P. Daunt1, C.G. Ballard2, B. Creese2, G. Davidson3, J. Hardy4, O. Oshota1, R.J. Pither1, A.M. Gibson1 for the Alzheimer’s Disease Neuroimaging Initiative*
1. Cytox Limited, Manchester, UK; 2. University of Exeter Medical School, Exeter, UK; 3. Ledcourt Associates Limited, Cambridge, UK.; 4. UK Dementia Research Institute, University College London, London, UK.
Corresponding Authors: Alex Gibson, Cytox Ltd., John Eccles House, Robert Robinson Avenue, Oxford Science Park, Oxford, OX4 4GP, United Kingdom. Email: alex.gibson@cytoxgroup.com. Tel:+44 (0)1865 338018
J Prev Alz Dis 2021;1(8):78-83
Published online November 11, 2020, http://dx.doi.org/10.14283/jpad.2020.64
Abstract
BACKGROUND: There is a clear need for simple and effective tests to identify individuals who are most likely to develop Alzheimer’s Disease (AD) both for the purposes of clinical trial recruitment but also for improved management of patients who may be experiencing early pre-clinical symptoms or who have clinical concerns.
OBJECTIVES: To predict individuals at greatest risk of progression of cognitive impairment due to Alzheimer’s Disease in individuals from the Alzheimer’s Disease Neuroimaging Initiative (ADNI) using a polygenic risk scoring algorithm. To compare the performance of a PRS algorithm in predicting cognitive decline against that of using the pTau/Aß1-42 ratio CSF biomarker profile.
DESIGN: A longitudinal analysis of data from the Alzheimer’s Disease Neuroimaging Initiative study conducted across over 50 sites in the US and Canada.
SETTING: Multi-center genetics study.
PARTICPANTS: 515 subjects who upon entry to the study were diagnosed as cognitively normal or with mild cognitive impairment.
MEASUREMENTS: Use of genotyping and/or whole genome sequencing data to calculate polygenic risk scores and assess ability to predict subsequent cognitive decline as measured by CDR-SB and ADAS-Cog13 over 4 years
RESULTS: The overall performance for predicting those individuals who would decline by at least 15 ADAS-Cog13 points from a baseline mild cognitive impairment in 4 years was 72.8% (CI:67.9-77.7) AUC increasing to 79.1% (CI: 75.6-82.6) when also including cognitively normal participants. Assessing mild cognitive impaired subjects only and using a threshold of greater than 0.6, the high genetic risk participant group declined, on average, by 1.4 points (CDR-SB) more than the low risk group over 4 years. The performance of the PRS algorithm tested was similar to that of the pTau/Aß1-42 ratio CSF biomarker profile in predicting cognitive decline.
CONCLUSION: Calculating polygenic risk scores offers a simple and effective way, using DNA extracted from a simple mouth swab, to select mild cognitively impaired patients who are most likely to decline cognitively over the next four years.
Key words: Polygenic risk, cognitive decline, Alzheimer’s disease.
Introduction
Alzheimer’s disease (AD) is the most common form of dementia with nearly 50 million people affected globally and an estimated economic impact of $818 billion (1).
As well as having a clear heritable component (2), AD is genetically complex. Neuropathologically, the disease is characterized by extracellular senile plaques containing β -amyloid (Aβ) and intracellular neurofibrillary tangles containing hyperphosphorylated tau protein. A relatively small number of dominant mutations in the amyloid precursor and presenilin genes are known to cause early onset Alzheimer’s disease. Over the past two decades, genome wide association studies (GWAS) have identified multiple loci and single nucleotide polymorphisms (SNPs) associated with the much more common, late-onset or sporadic form of the disease (LOAD) (3-5). Apolipoprotein E (ApoE) is a major cholesterol carrier that supports lipid transport and injury repair in the brain. The ε4 allele of ApoE (ApoE4) has been found to be a primary genetic risk factor for AD, associated with increased risk for both early-onset AD and LOAD (6, 7). Although only 20-30% of humans are ApoE4 carriers, these individuals account for up to 60% of all Alzheimer’s disease cases. In addition, ApoE4 is associated with an increased risk of lower age of onset (8, 9), making this an important subset of the population at high risk of developing AD.
Development of polygenic risk scoring (PRS) algorithms that can capture all the genetic contribution towards the risk of developing AD (10) is an attractive strategy to allow better clinical trials for AD prevention. PRS approaches have demonstrated accuracies of between 75 and 84% for predicting onset of AD when including APOE, sex and age in addition to PRS (11), In particular the PRS approach as developed by Escott-Price et al (12), is built as a sum of the weighted contributed of 10,000s of SNPs where the weights are the β-coefficients of each SNP association with the disease. In contrast to other PRS algorithms, where fewer SNPs have been used (for example just 31 SNPs (13)) this approach includes SNPs that are not considered as having genome wide significance in GWAS studies. However, inclusion of this vastly increased number of variants which alone carry sub-threshold significance provides an additive contribution to the overall performance that may be substantive and also reduce risk that performance is not lost when being applied across different cohorts.
Until now the analyses performed using this particular approach have been carried out to predict those individuals diagnosed with AD or MCI (14) versus those who are cognitively normal, though PRS algorithms have been used to look at a variety of AD pathology and risk by Altmann et al (15). Here we look to see how the PRS performs in predicting those individuals most likely to decline cognitively independent of whether they have cognitive impairment on entry or not.
Currently, the most frequently used approach to enrich clinical trial recruitment with participants who have increased likelihood of progressive cognitive and functional decline has been to focus on identifying individuals who are positive for amyloid biomarkers. In addition, measurement of tau in CSF often with amyloid levels, is increasing in use. We therefore also compare the ability to predict decline using PRS against that of using CSF tau and amyloid measurements.
Methods
Data used in the preparation of this article were obtained from the Alzheimer’s Disease Neuroimaging Initiative (ADNI) database (adni.loni.usc.edu). The ADNI was launched in 2003 as a public-private partnership, led by Principal Investigator Michael W. Weiner, MD. The primary goal of ADNI has been to test whether serial magnetic resonance imaging (MRI), positron emission tomography (PET), other biological markers, and clinical and neuropsychological assessment can be combined to measure the progression of mild cognitive impairment (MCI) and early Alzheimer’s disease (AD).
Sample Description
ADNI is an on-going longitudinal study that has been established to develop methods for early detection of AD and subsequent monitoring of disease trajectory using clinical, imaging and genetic data (16). Data for this analysis was collected from 515 participants, who entered the study with a diagnosis of Mild Cognitive Impairment or considered cognitively normal. In addition, 47 individuals diagnosed with AD were used to check the algorithm was performing as expected to differentiate AD cases from cognitively normal controls. All participants in addition to having suitable genetic data available had at least 4 years’ worth of follow up cognitive testing and imaging scans. Upon entry into the study 199 individuals were cognitively normal and 316 diagnosed as MCI. The average age of the total group was 73.2 years, with the CN group being on average approximately 3 years older than those diagnosed with MCI (75.1y and 72.0 y respectively). ADAS-Cog13 scores for cognitively normal and MCI groups upon entry were 9.0 and 14.9 respectively and at the 4 year assessment the average scores obtained were 9.6 and 19.8, clearly showing that on average the MCI group continued to decline compared with little change in the average score of the CN group. CSF biomarker data were not available for all participants, so analyses performed to compare PRS with biomarker (tau and amyloid) as a predictor for subsequent cognitive decline were carried out on 290 MCI subjects. Table 1a shows the classification of the ADNI dataset at baseline and changes to cognitive performance as measured by ADAS-Cog13 after 4 years. Similarly, Table 1b describes the sub-group that also had CSF biomarker data available.
Genotyping Procedures and Quality Control
The ADNI samples were genotyped using with Whole Genome Sequencing and/or the Illumina Omni 2.5M BeadChip array. Quality control checks were performed using PLINK software (www.cog-genomics.org/plink/2.0/) (17). Checks included exclusion of SNPs with missingness greater than 0.02, minor allele frequency of less than 0.01 and SNPs with Hardy-Weinberg equilibrium p-value less than 1 x 10-6 were also excluded. After such checks 8,990,292 SNPs were left for analysis of which approximately 114,000 were used as part of the polygenic risk scoring algorithm.
Calculation of Polygenic Risk Scores
A specifically built, proprietary software called SNPfitR was used for all subsequent PRS calculations. The PRS calculations are based on a pre-determined logistic regression model based on the modelling of the association between the incidences of variants within a large panel of SNPs with a known links to AD to the presence of the disease in a substantial cohort of subjects (Escott-Price et al12). Subject age, gender and presence of both APOE4 and APOE2 proteins are included as covariates. The software calculates the normalised sum of the individual scores weighted by their effect sizes for each SNP, adds the values for the covariates and derives the predicted risk from the model equation.
Effect sizes were determined from the IGAP study. The score contribution from SNPs with missing values were imputed based on the population frequency of the effect allele for that SNP.
Statistical Analysis
The polygenic risk scores generated were exported for the analysis presented.
The statistical analysis software package JMP 14.1.0 was used to carry out all data manipulation and analysis. The ROC analysis and AUC calculations were performed using the add in ‘Model Classification Explorer’. Values were cross checked with the AUC calculations carried out in the software.
Results
Association of AD PRS with onset of Alzheimer’s Disease
As a check that the algorithm was performing as expected polygenic risks scores were also generated for 47 Alzheimer Disease cases and compared with those generated for the 199 cognitively normal individuals. The accuracy of prediction of clinical AD cases (n = 47) versus cognitively normal control (n = 199) was 80% AUC. Furthermore, as shown in Figure 1, PRS score is clearly associated with tau levels and, as expected, diagnostic classification. In this analysis, data were plotted in this heat density format to illustrate a clear relationship between the pTau/Aß1-42 ratio (Hansson et al18) and the stage of disease. It can be seen that those subjects classified at AD, late-MCI (LMCI) or early-MCI (EMCI), predominantly sit in the group with a PRS of 0.6 or above, whereas cognitively normal (CN) individuals tend to be in the 0.6 or lower range of the PRS scores. Importantly, as shown in Figure 2, there is a substantial overlap between different APOE genotype sub-groups. As expected, ApoE4 carriers fall within the higher end (0.6 and above) end of the PRS range and ApoE2 carriers at the lower end. However, ApoE3/3 homozygotes, representing some 60% of the Caucasian population, span the high and low ends of the PRS spectrum, thereby demonstrating the additional genetic risk information provided by the risk algorithm over APOE alone.
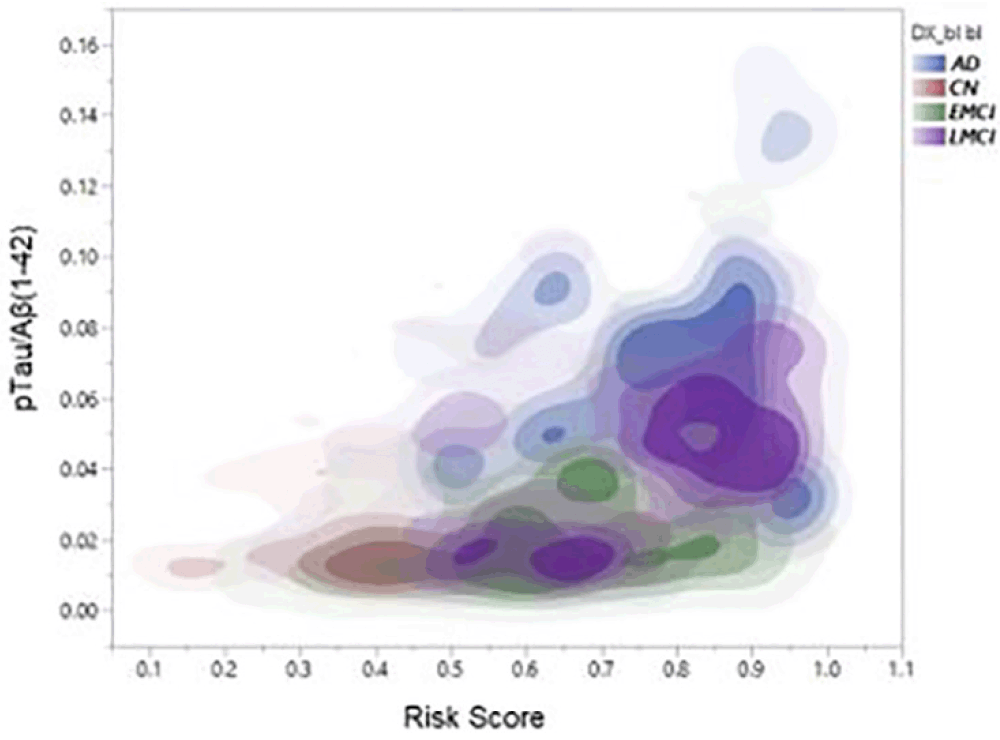
Figure 1. Density plots showing relationship between full PRS, pTau/Aβ(1-42) ratio and diagnostic classification (CN, EMCI, LMCI, AD)
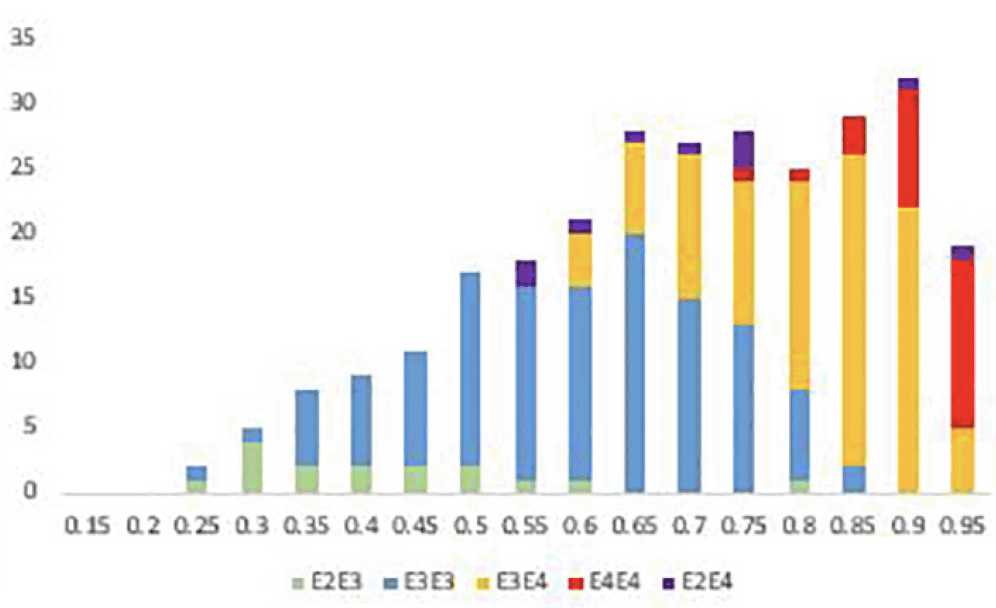
Figure 2. Distribution of risks scores across the MCI population (n = 316) coloured by APOE genotype
Association of AD PRS with cognitive decline from an MCI baseline
Rather than using specific clinical diagnosis to categorise cases as previously used, predicting the cognitive decline likely due to AD from either an MCI or cognitively normal baseline was performed. Table 2 shows the predictive accuracy of identifying those individuals who are most likely to decline as measured by ADAS-Cog13 testing from an MCI or CN baseline, irrespective of cognitive status at baseline. The analyses were performed defining significant progression as 5-point, 10-point or 15-point decline at their 4 year follow up assessment. Though we report the accuracy for predicting decline from a cognitively normal state, the number of individuals that decline significantly within the time period is relatively low and thus results cannot be considered statistically significant. However, of the 316 individuals who entered the study with an MCI diagnosis, significant numbers had declined by at least 5 points (107), 10 points (61) and 15 points (39) on the ADAS-Cog13 scale to allow meaningful prediction accuracies to be measured. In addition to the full PRS algorithm (APOE + PRS + Age + Sex) being used to generate risk scores, prediction accuracies based on APOE status alone and total genetic risk (APOE + PRS) were calculated. The best prediction accuracy is seen for testing cases that have declined by at least 10 points at 4 years versus those that have remained cognitively stable (< 5-point decline) with an AUC of 74.8%, compared with 67.4% for APOE alone and 73.5% for APOE + PRS. A similar performance is seen when predicting those individuals with 15-point decline. In both analyses all those individuals had polygenic risk scores in the upper half of the distribution. When looking at smaller changes in cognitive performance over 4 years, addition of the polygenic risk score term to APOE did not impart greater performance. In all cases addition of age and gender as co-variates did not add any additional predictive performance in this particular group presumably due to the particular age and sex distribution between the CN and MCI groups in this particular cohort. Given that the mean age of those that declined and those that remained relatively stable were similar the contribution provided by age to the overall risk score for both groups would, in turn, be broadly equivalent.

Table 2. Performance of polygenic risk scoring algorithm to predict cognitive decline up to 4 years after entry to study
* PRS = all risk associated with the genetics other than that contribution from APOE
To evaluate whether the full algorithm could predict cognitive decline as defined by predetermined thresholds and be compared with that predicted by CSF biomarker status (figure 3), the MCI population where both genetics, CSF and CDR-SB assessment data were available was studied (n=290). There was a significant difference in progression (as defined by CDR-SB) between patients whose risk score was greater than 0.6 (n=196) versus the group whose score was less 0.6 (n=94) as early as 6 months after baseline assessment. 0.6 was chosen as a threshold based on an optimal balance between sensitivity and specificity (data not shown here) High risk patients progressed, on average, by approximately 1 point over 24 months and 2 points over 48 months compared with low risk patients who on average decline 0.2 and 0.4 points over the same timepoints. A similar evaluation was carried out to compare the predictive performance using CSF biomarker positivity as determined by a pTau/Aβ(1-42) ratio using the cut off of 0.02818 and CSF Aβ(1-42) with a threshold of 880pg/mL18. Again, there was a significant difference in progression between biomarker positive and negative patients. pTau/Aβ(1-42) ratio positive patients progressed, on average, by 1.1 and 2.9 points over 24 and 48 months respectively, whereas there was an average decline of 0.1 and 0.2 points for the negative group. Similarly using Aβ(1-42) CSF levels only, the amyloid positive group progress by 1 and 2.6 points at 24 and 48 months respectively whilst the negative group only progressed by 0,3 points on average over 48 months. The performance of the PRS was broadly similar to that of either CSF biomarker measurement in identifying those subjects at highest and lowest risk of cognitive decline on the CDR-SB scale. Furthermore, a similar analysis was performed on APOE3 homozygote individuals (n=125) only (figure 3). Again, using a threshold of 0.6 to determine the high risk group (n =49), a difference a measured by a change of CDR-SB between the two groups was shown 12 months with a clear difference at 36 months. The high risk group declined, on average by 1.5 points at 36 months compared with the low risk group who only declined, on average, by 0.5 points.
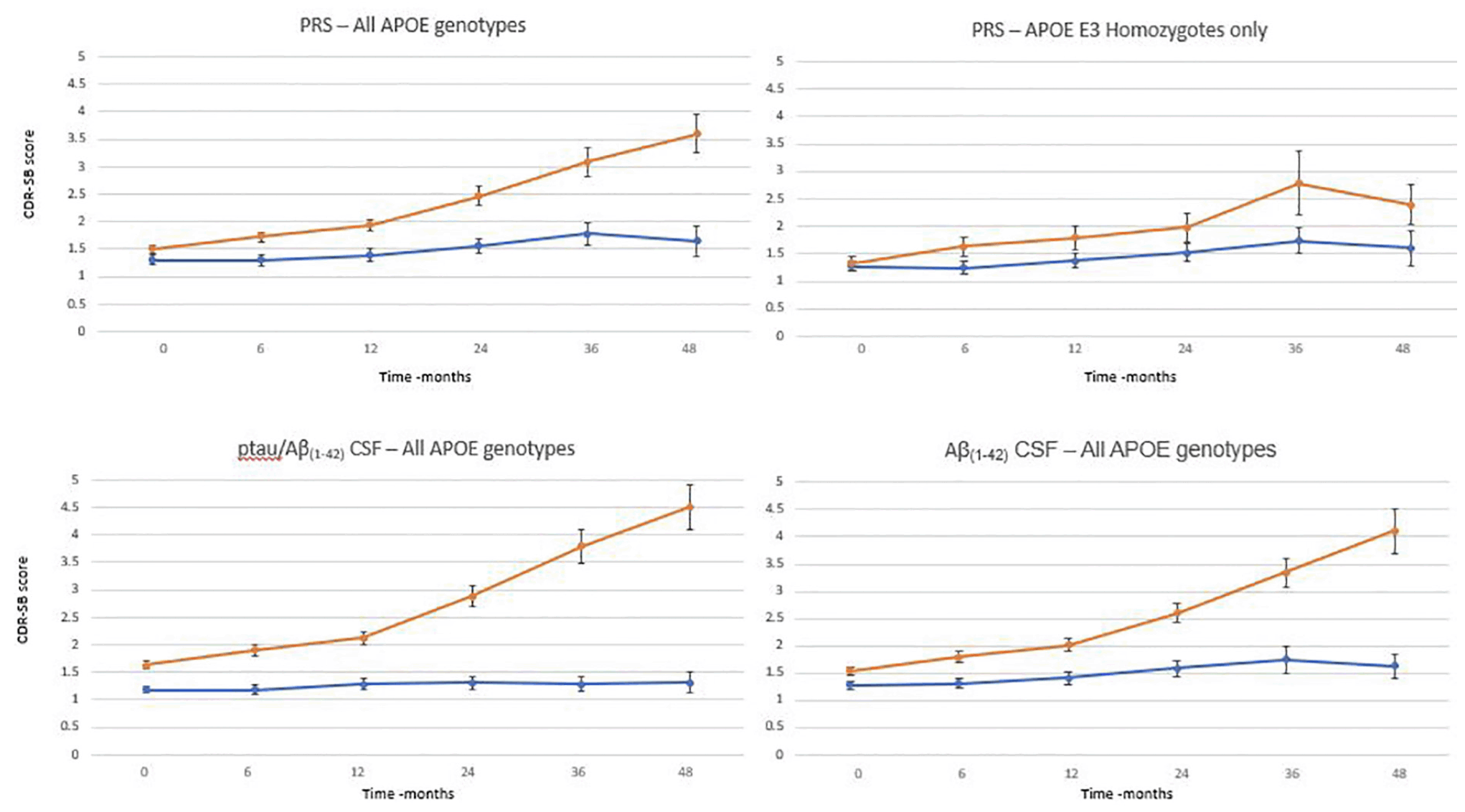
Figure 3. Time course of clinical progression in patients with MCI over 48 months. Average with standard errors by PRS group (orange >0.6; blue <0.6 at baseline) for all APOE genotypes and for APOE homozygotes only, pTau/Aβ(1-42) group (orange > 0.028; blue <0.028) and Aβ(1-42) (orange < 880pg/mL; blue >880pg/mL)
Discussion
This study was designed to demonstrate the potential utility of a specific PRS algorithm in identifying individuals at highest risk of clinically significant cognitively decline within a specific time period. Previously most studies reporting the use of PRS approaches have been used to differentiate two populations with clearly different clinical phenotypes (AD versus CN) and thus not necessarily demonstrating how this approach could be used prospectively. The results of these analyses show that using polygenic scoring algorithms which have been designed to understand the genetic risk of future onset of Alzheimer’s Disease, can be applied to enrich trial populations with individuals who are more likely to decline cognitively within a certain time period.
Though APOE genotype remains an important genetic risk factor within this cohort, it is clear there is an additional genetic component that should be considered in assessing genetic risk. This will subsequently allow further risk stratification within APOE genotypes such as identifying APOE3 homozygotes who are at relatively higher risk even compared with some APOE4 carriers. This has implications in the design of clinical trials where in many trial designs possession of at least one APOE4 allele is used as an enrichment strategy in prevention trials.
It is broadly accepted that CSF-tau/amyloid ratios are a reasonable predictor of future cognitive decline (18-20) though definitive studies have yet to be performed, and testing for amyloid alone, via PET imaging or CSF remains the standard method to enrich trials with patient most likely to decline cognitively. This study shows that PRS predictions, are able to perform to a similar level in predicting further progression, as measured by CDR-SB, in patients who have an MCI diagnosis. Importantly this genetic risk assessment can be more easily accessed (cost and patient burden) through whole blood or mouth swab testing, rather than by performing an invasive lumbar puncture procedure and subsequent CSF testing; such invasive procedures are particularly challenging in elderly subjects who may be relatively cognitively robust (early MCI or prodromal). The PRS algorithm therefore represents a promising method to facilitate broad screening of potential trial participants in order to identify those at highest risk for cognitive decline. Further confirmatory testing, via the use of more invasive and expensive CSF and/or PET imaging, could then be focussed on a significantly reduced number of individuals for final patient recruitment decisions. Furthermore, a combination of PRS and tau levels (underlying genetic risk coupled with manifestation of that risk through pathology) may provide a more optimal model for likelihood of subsequent onset of AD in early symptomatic or pre-symptomatic individuals. Whilst there may be specific reasons why amyloid or tau biomarkers may be required for clinical trials focussing on treatments specifically targeting amyloid or tau, PRS may have advantages for therapies with different treatment targets independent of potential mechanisms.
Further studies will be important to determine the added value of combing amyloid/tau and PRS markers and to fully determine the utility of PRS in predicting cognitive decline in cognitively normal individuals.
It is recognised that this work has considered genetic risk together with age and gender in developing a model for predicting further development of cognitive symptoms and so does not consider other risk factors that are known to influence onset and development of disease. Combining both genetic and lifestyle risk factors for the purposes of identifying those individuals most at risk of Alzheimer’s Disease is likely to add further to the predictive accuracy.
Study Limitations
This study is not without limitations, with sample size being the primary shortcoming. This was particularly relevant in evaluating the APOE E3 homozygote only sub-group. Furthermore, studies with larger sample sizes across all diagnostic categories, including those declining from a cognitively normal baseline, is important to understand broader utility. As with most studies of this nature, observing similar performance in alternative cohorts is important and is critical towards the understanding and confirmation of polygenic risk score assessment for use in clinical trial recruitment and in clinical practice.
*Data used in preparation of this article were obtained from the Alzheimer’s Disease Neuroimaging Initiative (ADNI) database (adni.loni.usc.edu). As such, the investigators within the ADNI contributed to the design and implementation of ADNI and/or provided data but did not participate in analysis or writing of this report. A complete listing of ADNI investigators can be found at: http://adni.loni.usc.edu/wp-content/uploads/how_to_apply/ADNI_Acknowledgement_List.pdf
Acknowledgements: Data collection and sharing for this project was funded by the Alzheimer’s Disease Neuroimaging Initiative (ADNI) (National Institutes of Health Grant U01 AG024904) and DOD ADNI (Department of Defense award number W81XWH-12-2-0012). ADNI is funded by the National Institute on Aging, the National Institute of Biomedical Imaging and Bioengineering, and through generous contributions from the following: AbbVie, Alzheimer’s Association; Alzheimer’s Drug Discovery Foundation; Araclon Biotech; BioClinica, Inc.; Biogen; Bristol-Myers Squibb Company; CereSpir, Inc.; Cogstate; Eisai Inc.; Elan Pharmaceuticals, Inc.; Eli Lilly and Company; EuroImmun; F. Hoffmann-La Roche Ltd and its affiliated company Genentech, Inc.; Fujirebio; GE Healthcare; IXICO Ltd.; Janssen Alzheimer Immunotherapy Research & Development, LLC.; Johnson & Johnson Pharmaceutical Research & Development LLC.; Lumosity; Lundbeck; Merck & Co., Inc.; Meso Scale Diagnostics, LLC.; NeuroRx Research; Neurotrack Technologies; Novartis Pharmaceuticals Corporation; Pfizer Inc.; Piramal Imaging; Servier; Takeda Pharmaceutical Company; and Transition Therapeutics. The Canadian Institutes of Health Research is providing funds to support ADNI clinical sites in Canada. Private sector contributions are facilitated by the Foundation for the National Institutes of Health (www.fnih.org). The grantee organization is the Northern California Institute for Research and Education, and the study is coordinated by the Alzheimer’s Therapeutic Research Institute at the University of Southern California. ADNI data are disseminated by the Laboratory for Neuro Imaging at the University of Southern California. We also acknowledge Prof. Julie Williams, Prof. Valentina Escott-Price, Dr Rebecca Sims and Dr Eftychia Bellou from the University of Cardiff for their advice on adaptation and implementation of of the polygenic risk algorithm.
Funding: Funding for this study was provided under an Innovate UK grant (Project No 5195).
Conflict of Interest: P. Daunt, A.Gibson, O.Oshota and R. Pither are all employees of Cytox Ltd. G. Davidson received payment from Cytox Ltd. for work done both within and outside the scope of this article.
Ethical Standards: The ADNI protocols were approved by all the Institutional Review Boards of the participating institutions. Only data from volunteers who had provided written informed consent were used to complete these analyses.
Open Access: This article is distributed under the terms of the Creative Commons Attribution 4.0 International License (http://creativecommons.org/licenses/by/4.0/), which permits use, duplication, adaptation, distribution and reproduction in any medium or format, as long as you give appropriate credit to the original author(s) and the source, provide a link to the Creative Commons license and indicate if changes were made.
References
1. Prince M, Wimo A, Guerchet M et al. World Alzheimer Report 2015: the Global Impact of Dementia – An analysis of prevalence, incidence, cost and trends. Alzheimer’s Dis Int 2015; 84:425
2. Gatz M, Reynolds CA, Fratiglioni L, et al. Role of genes and environments for explaining Alzheimer’s disease. Arch Gen Psychiatry 2006; 63: 168-174
3. Lambert JC, Ibrahim-Verbaas CA, Harold D, et al. Meta-analysis of 74,046 individuals identifies 11 new susceptibility loci for Alzheimer’s disease. Nat Genet 2013; 45: 1452-1458
4. Naj, AC, Jun G, Beecham, GW et al. Common variants at MS4A4/MS4A6E, CD2AP, CD33 and EPHA1 are associated with late-onset Alzheimer’s disease. Nat Genet 2011; 43: 436-441
5. Seshadri, S, Fitzpatrick AL, Arfan Ikram M et al. Genome-wide analysis of genetic loci associated with Alzheimer disease. JAMA 2010; 303: 1832-1840
6. Corder EH, Saunders AM, Strittmatter WJ, et al. Gene Dose of Apolipoprotein-E Type-4 Allele and the Risk of Alzheimers-Disease in Late-Onset Families. Science1993;261:921–923.
7. Farrer LA, Cupples LA, Haines JL, et al. Effects of age, sex, and ethnicity on the association between apolipoprotein E Genotype and Alzheimer Disease: a Meta-analysis. JAMA 1997;278:1349–1356.
8. Blacker D, Haines JL, Rodes L, et al. ApoE-4 and age at onset of Alzheimer’s disease: the NIMH genetics initiative. Neurology 1997;48;139-147
9. Bonham LW, Geier EG, Fan CC, et al. Age-dependent effects of APOE ε4 in preclinical Alzheimer’s disease. Ann Clin Transl Neurol 2016;3;668-677
10. Stocker H, Möllers T, Perna L, et al, The genetic risk of Alzheimer’s disease beyond APOE ε4: systematic review of Alzheimer’s genetic risk scores. Transl Psych 2018;8;166-174
11. Escott-Price V, Myers A, Huentelman AJ, et al, Polygenic risk score analysis of pathologically confirmed Alzheimer’s disease. Ann Neurol 2017;82;311-314
12. Escott-Price V, Sims R, Williams J et al, Common polygenic variation enhances risk prediction for Alzheimer’s disease. Brain 2015;138;3673-3684
13. Desikan RS, Fan CC, Wang Y et al, Genetic assessment of age-associated Alzheimer disease risk: development and validation of polygenic hazard score. PLoS Med 2017 e1002258
14. Escott-Price V, Leonenko G, Sims R et al, Polygenic risk and hazard scores for Alzheimer’s disease prediction. Ann Clin Transl Neurol 2019;6;456-465
15. Altmann A, Schott JM, Scelsi et al, A comprehensive analysis of methods for assessing polygenic burden on Alzheimer’s disease pathology and risk beyond APOE. Brain Commun 2020;2;fcz047
16. Peterson R, Aisen PS, Beckett LA et al, Alzheimer’s Disease Neuroimaging Initiative (ADNI): clinical characterization. Neurology 2010;74;201-209
17. Chang CC, Chow CC, Tellier LCAM et al, Second-generation PLINK: rising to the challenge of larger and richer datasets. GigaScience 2015;4;s13742
18. Hansson O, Seibyl J, Stomrud et al, CSF biomarkers of Alzheimer’s disease concord with amyloid-β PET and predict clinical progression: A study of fully automated immunoassays in BioFINDER and ADNI cohorts. Alzhemers Dement 2018;14;1470-1481.
19. Ritchie C, Smailagic N, Noel-Storr et al, CSF tau and CSF tau/ABeta ratio for the diagnosis of Alzheimer’s disease dementia and other dementias in people with mild cognitive impairment (MCI). Cochrane Database Syst Rev 2017;3;CD10803.
20. Wolfsgruber S, Polcher A, Koppara A et al. Cerebrospinal fluid biomarkers and clinical progression in patients with subjective cognitive decline and mild cognitive impairment. Alzheimers Dis 2017;58;939-950.