individual, system, and community levels. The majority of our patients are women; they scored higher on our Readiness Questionnaire indicating that we may need to work harder to identify and engage men. On the other hand, as the lifetime risk of Alzheimer’s disease is double in woman versus men (1), this gender bias in referrals may be appropriate.
Only four patients (3.4%) were strongly adherent to the Mediterranean diet, and the median BMI for both genders was above the threshold of normal weight-for-height. As midlife obesity increases risk for AD (3, 11) and strong adherence to the Mediterranean diet reduces risk (3), these data serve as examples that characterize and describe the opportunity we have for risk mitigation in our ever expanding cohort While long term outcomes are beyond the scope of this descriptive paper, we aim to publish data regarding patient compliance and outcomes as the cohort matures and when change can be measured.
Identification of high-risk individuals within a population, risk mitigation, and surveillance are building blocks of primary prevention initiatives. We demonstrate that this is possible in a large health system and that leveraging the EMR and analytics can automate efforts and create learning opportunities. Not long ago, the adage that Alzheimer’s disease cannot be prevented was ubiquitous. But literature disputing that adage is growing, as are calls for action on primary prevention and public health approaches to the AD epidemic. In Baumgart’s 2015 meta-analysis (3), the authors state that it’s no longer acceptable to linger in academic discussion; that the evidence is too strong to warrant inaction. Similarly, Norton and others urge the undertaking of a population health approach (12). And, we may have proof that risk factor management will lower dementia prevalence in the real-world. This year, Satizabal and others reported a decline of dementia incidence among Framingham Heart Study participants of 44% over 3 decades (30). While the factors responsible for this reduction remain unclear, it is noteworthy that during those 3 decades, the level of education rose and most vascular risk factors declined (30).
We are not alone in this venture; similar initiatives have been developed around the country (Weil Cornell Alzheimer’s Prevention Clinic by New York Presbyterian, the Alzheimer’s Prevention Program at Cedars-Sinai, the Alzheimer’s Risk Assessment and Intervention Program at the University of Alabama at Birmingham) but we are unique in many ways including 1) our scope: we aim to primarily prevent not only Alzheimer’s disease, but also Parkinson’s disease and chronic traumatic encephalopathy; 2) our use of informatics: we are utilizing SCDS tools built into the EMR, and are building into the EMR an Alzheimer’s Risk Score to assist patients and physicians to identify and define risk; 3) our commitment to genomics and clinomics: we are biobanking DNAs from consenting subjects, genotyping the samples for 1 million genomic markers, and associating the genotypes with electronically captured clinical data. We anticipate referrals from NorthShore’s system-wide Genomic Health Initiative that will identify thousands of APOE E4 carriers; 4) our community engagement: through the Community Advisory Board, we partner to expand the scope of our services; 5) our collaborations: through the Neurology Practice Based Research Network (13) we are sharing our EMR tools and data, which will vastly increase our ability to improve the quality of the care we provide, to make new discoveries relating to brain health, to achieve better outcomes, and ultimately to reduce the burden of brain disorders in the communities we serve.
Acknowledgments: The authors acknowledge the generous support of the Auxiliary of NorthShore University HealthSystem with respect to the initial building of electronic medical record (EMR) toolkits, and thank the medical assistants, nurses, neurologists, EMR Optimization and Enterprise Data Warehouse programmers, administrators, and research personnel at NorthShore University HealthSystem who contributed to the quality improvement and practice-based research initiative using the EMR. The authors thank Vimal Patel, PhD for his assistance with editing, formatting, and submitting the manuscript for publication. Finally, the authors thank the neurology patients who inspire us to improve quality and to innovate our clinical practice every day.
Funding: The authors also acknowledge funding support from the Agency for Healthcare Research and Quality (R01HS024057).
Ethical standards: This is a cross-sectional description of historical patients seen in a clinical practice. There is no enrollment.
References
1. Alzheimer’s Association. 2016 Alzheimer’s Disease Facts and Figures. Alzheimer’s & Dementia 2016;12(4). https://www.alz.org/documents_custom/2016-facts-and-figures.pdf. [Accessed September 1, 2016]
2. Schneider LS, Mangialasche F, Andreasen N, et al. Clinical trials and late-stage drug development for Alzheimer’s disease: an appraisal from 1984 to 2014. J Intern Med. 2014;275(3):251-83.
3. Baumgart M, Snyder HM, Carrillo MC, Fazio S, Kim H, Johns H. Summary of the evidence on modifiable risk factors for cognitive decline and dementia: A population-based perspective. Alzheimers Dement. 2015;11(6):718-26.
4. Genworth Financial, Inc. Genworth 2016 Cost of Care Survey. Home Care Providers, Adult Day Health Care Facilities, Assisted Living Facilities and Nursing Homes. https://www.genworth.com/about-us/industry-expertise/cost-of-care.html. [Accessed September 1, 2016]
5. Roses AD. On the discovery of the genetic association of Apolipoprotein E genotypes and common late-onset Alzheimer disease. J Alzheimers Dis. 2006;9(3 Suppl):361-6.
6. Daviglus ML, Bell CC, Berrettini W, et al. National Institutes of Health State-of-the-Science Conference statement: preventing alzheimer disease and cognitive decline. Ann Intern Med. 2010;153(3):176-81.
7. Burke SL, Maramaldi P, Cadet T, Kukull W. Associations between depression, sleep disturbance, and apolipoprotein E in the development of Alzheimer’s disease: dementia. Int Psychogeriatr. 2016;28(9):1409-24.
8. Lim AS, Yu L, Kowgier M, Schneider JA, Buchman AS, Bennett DA. Modification of the relationship of the apolipoprotein E ε4 allele to the risk of Alzheimer disease and neurofibrillary tangle density by sleep. JAMA Neurol. 2013;70(12):1544-51.
9. Morris MC, Brockman J, Schneider JA, et al. Association of Seafood Consumption, Brain Mercury Level, and APOE ε4 Status With Brain Neuropathology in Older Adults. JAMA. 2016;315(5):489-97.
10. Ngandu T, Lehtisalo J, Solomon A, et al. A 2 year multidomain intervention of diet, exercise, cognitive training, and vascular risk monitoring versus control to prevent cognitive decline in at-risk elderly people (FINGER): a randomized controlled trial. Lancet. 2015;385(9984):2255-63.
11. Norton S, Matthews FE, Barnes DE, Yaffe K, Brayne C. Potential for primary prevention of Alzheimer’s disease: an analysis of population-based data. Lancet Neurol. 2014;13(8):788-94.
12. Barnes DE, Yaffe K. The projected effect of risk factor reduction on Alzheimer’s disease prevalence. Lancet Neurol. 2011;10(9):819-28.
13. Maraganore DM, Frigerio R, Kazmi N, et al. Quality improvement and practice-based research in neurology using the electronic medical record. Neurol Clin Pract. 2015;5(5):419-429.
14. Foley SF, Tansey KE, Caseras X, et al. Multimodal Brain Imaging Reveals Structural Differences in Alzheimer’s Disease Polygenic Risk Carriers: A Study in Healthy Young Adults. Biol Psychiatry. 2016;pii: S0006-3223(16)31114-3.
15. Jansen WJ, Ossenkoppele R, Knol DL, et al. Prevalence of cerebral amyloid pathology in persons without dementia: a meta-analysis. JAMA. 2015;313(19):1924-38.
16. Scarabino D, Gambina G, Broggio E, Pelliccia F, Corbo RM. Influence of family history of dementia in the development and progression of late-onset Alzheimer’s disease. Am J Med Genet B Neuropsychiatr Genet. 2016;171B(2):250-6.
17. Xu W, Tan L, Wang HF, Jiang T, et al. Meta-analysis of modifiable risk factors for Alzheimer’s disease. J Neurol Neurosurg Psychiatry. 2015;86(12):1299-306.
18. Ide M, Harris M, Stevens A, et al. Periodontitis and Cognitive Decline in Alzheimer’s Disease. PLoS One. 2016;11(3):e0151081.
19. Hu Q, Teng W, Li J, Hao F, Wang N. Homocysteine and Alzheimer’s Disease: Evidence for a Causal Link from Mendelian Randomization. J Alzheimers Dis. 2016;52(2):747-56.
20. Mukamal KJ, Kuller LH, Fitzpatrick AL, Longstreth WT Jr, Mittleman MA, Siscovick DS. Prospective study of alcohol consumption and risk of dementia in older adults. JAMA. 2003;289(11):1405-13.
21. Rocca WA, Shuster LT, Grossardt BR, et al. Long-term effects of bilateral oophorectomy on brain aging: unanswered questions from the Mayo Clinic Cohort Study of Oophorectomy and Aging. Womens Health (Lond). 2009;5(1):39-48.
22. Badiola N, Alcalde V, Pujol A, et al. The proton-pump inhibitor lansoprazole enhances amyloid beta production. PLoS One. 2013;8(3):e58837.
23. Risacher SL, McDonald BC, Tallman EF, et al. Association Between Anticholinergic Medication Use and Cognition, Brain Metabolism, and Brain Atrophy in Cognitively Normal Older Adults. JAMA Neurol. 2016;73(6):721-32.
24. Caamaño-Isorna F, Corral M, Montes-Martínez A, Takkouche B. Education and dementia: a meta-analytic study. Neuroepidemiology. 2006;26(4):226-32.
25. Erickson KI, Voss MW, Prakash RS, et al. Exercise training increases size of hippocampus and improves memory. Proc Natl Acad Sci U S A. 2011;108(7):3017-22.
26. Miller JW, Harvey DJ, Beckett LA, et al. Vitamin D Status and Rates of Cognitive Decline in a Multiethnic Cohort of Older Adults. JAMA Neurol. 2015;72(11):1295-303.
27. Kantarci K, Lowe VJ, Lesnick TG, et al. Early Postmenopausal Transdermal 17β-Estradiol Therapy and Amyloid-β Deposition. J Alzheimers Dis. 2016;53(2):547-56.
28. Tully PJ, Dartigues JF, Debette S, Helmer C, Artero S, Tzourio C. Dementia risk with antihypertensive use and blood pressure variability: A cohort study. Neurology. 2016;87(6):601-8.
29. NorthShore University HealthSystem. http://www.northshore.org/. [Accessed September 1, 2016]
30. Satizabal CL, Beiser AS, Chouraki V, Chêne G, Dufouil C, Seshadri S. Incidence of Dementia over Three Decades in the Framingham Heart Study. N Engl J Med. 2016;374(6):523-32.
APPENDIX