M. Mc Carthy, P. Schueler
ICON plc, Dublin, Ireland
Corresponding Author: Marie Mc Carthy, ICON plc Dublin, Ireland, marie.mccarthy@iconplc.com
J Prev Alz Dis
Published online September 9, 2019, http://dx.doi.org/10.14283/jpad.2019.32
Abstract
The report explores the potential digital technology has to generate novel endpoints and digital biomarkers for Alzheimer’s disease drug development studies. Drawing from literature and novel pilots, we explore the value of innovative digital technology to digitize physiological behaviours such as sleep disturbance and gait changes. Technology now exists to monitor and quantify our use and interaction with electronics in the home, the use of social platforms and smart-phones, geolocation, sleep and activity patterns. These multimodal digital data are a feasible alternative to capturing the more complex activities of daily living that require higher cognitive processes and are a sensitive predictor of disease. The combination of biosensors and the internet of things (IoT), offers the potential to collect highly relevant, objective data in a continuous, passive and low burden manner. Digital endpoints and biomarkers could have value in the diagnosis, monitoring and development of therapies for patients living with Alzheimer’s disease.
Key words: Digital Biomarkers, Clinical trials, Ecological Momentary Assessment, Gait, ADL, Alzheimer’s Disease, Smartphone.
What is the Problem?
There have been no new Alzheimer’s disease drug therapies on the market in over a decade. Alzheimer’s disease is a complex multifactorial disease and there are many reasons proposed for this stasis, including limited validated drug targets, lack of reliable surrogate biomarkers, slow and variable disease progression, and the dependency on soft endpoints (1). The limited sensitivity of existing tools to detect and monitor Alzheimer’s disease is compounded by the narrow set of outcomes (2). These limitations become even more impactful in trials of disease-modifying therapies; current measures of cognition are not sensitive in individuals with very early stages of the disease (3) and profoundly affect the value of these assessments in studies where subjects with no or minimal symptomatology are followed for several years before they may reach a pre-defined outcomes.
The Solution?
In its most recent, draft Guidance the Food and Drug Administration (FDA) (4) accepts that cognition, in its entirety, encompassing all its constituent processes and domains, is meaningful in terms of daily function. However, it caveats this with the following statement that reinforces the dilemma facing the industry: “when measured using conventional approaches with sensitive tools directed at particular cognitive domains, the meaningfulness of measured changes may not be apparent.” This still leads to a certain need for co-primary endpoints where cognitive change needs to be accompanied by a benefit reflected by an independent endpoint assessing daily function, operationalized as “Activities of Daily Living” (ADL). The more complex activities linked to independent living are assessed by Instrumental Activities of Daily Living (IADLs) and include items such as housework, communication using computer and telephone, food preparation etc. There is a growing body of evidence that subtle deficits in IADL, particularly those that are performance based, are more sensitive to early cognitive decline and may be present in mild cognitive impairment (MCI) (5, 6).
Smart Home Technology
Smart home technology is readily available, combining sensors and connected devices that monitor and control the use of appliances in the home (7). These systems are a network of connected technologies that can monitor a number of activities in the home including; the opening and closing of doors, movement in specific locations, heat and light and the presence or absence of an individual. In a short pilot, we combined smart home data (Table 1) with actigraphy data to explore the potential to generate insights more usually collected by questionnaires (8). Connected home systems are already utilized by health agencies to support older adults living in the community (9) as part of healthy aging programs for safety and health monitoring (10). There are significant possibilities for their use in drug development studies by providing continuous data to generate novel endpoints that have the potential to be more sensitive to change than existing methodologies. Digital technologies could benefit Alzheimer’s disease research by generating a more patient centric assessment by removing domains from questionnaires better captured by passive digital technologies (Table 1). These new multi-modal assessments could facilitate the capture of complex digital IADL’s, such as the ability to use a smartphone, conduct online banking, social media interaction etc. New composite digital endpoints could emerge by mapping the discordance between subjective data of the individual’s perceived behaviour and their objective data as gathered by sensors. Finally the high number of data points reduces the bias from rare samples during the pre-scheduled on site study visits, what should increase the robustness and reliability of the data, ultimately leading to less data variability (11).
Gait
There is growing interest in gait change as a marker for cognitive decline. Reports of gait disturbances have been found to precede dementia by more than 5 years (12, 13). While the use of wrist or ankle worn physical activity monitors (PAM) to collect steps and gait cadence is well established, assessing spatiotemporal gait is not a simple process and is limited to specialist clinics equipped with electronic walkways. This significantly affects the utility of this approach in clinical trials due to the limited number of sites available for gait assessments.
New technology such as smart-insoles is emerging. In a recent pilot, we used smart insoles to quantify gait speed and stride variability (14) in a non-clinical setting. The potential value of smart-insoles is in the portability of the technology, enabling their use outside of specialist gait clinics and thereby monitoring gait change in the individual’s home or residential care setting. This has the potential to capture more nuanced assessment of gait change, including balance, inter-gait variability and even stance. These devices generate vast quantities of data leading to the possibility of using machine learning to identify new clinical sensitive signals within the data set. However it was outside the scope of our pilot study to determine the minimal clinically important differences (MCID) for cognitive decline. In addition, it should be noted that factors such as footwear and data transfer could impact the operationalization of these devices in a clinical trial.
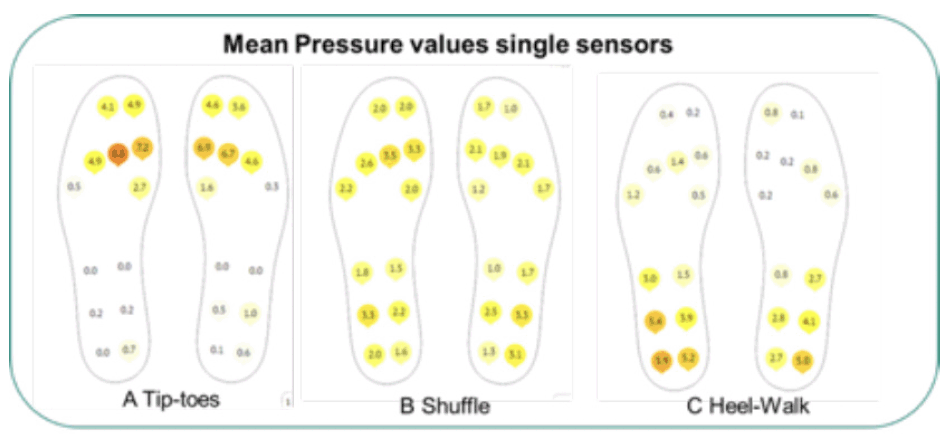
Figure 1. The smart insoles generate data from 13 embedded tri-axial accelerometers each capable of generating 100hertz data
The static report shows pressure distribution, single pressure values and total forces for the single sensors embedded in the insoles when a healthy volunteer engaged in different patterns of walking.
Smart Phones and Smart watch
The smartphone and smartwatch are emerging as significant digital tool for the collection of disease specific biomarkers and endpoints for Alzheimer’s disease. Smartphone are widely available and have an array of inbuilt technology including accelerometers, gyroscopes, magnetometers, global positioning system (GPS), proximity sensors, ambient light sensors, microphones, cameras, touch-screen sensors. These sensors facilitate the capture a multitude of data including; activity, cadence, speech, tremor and location. Smartphones can facilitate the ready deployment of a growing array of applications (apps) and can be to deploy cognitive assessments and gamification. Used outside of the clinic as screening tools, frequent burst cognitive assessments have the potential to make results more reliable and can potentially offer a means of continuous longitudinal monitoring. Changes in language and voice are being evaluated as predicators of disease progression (15, 16) with the goal to develop smartphone apps that could be used to for this purpose.
Smartwatches are evolving from simple actigraphy devices that measure sleep and activity to biosensors that that contain an array of sensors including photoplethysmography (PPG) and electrodermal activity (EDA) sensors. These biosensors can generate a myriad of endpoints including sleep disturbance, activity, heart rate, respiration rate, oxygen saturation and galvanic skin response. These biosensors could have particular utility in the ongoing research into the influence of cardiovascular factors on the development and progression of AD (17). It is entirely feasible that biosensors capable of continuously monitoring cardiovascular and respiratory signals could play an increasingly significant role as low burden tools to generate new targets for treatment and prevention of AD.
What does the future look like
Clinical development programs for Alzheimer’s disease are becoming larger and longer; the sustainability of existing methodologies and study designs is questionable. There is growing interest in the use of digital technology and exploring the transformative potential of these technologies as a means of providing additional insights (18).
The value of actigraphy devices in the study of this population has been previously discussed (19) and this report focuses on primarily on two areas gait assessment and IADL where digital technology could have a significant impact on patient centric trial design and the generation of new digital endpoints. There is significant potential for the use of connected devices and IoT, particularly for disease-outcome driven prevention studies. These sensors are gaining acceptance by health care systems as a means of keeping older adults in the community. They are relatively easy to install and can passively capture individuals’ behaviour as they go about their normal activities of daily living. These use cases are ensuring that the systems are becoming more robust in terms of connectivity and compliant in terms of data privacy and security and more robust for the generation of data for use in clinical trials.
There is potential value in combining data from multimodal digital devices and developing composite end-points that are more responsive to change then viewing each dataset as a singularity. Advanced analytic platforms that use artificial intelligence and machine learning are available that can ingest data from multiple sources including; sensors, smartphone, smart-home, environmental, geolocation, voice and questionnaires, generating insights into behavioural changes and cognitive decline. The correlation of these data with clinical observations and laboratory biomarkers could help characterise the populations, monitor progression over time and assess the efficacy of interventions.
What more needs to be done
As with any new outcome assessment, digital endpoints need to be clinically validated. The technology is required to measure endpoints that are both meaningful to the patient and clinically relevant. The data generated by the digital assessment needs to capture the concept of interest that reflects the meaningful health aspect for individuals living with Alzheimer’s disease. In addition, the data generated needs to measure meaningful change that is consistent across specific populations. The same scientific rigor and data quality criteria are required whether considering digital or traditional methodologies. The digital technology need verification and validation to ensure there is sufficient scientific evidence to support its use in a specific study (20). Data privacy, security and storage need to align with local regulations. The potential of Digital Biomarkers and endpoints is immense, however, if a digital strategy is to be successful, the inclusion of a conscious patient centric approach including strategies to quantify and reduce patient burden and ensure patient engagement is essential.
Conflict of Interest: There are no conflicts of interest.
Open Access: This article is distributed under the terms of the Creative Commons Attribution 4.0 International License (http://creativecommons.org/licenses/by/4.0/), which permits use, duplication, adaptation, distribution and reproduction in any medium or format, as long as you give appropriate credit to the original author(s) and the source, provide a link to the Creative Commons license and indicate if changes were made.
References
1. Schueler P, Siegfried K and Hüll M. Lessons Learned from Major Clinical Trials Conducted Over the Past Decades; in M Bairu; Alzheimer’s Disease Trials, Elsevier, 2013.
2. Schneider LS1, Mangialasche F, Andreasen N, et al. Clinical trials and late-stage drug development for Alzheimer’s disease: an appraisal from 1984 to 2014. J Intern Med 2014 March; 2014;275(3): 251–283. doi:10.1111/joim.12191, 251-283.
3. Vellas R, Bateman K. Blennow, G et al. Endpoints for Pre-Dementia AD Trials: A Report from the EU/US/CTAD Task Force. J Prev Alzheimers Dis. 2015;Jun; 2(2): 128–135.
4. U.S. Department of Health and Human Services, Food and Drug Administration, Center for Drug Evaluation and Research (CDER), 2018. Center for Biologics Evaluation and Research (CBER) Early Alzheimer’s Disease: Developing Drugs for Treatment Guidance for Industry 01/29/18. Revision 1. https://www.fda.gov/downloads/Drugs/GuidanceComplianceRegulatoryInformation/Guidances/UCM596728.pdf. Accessed 11/02/2019
5. Mlinac ME, F. M. Assessment of Activities of Daily Living, Self-Care, and Independence. , Volume 31, Issue 6, 1 September 2016, Pages 506–516,. Archives of Clinical Neuropsychology, 2016;506-516.
6. Jekel K., Damian M, Wattmo C et al. Mild cognitive impairment and deficits in instrumental activities of daily living: a systematic review 2015;7:17. . Alzheimer’s Disease Research and therapy .
7. Wang Ju, Bauer J, Becker M et al, A novel approach for discovering human behavior patterns using unsupervised methods. Z Gerontol Geriat 2014;47:648–660
8. Mc Carthy M, Walsh D, Tallon J, et al . Can wearables and sensor data be used to add context to activities of daily living questionnaires? Qual Life Res 2018;27(Suppl 1): 1 S159.
9. Bipartisan Policy Centre. Healthy Aging Begins at Home. https://bipartisanpolicy.org/wp-content/uploads/2016/05/BPC-Healthy-Aging.pdf.accessed 2016;11/02/2019
10. Azimi I, Rahmani A M, Liljeberg P et al. Internet of things for remote elderly monitoring: a study. J Ambient Intell Human Comput, 2016.
11. Dodge HH, Zhu J, Mattek NC, et al, Use of high-frequency in-home monitoring data may reduce sample sizes needed in clinical trials. PLoS One 2015;10:e0138095.
12. Deschamps T, Beauchet O, Annweiler C, et al. Postural control and cognitive decline in older adults: position versus velocity implicit motor strategy. Gait Posture. 2014;39(1):628-30
13. Beauchet O, Allali G, Launay C, et al. Gait variability at fast-pace walking speed: a biomarker of mild cognitive impairment? Neurol Sci. 2013; Aug;34(8):1275-82.
14. Mc Carthy M, Gon C. Can digital footprints capture clinically relevant gait endpoints in non clinical setting: A proof of concept. The Journal of Prevention of Alzheimer’s Disease – JPAD Volume 5, Supplement 1, 2018 S186.
15. Au R, Piers RJ, Devine S. How Technology is Reshaping Cognitive Assessment: Lessons from the Framingham Heart Study. Neuropsychology. 2017 ;31(8): 846–861.
16. Martínez-Sánchez F, Meilán JJG, Carro J, et al, A Prototype for the Voice Analysis Diagnosis of Alzheimer’s Disease. J Alzheimers Dis. 2018;64(2):473-481
17. Santos CY, Snyder PJ, Wu W et al. Pathophysiologic relationship between Alzheimer’s disease, cerebrovascular disease, and cardiovascular risk: A review and synthesis. Alzheimers Dement (Amst). 2017; 7: 69–87.
18. Gold M, Amatniek J, Carrillo MC, et al. Digital technologies as biomarkers, clinical outcomes assessment, and recruitment tools in Alzheimer’s disease clinical trials. Alzheimers Dement 2018; 4: 234–242.
19. M. Mc Carthy, W. Muehlhausen, P. Schüler. The case for using actigraphy generated sleep and activity endpoints in Alzheimer’s Disease clinical trials. J Prev Alz Dis 2016;3(3):173-176
20. https://www.ctti-clinicaltrials.org/sites/www.ctti-clinicaltrials.org/files/mobile-devices-recommendations.pdf#Pg18Ln30 accessed 11/02/2019
Published online September 9, 2019, http://dx.doi.org/10.14283/jpad.2019.32