M. Ortner1, O. Goldhardt1, J. Diehl-Schmid1, I. Yakushev2, K. Lanz1, D.M. Hedderich3, E. Manuilova4,
M. Simon5, J.-P. Weinberger4, T. Grimmer1
1. Department of Psychiatry and Psychotherapy, Klinikum rechts der Isar, Technical University of Munich, School of Medicine, Munich, Germany; 2. Department of Nuclear Medicine, Klinikum rechts der Isar, Technical University of Munich, School of Medicine, Munich, Germany; 3. Department of Neuroradiology, Klinikum rechts der Isar, Technical University of Munich, School of Medicine, Munich, Germany; 4. Roche Diagnostics GmbH, Penzberg, Germany; 5. Roche Diagnostics International Ltd, Rotkreuz, Switzerland
Corresponding Author: Dr Marion Ortner, MD, Department of Psychiatry and Psychotherapy, Klinikum rechts der Isar, Technical University of Munich, School of Medicine, Ismaninger Str. 22, 81675 Munich, Germany; Telephone: +49 89 41404275; Fax: +49 89 41404923; Email: marion.ortner@tum.de
J Prev Alz Dis 2022;3(9):491-498
Published online March 4, 2022, http://dx.doi.org/10.14283/jpad.2022.27
Abstract
BACKGROUND: Alzheimer’s disease (AD) and frontotemporal lobar degeneration (FTLD) are heterogeneous in their clinical presentation and underlying pathology, but they often have overlapping features. Diagnostic accuracy is critical for guiding patient management. Cerebrospinal fluid (CSF) diagnostic assays for the differentiation of AD and FTLD may increase diagnostic accuracy.
OBJECTIVES: In this study, we aimed to understand the potential role of CSF biomarkers and biomarker ratios, measured using Elecsys® CSF immunoassays (Roche Diagnostics International Ltd, Rotkreuz, Switzerland), in the differential diagnosis of AD and FTLD.
DESIGN: This study was conducted at a single center in Munich, Germany between July 2019 and July 2020. Patient CSF samples were retrospectively collected from the study center biobank.
PARTICIPANTS: A total of 130 patients with cognitive impairment were included in the study; 86 patients were diagnosed with AD and 44 with FTLD (behavioral variant frontotemporal dementia, semantic variant of primary progressive aphasia, and non-fluent variant of primary progressive aphasia), based on core clinical criteria and a non-CSF biomarker, a typical pattern of regional hypometabolism on [18F] fluorodeoxyglucose positron emission tomography.
MEASUREMENTS: Patient CSF biomarker concentrations were measured using Elecsys CSF immunoassays. Receiver operating characteristic analyses were conducted to determine areas under the curve (AUCs) for CSF biomarker performance. Sensitivity and specificity analyses were conducted to evaluate the performance of established cut-offs (Aβ42 ≤1000 pg/mL, pTau181/Aβ42 ratio >0.024, and tTau/Aβ42 ratio >0.28) and optimized cut-offs based on Youden’s index.
RESULTS: AUC-based performance was similarly good for the pTau181/Aβ42 ratio (AUC=0.841; 95% CI: 0.759−0.923), pTau181/Aβ40 ratio (AUC=0.837; 95% CI: 0.754−0.919), Aβ42/Aβ40 ratio (AUC=0.829; 95% CI: 0.746−0.912), tTau/Aβ42 ratio (AUC=0.822; 95% CI: 0.736−0.908), pTau181/Aβ42/Aβ40 ratio (AUC=0.817; 95% CI: 0.734–0.901), and Aβ42 (AUC=0.812; 95% CI: 0.722−0.902). Performance was slightly lower for the tTau/Aβ42/Aβ40 ratio (AUC=0.799; 95% CI: 0.713−0.885), pTau181 alone (AUC=0.793; 95% CI: 0.707−0.880), tTau/Aβ40 ratio (AUC=0.751; 95% CI: 0.657−0.844), and tTau alone (AUC=0.706; 95% CI: 0.613−0.799). The highest qualitative performance was observed with the pTau181/Aβ42 ratio with an established cut-off value of >0.024 and optimized cut-off value of >0.022: sensitivity and specificity values were 0.892 and 0.773, respectively.
CONCLUSIONS: Elecsys CSF immunoassays demonstrate good diagnostic accuracy in differentiating patients with AD from those with FTLD. These immunoassays have the potential to support clinical decision making, i.e. in diagnosing patients with FTLD by excluding patients with amyloid positivity, which is indicative of underlying AD.
Key words: Alzheimer’s disease, cerebrospinal fluid assays, CSF biomarker, differential diagnosis, FDG-PET, frontotemporal lobar degeneration.
Introduction
Alzheimer’s disease (AD) is the most common form of dementia, accounting for approximately two-thirds of all cases (1). Other major forms of dementia include vascular dementia, dementia with Lewy bodies, and frontotemporal lobar degeneration (FTLD) (1). FTLD is the second most common cause of dementia in patients younger than 65 years, though 25% of FTLD cases are diagnosed in patients aged more than 65 years (2, 3). While AD and FTLD are heterogeneous in their clinical presentation and underlying pathology, they often have overlapping features (4).
AD is defined by neuropathological changes, namely neuritic plaques containing amyloid-beta (Aβ) peptides and neurofibrillary tangles containing aggregated tau proteins, which ultimately lead to neuronal injury and degeneration (5). AD is typically recognized by initial memory impairment and cognitive decline, followed by the deterioration of language and behavioral functions, visuospatial orientation, and the motor system (6). FTLD is neuropathologically defined by aggregates of tau, TAR DNA-binding protein 43, or fused-in-sarcoma protein, which are associated with degeneration of the frontal and anterior temporal lobes (7), and is typically characterized by a progressive decline in behavior, language, and executive function (2, 8). FTLD is an umbrella clinical term encompassing a group of neurodegenerative diseases, which can be classified into three clinical syndromes that are dependent on early and predominant symptoms: behavioral variant frontotemporal dementia (bvFTD), semantic variant of primary progressive aphasia (svPPA; also known as semantic dementia), and non-fluent variant of primary progressive aphasia (nfvPPA) (8). The early and predominant symptoms of bvFTD include personality changes, disinhibition, and apathy, whereas svPPA is characterized by semantic aphasia and associative agnosia, and nfvPPA is characterized by slow, labored, and halting speech production, and by omission or misuse of grammar (8). Neuropathological changes that are typical for FTLD have also been seen in movement disorders, such as corticobasal syndrome, progressive supranuclear palsy, and amyotrophic lateral sclerosis (9–11).
Differential diagnosis of AD and FTLD usually involves patients undergoing a plethora of neuropsychological tests, neurological assessments, neuroimaging, and laboratory tests (2, 8). Low concentrations of Aβ in cerebrospinal fluid (CSF) are suggestive of AD, and Aβ-specific imaging may be helpful, particularly in young patients, to rule out AD; however, there may be co-occurrence of AD and FTLD neuropathology (8). Research criteria for FTLD propose structural brain scans/imaging in order to increase diagnostic certainty, such as structural brain magnetic resonance imaging or [18F] fluorodeoxyglucose positron emission tomography (FDG-PET), with CSF biomarker analysis recommended in cases where initial investigations for FTLD are inconclusive and/or to rule out suspicion of AD (12).
As AD and FTLD have overlapping features, diagnostic accuracy is critical to enable more reliable prognostication and guide specific treatment and management (4). Differentiating between the two diseases is particularly important for treatment and management, as Aβ peptides are a hallmark of AD, but are not part of the FTLD pathologic spectrum (13). While there are symptomatic treatments available for patients with AD, currently, there are no approved treatments for patients with FTLD (though several are in development, i.e. treatments based on antisense oligonucleotides) (14–16). There are also novel disease-modifying treatments for AD (e.g. anti-Aβ drugs) in development for patients diagnosed in the early stages of the disease (17), including aducanumab, which recently received US Food and Drug Administration approval for the treatment of AD (18).
The use of CSF biomarkers to differentiate between AD and amyloid-negative diseases, such as FTLD, is not well established; however, CSF diagnostic assays for the differentiation of AD from FTLD may increase diagnostic accuracy and aid in the development of disease-modifying treatments (19). CSF biomarkers for Aβ(1–42) (Aβ42), phosphorylated tau 181 (pTau181), and total tau (tTau) have demonstrated the potential to accurately identify AD, even at the stage of incipient dementia (20,21). The fully automated Elecsys® CSF immunoassays (Roche Diagnostics International Ltd, Rotkreuz, Switzerland) quantitatively measure CSF biomarkers, and accurately detect amyloid positivity in patients with AD and those with subjective cognitive decline (SCD) or mild cognitive impairment (MCI) due to AD by determining pTau181/Aβ42 and tTau/Aβ42 biomarker ratios (22). Time taken for the CSF analyses is reduced using the Elecsys CSF immunoassays, which also demonstrate superior interlaboratory variation compared with existing manual CSF assays (coefficient of variation [CV]: 4% versus >15%) (22).
In this study, we aimed to understand the potential role of CSF biomarkers in the differential diagnosis of AD and FTLD. In a heterogeneous patient cohort and using Elecsys CSF immunoassays, we investigated whether levels of CSF biomarkers Aβ42, pTau181, and tTau, and biomarker ratios pTau181/Aβ42, pTau181/Aβ40, tTau/Aβ42, tTau/Aβ40, Aβ42/Aβ40, pTau181/Aβ42/Aβ40, and tTau/Aβ42/Aβ40, could be used to differentiate between patients with AD and those with FTLD.
Methods
Study Design and Patients
This study was conducted at a single center in Munich, Germany (the Outpatient Clinic at the Centre for Cognitive Disorders, Department of Psychiatry and Psychotherapy, Klinikum rechts der Isar, Technical University of Munich, School of Medicine) between July 2019 and July 2020. The study was approved by the Ethics Committee of the Technical University of Munich, Munich, Germany (Project Code: 312/19S). All participants provided written consent for research use of their data and the study was performed according to the principles of the Declaration of Helsinki.
CSF samples from patients with cognitive impairment were retrospectively collected from the study center biobank. Patients were diagnosed with AD and FTLD using standard diagnostic criteria, the global clinical dementia rating scale (CDR) (23), and the identification of a pattern of regional hypometabolism on FDG-PET. Patients diagnosed with AD were classified into two groups (amnestic MCI and mild dementia) and patients with FTLD into three groups (bvFTD, svPPA, and nfvPPA), based on standard diagnostic criteria, including FDG-PET (24–27). From the CDR, patients who scored 0.5 were diagnosed with MCI and patients who scored 1.0 were diagnosed with mild dementia due to AD. FDG-PET was used to enhance the diagnostic certainty as suggested by the standard diagnostic criteria for bvFTD, svPPA, and nfvPPA.
CSF Biomarker Analyses
CSF collection and storage were performed according to a site-specific pre-analytical procedure; this was a modified protocol that did not strictly adhere to the Elecsys CSF immunoassay package inserts, as the type of polypropylene tubes used and size of the aliquots (0.5 mL) varied. For each patient, 5−8 mL of CSF were acquired by lumbar puncture, between the L3/L4 or L4/L5 intervertebral space, using atraumatic cannulas and collected in two sterile polypropylene tubes. Immediately after collection, CSF from one polypropylene tube was centrifuged at 2000 x g for 10 minutes at 4 °C (28). Aliquots were frozen and stored at -80 °C at the study center, prior to measurement with the Elecsys CSF immunoassays. CSF from the second polypropylene tube was used to determine routine parameters, including cell count, glucose and lactate measurement, protein differentiation, and CSF/serum ratios of albumin, immunoglobulin G, immunoglobulin A, and immunoglobulin M.
The Elecsys CSF immunoassays are fully automated electrochemiluminescence immunoassays, which utilize monoclonal antibodies in the form of a sandwich test principle. Patient CSF samples were tested using Elecsys β-Amyloid(1−42) CSF, Phospho-Tau (181P) CSF, and Total-Tau CSF immunoassays (29–31), and a robust prototype β-Amyloid(1−40) CSF immunoassay modified for use on the cobas e 411 analyzer (Roche Diagnostics International Ltd, Rotkreuz, Switzerland). The Elecsys β-Amyloid(1–42) CSF, Phospho-Tau (181P) CSF, and Total-Tau CSF immunoassays are Conformité Européen approved in vitro diagnostic devices; however, the β-Amyloid(1−40) CSF immunoassay is available for research use only. CSF biomarker levels were measured for Aβ42, Aβ40, pTau181, and tTau, and biomarker ratio values for pTau181/Aβ42, pTau181/Aβ40, tTau/Aβ42, tTau/Aβ40, Aβ42/Aβ40, pTau181/Aβ42/Aβ40, and tTau/Aβ42/Aβ40 were determined from immunoassay measurements for the individual biomarkers. All data were analyzed using the statistical platform software IBM SPSS Statistics Version 26. No missing data were imputed.
Receiver Operating Characteristic (ROC) Analysis
ROC analysis was performed to assess CSF biomarker and biomarker ratio performance for the differentiation between AD and FTLD; standard diagnostic criteria, together with typical FDG-PET metabolic pattern, were used as the standard-of-truth. Area under the curve (AUC) values, p values (p<0.05 indicates statistical significance), and 95% confidence intervals (CI) were calculated (32). Significance levels and p-values were not adjusted for multiple testing.
Sensitivity and Specificity Analysis
The sensitivity and specificity of Elecsys CSF immunoassays for the differentiation between AD and FTLD were calculated to evaluate the performance of established cut-offs (Aβ42 ≤1000 pg/mL, pTau181/Aβ42 ratio >0.024, and tTau/Aβ42 ratio >0.28; according to the manufacturer package inserts) and optimized cut-offs based on optimization of Youden’s index. The established CSF immunoassay cut-off values were previously determined in a sample set of patients with SCD and early or advanced MCI (BioFINDER study), and validated in a sample set of patients with SCD, early or advanced MCI, and AD (ADNI GO/ADNI 2 studies) (22); the cut-off values were originally developed for a different endpoint (visual amyloid PET result) and using a different pre-analytical protocol than that used in the present study. In the current study, patients with advanced cognitive impairment were included and the endpoint was differential diagnosis of AD versus FTLD.
Post-hoc ROC Analysis
Based on the overlap of clinical syndromes and FDG-PET metabolic pattern, and a comparable CSF biomarker pattern between the AD and nfvPPA subgroups, a post-hoc ROC analysis excluding the nfvPPA subgroup was conducted to explore the effects on AUC. FDG-PET is used in the diagnosis of AD and FTLD; however, it is not considered as a specific biomarker for either disease as reliance on a metabolic pattern can lead to misdiagnosis (33). As for the pre-defined ROC analysis, AUCs, p values (p<0.05 indicates statistical significance), and 95% CIs were calculated. Significance levels and p-values were not adjusted for multiple testing.
Results
Patient Characteristics
A total of 130 patients with cognitive impairment were retrospectively recruited and included in the study. Based on clinical diagnosis and FDG-PET metabolic pattern, 86 patients were diagnosed with AD and 44 were diagnosed with FTLD. Patient characteristics for the AD and FTLD subgroups are shown in Table 1.
Of the 86 patients with AD (male: 45.3%; mean age ± standard deviation [SD; years]: 65.4 ± 8.7), 54 were deemed to be at the stage of MCI (CDR=0.5) and 32 at the stage of mild dementia due to AD (CDR=1.0). Of the 44 patients with FTLD (male: 38.6%; mean age ± SD [years]: 66.7 ± 8.7), 27 patients were diagnosed with bvFTD, 11 with svPPA, and six with nfvPPA.
*AD and FTLD were diagnosed using standard diagnostic criteria, the global CDR, and the identification of a typical metabolic pattern using FDG-PET. Abbreviations: AD = Alzheimer’s disease; bvFTD = behavioral-variant frontotemporal dementia; CDR = clinical dementia rating scale; FDG-PET = [18F] fluorodeoxyglucose positron emission tomography; FTLD = frontotemporal lobar degeneration; MCI = mild cognitive impairment; N = number; nfvPPA = non-fluent variant primary progressive aphasia; SD = standard deviation; svPPA = semantic variant primary progressive aphasia.
AUC-based Performance of CSF Biomarkers/Biomarker Ratios to Differentiate AD and FTLD
AUC-based performance was good for the pTau181/Aβ42 ratio (AUC=0.841; 95% CI: 0.759−0.923), pTau181/Aβ40 ratio (AUC=0.837; 95% CI: 0.754−0.919), Aβ42/Aβ40 ratio (AUC=0.829; 95% CI: 0.746−0.912), tTau/Aβ42 ratio (AUC=0.822; 95% CI: 0.736−0.908), pTau181/Aβ42/Aβ40 (AUC=0.817; 95% CI: 0.734–0.901), and Aβ42 as a single biomarker (AUC=0.812; 95% CI: 0.722−0.902; Table 2; Figure 1). Performance was slightly lower for the tTau/Aβ42/Aβ40 ratio (AUC=0.799; 95% CI: 0.713−0.885), pTau181 alone (AUC=0.793; 95% CI: 0.707−0.880), tTau/Aβ40 ratio (AUC=0.751; 95% CI: 0.657−0.844), and tTau alone (AUC=0.706; 95% CI: 0.613−0.799).
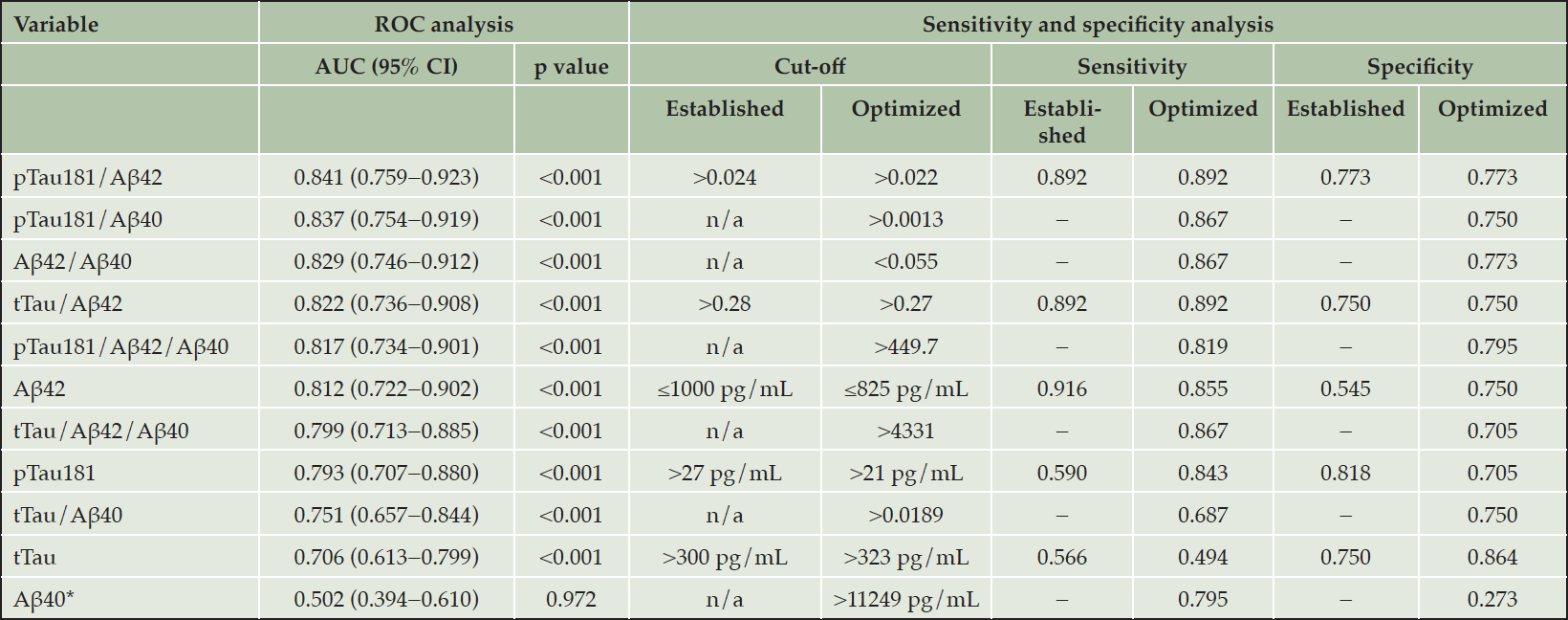
Table 2. ROC and sensitivity/specificity analysis of Elecsys CSF immunoassays for the differentiation between AD and FTLD (n=130)
*Aβ40 was included in the study as a normalization factor. Abbreviations: Aβ = amyloid-beta; AD = Alzheimer’s disease; AUC = area under the curve; CI = confidence interval; CSF = cerebrospinal fluid; FTLD = frontotemporal lobar degeneration; n/a = not applicable; pTau181 = phosphorylated tau 181; ROC = receiver operating characteristic; tTau = total tau.
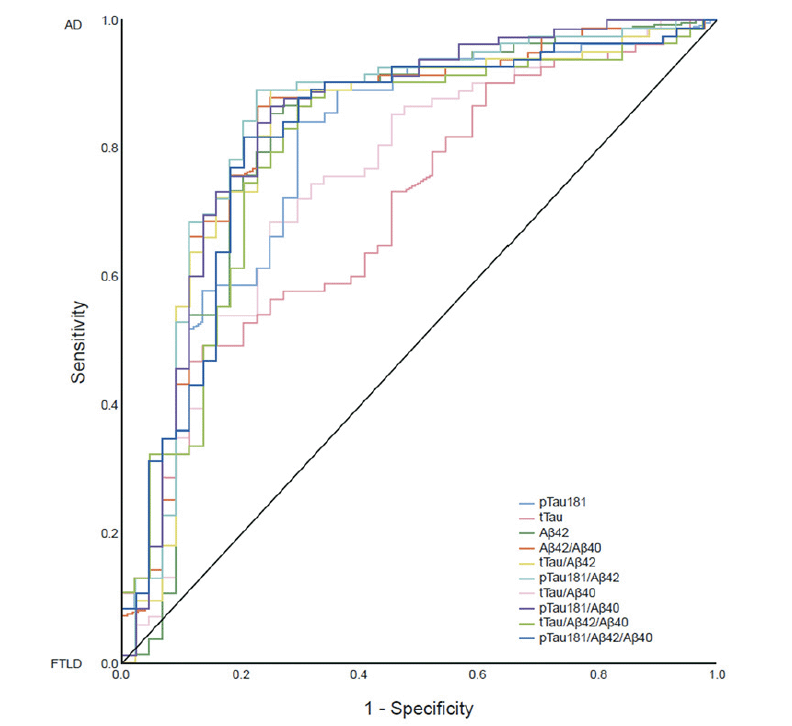
Figure 1. ROC analysis of AUC-based performance of CSF biomarker/biomarker ratios to differentiate AD and FTLD (n=130)
Abbreviations: Aβ = amyloid-beta; AD = Alzheimer’s disease; AUC = area under the curve; CSF = cerebrospinal fluid; FTLD = frontotemporal lobar degeneration; pTau181 = phosphorylated tau 181; ROC = receiver operating characteristic; tTau = total tau.
Sensitivity and Specificity Analysis
The highest qualitative performance was observed with the pTau181/Aβ42 ratio with an established cut-off value of >0.024 and optimized cut-off value of >0.022: sensitivity and specificity values for these cut-offs were 0.892 and 0.773, respectively (Table 2). The sensitivity and specificity values observed for the pTau181/Aβ40 ratio, with an optimized cut-off value of >0.0013, were 0.867 and 0.750, respectively. The sensitivity and specificity values observed for the Aβ42/Aβ40 ratio, with an optimized cut-off value of <0.055, were 0.867 and 0.773, respectively. The sensitivity and specificity values observed for the tTau/Aβ42 ratio, with an established cut-off value of >0.28 and optimized cut-off value of >0.27, were 0.892 and 0.750, respectively. For Aβ42, sensitivity and specificity values were 0.916 and 0.545, respectively, with an established cut-off value of ≤1000 pg/mL, and 0.855 and 0.750, respectively, with an optimized cut-off value of ≤825 pg/mL.
Post-hoc ROC Analysis
In the FTLD nfvPPA subgroup, the CSF biomarker pattern was comparable to the AD biomarker pattern (Figure 2). Excluding the nfvPPA subgroup from the FTLD group resulted in higher AUC values compared with the pre-defined ROC analysis: pTau181/Aβ42 ratio (AUC=0.875; 95% CI: 0.792−0.958); pTau181/Aβ40 ratio (AUC=0.871; 95% CI: 0.788−0.954); Aβ42/40 ratio (AUC=0.867; 95% CI: 0.787−0.946); tTau/Aβ42 ratio (AUC=0.854; 95% CI: 0.768−0.941); and Aβ42 (AUC=0.829; 95% CI: 0.738−0.921; Figure 3). The performances of pTau181 and tTau as single biomarkers were considerably improved by the exclusion of the nfvPPA subgroup (AUC improvement: >5% and 4%, respectively) compared with Aβ42 as a single biomarker (AUC improvement: 1%).
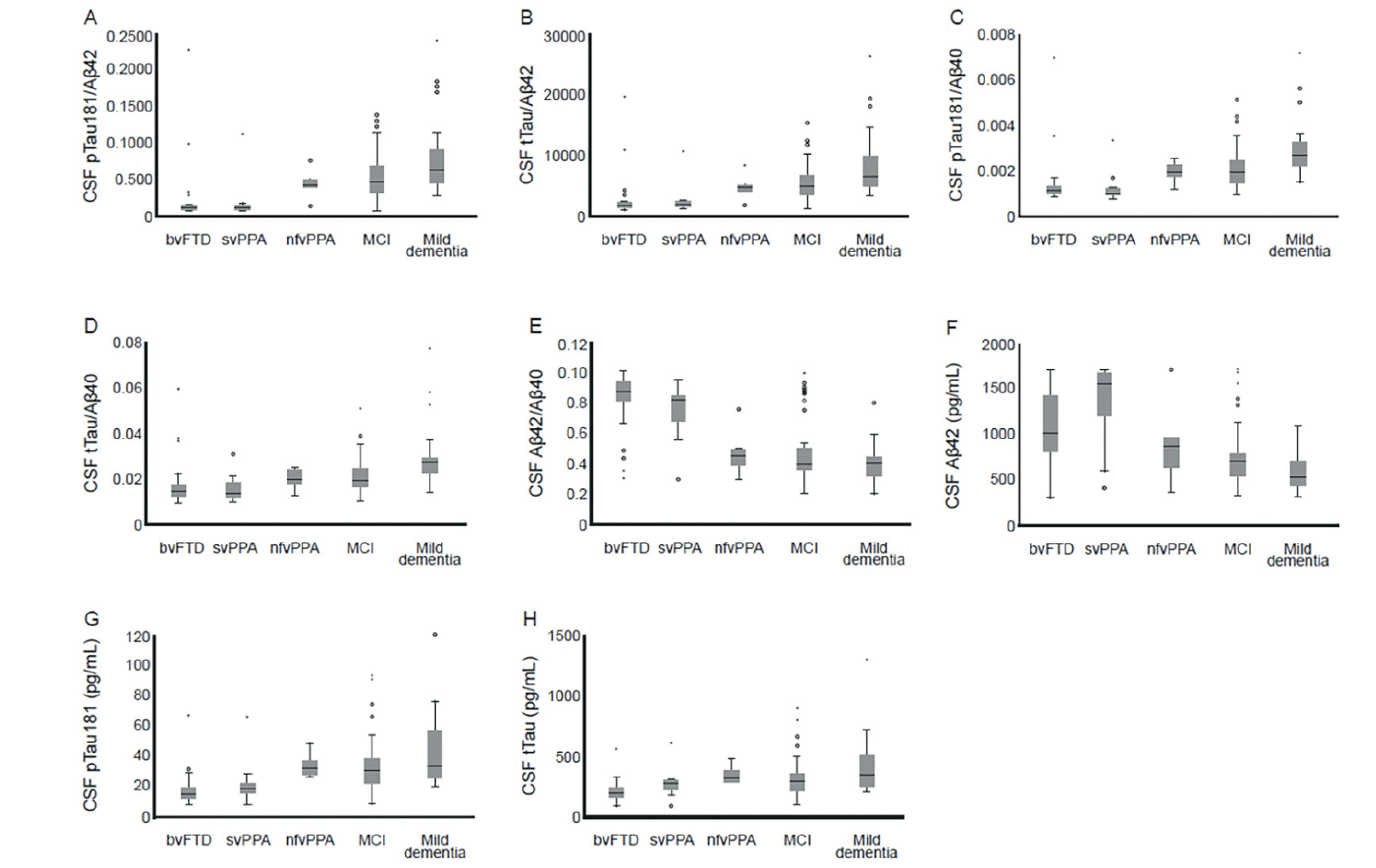
Figure 2. Box plots for A) pTau181/Aβ42, B) tTau/Aβ42, C) pTau181/Aβ40, D) tTau/Aβ40, E) Aβ42/Aβ40, F) Aβ42, G) pTau181, and H) tTau compared with AD and FTLD diagnosis based on FDG-PET (n=130)
* and ◦ symbols represent outliers. Abbreviations: Aβ = amyloid-beta; AD = Alzheimer’s disease; bvFTD = behavioral-variant frontotemporal dementia; CSF = cerebrospinal fluid; FDG-PET = [18F] fluorodeoxyglucose positron emission tomography; FTLD = frontotemporal lobar degeneration; MCI = mild cognitive impairment; nfvPPA = non-fluent variant primary progressive aphasia; pTau181 = phosphorylated tau 181; svPPA = semantic variant primary progressive aphasia; tTau = total tau.
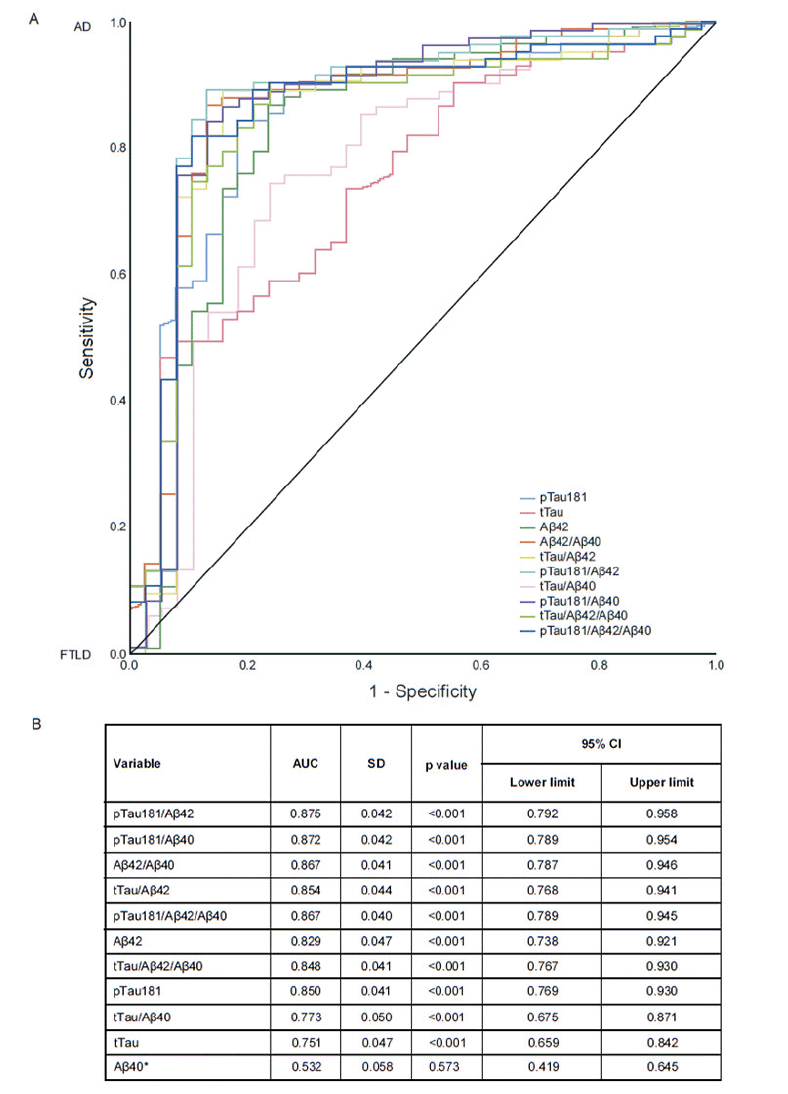
Figure 3. Post-hoc ROC analysis for the differentiation between AD and FTLD, excluding the nfvPPA subgroup (n=124): (A) ROC curves, (B) AUC values
*Aβ40 was included in the study as a normalization factor. Abbreviations: Aβ = amyloid-beta; AD = Alzheimer’s disease; AUC = area under the curve; CI = confidence intervals; FTLD = frontotemporal lobar degeneration; nfvPPA = non-fluent variant primary progressive aphasia; pTau181 = phosphorylated tau 181; ROC = receiver operating characteristic; SD = standard deviation; tTau = total tau.
Discussion
While AD and FTLD are heterogeneous in their clinical presentation and underlying pathology, they often have overlapping features. The ability to accurately differentiate AD from FTLD has important implications for prognostication and in guiding decision-making around treatment and disease management (4), particularly since the approval of aducanumab for the treatment of AD (18). To this end, diagnostic assays measuring CSF biomarkers may be useful tools to increase diagnostic accuracy and aid in further development of disease-modifying treatments (19). The results of this study suggest that Elecsys CSF immunoassays can accurately differentiate AD from FTLD by detecting the underlying amyloid pathology in patients with AD. In a heterogeneous cohort of patients diagnosed with AD or FTLD, based on core clinical criteria and FDG-PET metabolic pattern, Elecsys CSF immunoassays demonstrated good diagnostic accuracy, with biomarker ratios pTau181/Aβ42, pTau181/Aβ40, Aβ42/Aβ40, tTau/Aβ42, and pTau181/Aβ42/Aβ40, and the single biomarker Aβ42, demonstrating the strongest performance out of all biomarkers and biomarker ratios tested. Performance was slightly lower for the tTau/Aβ42/Aβ40 and tTau/Aβ40 ratios, and pTau181 and tTau as single biomarkers. These results add to the body of knowledge around the capabilities of Elecsys CSF immunoassays to aid the diagnosis of cognitive disorders, specifically the differential diagnosis of AD from other types of dementia including FTLD. The sensitivity and specificity analysis showed that the highest qualitative performance for the differentiation between AD and FTLD was observed with the pTau181/Aβ42 ratio, with an established cut-off value of >0.024 and Youden’s optimized cut-off value of >0.022. While additional research is needed to verify the performance of the cut-offs used in this study, our results suggest that Elecsys CSF immunoassays have the potential to support clinicians in diagnosing patients with FTLD by excluding patients with amyloid positivity, which is indicative of underlying AD. It is important to highlight that the cut-offs used in this study were originally developed for a different pre-analytical protocol and an endpoint optimized for concordance with visual amyloid PET; therefore, the cut-off performance observed was expected as AD is associated with amyloid pathology, whereas FTLD is not.
Although FDG-PET is used in the diagnosis of AD and FTLD, it is not considered as a specific biomarker for either disease as reliance on a metabolic pattern can lead to misdiagnosis (33). In this study, it is possible that patients with non-amnestic language presentation of AD were misdiagnosed with nfvPPA due to a known overlap of clinical syndromes and FDG-PET metabolic pattern (26,33). This was indicated by a comparable CSF biomarker pattern between the AD and FTLD nfvPPA subgroups. A post-hoc ROC analysis conducted to exclude the nfvPPA subgroup from the FTLD group resulted in higher AUC values compared with the pre-defined ROC analysis; this suggests that CSF biomarkers can be used as a rule-out test to increase diagnostic certainty. It is also possible that the comparable CSF biomarker pattern observed with the AD and FTLD nfvPPA subgroups was a result of co-morbidity, as many patients diagnosed with nfvPPA are found to have AD pathology (34). In such cases, specific biomarkers to identify the underlying causes of the FTLD spectrum may be needed for an accurate diagnosis of AD versus FTLD. For example, one biomarker that may be useful for the understanding and diagnosis of FTLD is Aβ38, which has demonstrated the ability to differentiate patients with FTLD from a control population (35).
Novel disease-modifying therapies are emerging for neurodegenerative diseases; therefore, it will be important to identify patients with AD versus those with FTLD, so that treatment can be tailored appropriately (4,17,19). Studies have demonstrated that CSF biomarkers have the potential to increase diagnostic accuracy for AD (22). Thus, CSF biomarkers have been incorporated into multiple diagnostic frameworks for AD (36–38). Many existing CSF immunoassays for AD are limited by lot-to-lot and interlaboratory variations, which have hindered the widespread introduction of CSF biomarkers into clinical practice (39). However, the fully automated Elecsys CSF immunoassays used in this study have demonstrated superior interlaboratory variation compared with existing manual CSF assays (CV: 4% versus >15%) (22).
Current diagnostic research criteria for FTLD do not recommend use of CSF biomarkers (25,27). Differential diagnosis between AD and FTLD usually requires patients to undergo a plethora of neuropsychological tests, neurological assessments, neuroimaging, and laboratory tests, which can be onerous for patients and relies on access to specialists (2,8). Thus, improving diagnostic accuracy would benefit the patient. Studies have demonstrated that amyloid PET can be used as a tool for differential diagnosis of AD and FTLD, based on characterization of underlying AD pathology, which in turn improves diagnosis, prognosis, and selection of patients for clinical trials (40,41). However, amyloid PET scans are expensive and may not be universally available, whereas using CSF analysis would be cheaper, patients would not be exposed to radioactivity, and CSF assessment could take place at non-specialized centers, which would increase patient accessibility to accurate diagnosis (22). The results of this study show that CSF biomarkers can differentiate AD from FTLD by identifying underlying amyloid pathology and can be used as a rule-out test to increase diagnostic certainty. Therefore, CSF biomarkers could potentially be an alternative to amyloid PET for the differential diagnosis of AD and FTLD.
While the sample size of this study was limited (n=130), the patients included were highly characterized. Our study utilized the well-established Elecsys CSF immunoassays for measurement of CSF biomarkers; however, the pre-defined cut-off values were originally developed using a different pre-analytical protocol. Nevertheless, the optimized cut-off values calculated by Youden’s index equated to the established cut-off values for pTau181/Aβ42 and tTau/Aβ42, which underscores the robustness and practicality of both cut-off values in the clinical setting. Our study deviates from previously published studies: patients were diagnosed with AD and FTLD using standard diagnostic criteria, plus confirmation by a non-CSF biomarker (FDG-PET), rather than clinical diagnosis alone. A wider range of biomarkers and biomarker ratios were evaluated, including the pTau181/Aβ40 ratio; the analyses were run on the fully automated Elecsys CSF immunoassays and allowed for the identification of different clinical subtypes of FTLD (namely, bvFTD, svPPA, and nfvPPA). Limitations of this study include the retrospective and post-hoc nature of the analyses. Future studies to confirm these findings should be conducted prospectively, in a larger sample size, and in an independent cohort.
In conclusion, our findings suggest that Elecsys CSF immunoassays demonstrate good diagnostic accuracy in differentiating patients with AD from those with FTLD. The Elecsys CSF immunoassays have the potential to support clinical decision making, i.e. in diagnosing patients with FTLD by excluding patients with amyloid positivity, which is indicative of underlying AD.
Acknowledgments: Third-party medical writing assistance, under the direction of the authors, was provided by Sophie Lavelle, MSc, of Ashfield MedComms (Macclesfield, UK), an Ashfield Health company, and was funded by Roche Diagnostics International Ltd (Rotkreuz, Switzerland). ELECSYS, COBAS, and COBAS E are trademarks of Roche.
Funding: This study was funded by Roche Diagnostics International Ltd (Rotkreuz, Switzerland). Open Access funding enabled and organized by Projekt DEAL.
Disclosures: OG reports receiving grants from Actelion and payment from Julius Clinical/Toyama for prescreening activities. IY reports receiving grants or contracts from the Federal Ministry of Education and Research Germany (BMBF); consulting fees from Blue Earth Diagnostics and ABX-CRO; payment or honoraria for lectures, presentations, speakers bureaus, manuscript writing or educational events from Piramal; support for attending meetings and/or travel from the Society for Nuclear Medicine and Molecular Imaging; and leadership or fiduciary role in the Society for Nuclear Medicine and Molecular Imaging (Board of Directors, Brain Imaging Council). JPW is an employee of Roche Diagnostics GmbH and holds shares in F. Hoffmann-La Roche Ltd. EM is an employee of Roche Diagnostics GmbH. MS is an employee of Roche Diagnostics International Ltd. TG reports receiving consulting fees from Abbvie, Anavex, Biogen, Bracket, Eli Lilly, Functional Neuromodulation, Grifols, IQVIA/Quintiles, Novartis, Novo Nordisk, Noselab, NuiCare, Roche Pharma, Toyama, and Vivoryon; payment or honoraria for lectures, presentations, speakers bureaus, manuscript writing or educational events from Actelion, B. Braun, Biogen, Eli Lilly, Life Molecular Imaging, Novartis, Parexel, and Roche Pharma; and support for attending meetings and/or travel from Biogen. The remaining authors declare no known competing financial interests or personal relationships that could have appeared to influence the work reported in this manuscript.
Ethical standards: The study was approved by the Ethics Committee of the Technical University of Munich, Munich, Germany (Project Code: 312/19S). All participants provided written consent for research use of their data and the study was performed according to the principles of the Declaration of Helsinki.
Open Access: This article is distributed under the terms of the Creative Commons Attribution 4.0 International License (http://creativecommons.org/licenses/by/4.0/), which permits use, duplication, adaptation, distribution and reproduction in any medium or format, as long as you give appropriate credit to the original author(s) and the source, provide a link to the Creative Commons license and indicate if changes were made.
References
1. World Health Organization. Dementia. 2020. https://www.who.int/news-room/fact-sheets/detail/dementia. Accessed 13 August 2021.
2. Rabinovici GD, Miller BL. Frontotemporal lobar degeneration: Epidemiology, pathophysiology, diagnosis and management. CNS Drugs 2010;24:375−398. https://doi.org/10.2165/11533100-000000000-00000.
3. Young JJ, Lavakumar M, Tampi D, Balachandran S, Tampi RR. Frontotemporal dementia: Latest evidence and clinical implications. Ther Adv Psychopharmacol 2018;8:33−48. https://doi.org/10.1177/2045125317739818.
4. Erkkinen MG, Kim MO, Geschwind MD. Clinical neurology and epidemiology of the major neurodegenerative diseases. Cold Spring Harb Perspect Biol 2018;10:a033118. https://doi.org/10.1101/cshperspect.a033118.
5. DeTure MA, Dickson DW. The neuropathological diagnosis of Alzheimer’s disease. Mol Neurodegeneration 2019;14:32. https://doi.org/10.1186/s13024-019-0333-5.
6. Schultz C, Del Tredici K, Braak H. Neuropathology of Alzheimer’s disease. In: Richter R.W., Richter B.Z. (eds) Alzheimer’s Disease. Current Clinical Neurology. 2004. Humana Press, Totowa, NJ, pp 21–31. https://doi.org/10.1007/978-1-59259-661-4_2.
7. Wang X, Shen Y, Chen W. Progress in frontotemporal dementia research. Am J Alzheimers Dis Other Demen 2013;28:15–23. https://doi.org/10.1177/1533317512467681.
8. Bang J, Spina S, Miller BL. Frontotemporal dementia. Lancet 2015;386:1672−1682. https://doi.org/10.1016/S0140-6736(15)00461-4.
9. Mackenzie IR, Neumann M, Bigio EH, et al. Nomenclature and nosology for neuropathologic subtypes of frontotemporal lobar degeneration: An update. Acta Neuropathol 2010;119:1−4. https://doi.org/10.1007/s00401-009-0612-2.
10. Rohrer JD, Lashley T, Schott JM, et al. Clinical and neuroanatomical signatures of tissue pathology in frontotemporal lobar degeneration. Brain 2011;134:2565−2581. https://doi.org/10.1093/brain/awr198.
11. Geser F, Brandmeir NJ, Kwong LK, et al. Evidence of multisystem disorder in whole-brain map of pathological TDP-43 in amyotrophic lateral sclerosis. Arch Neurol 2008;65:636−641. https://doi.org/10.1001/archneur.65.5.636.
12. Gossye H, Van Broeckhoven C, Engelborghs S. The use of biomarkers and genetic screening to diagnose frontotemporal dementia: Evidence and clinical implications. Front Neurosci 2019;13:757. https://doi.org/10.3389/fnins.2019.00757.
13. Rabinovici GD, Rosen HJ, Alkalay A, et al. Amyloid vs FDG-PET in the differential diagnosis of AD and FTLD. Neurology 2011;77:2034−2042. https://doi.org/10.1212/WNL.0b013e31823b9c5e.
14. Boxer AL, Gold M, Feldman H, et al. New directions in clinical trials for frontotemporal lobar degeneration: Methods and outcome measures. Alzheimer’s Dement 2020;16:131–143. https://doi.org/10.1016/j.jalz.2019.06.4956.
15. Yiannopoulou KG, Papageorgiou SG. Current and future treatments in Alzheimer disease: An update. J Cent Nerv Syst Dis 2020;12:1−12. https://doi.org/10.1177/1179573520907397.
16. Liscic RM, Alberici A, Cairns NJ, Romano M, Buratti E. From basic research to the clinic: Innovative therapies for ALS and FTD in the pipeline. Mol Neurodegener 2020;15:31. https://doi.org/10.1186/s13024-020-00373-9.
17. Cummings J, Lee G, Ritter A, Sabbagh M, Zhong K. Alzheimer’s disease drug development pipeline: 2020. Alzheimers Dement (N Y) 2020:16;6:e12050. https://doi.org/ 10.1002/trc2.12050.
18. FDA. FDA news release: FDA grants accelerated approval for Alzheimer’s drug (7 June 2021). https://www.fda.gov/news-events/press-announcements/fda-grants-accelerated-approval-alzheimers-drug. Accessed 13 August 2021.
19. Irwin DJ, Trojanowski JQ, Grossman M. Cerebrospinal fluid biomarkers for differentiation of frontotemporal lobar degeneration from Alzheimer’s disease. Front Aging Neurosci 2013;5:6. https://doi.org/10.3389/fnagi.2013.00006.
20. Hansson O, Zetterberg H, Buchhave P, Londos E, Blennow K, Minthon L. Association between CSF biomarkers and incipient Alzheimer’s disease in patients with mild cognitive impairment: A follow-up study. Lancet Neurol 2006;5:228–234. https://doi.org/10.1016/S1474-4422(06)70355-6.
21. Mattsson N, Zetterberg H, Hansson O, et al. CSF biomarkers and incipient Alzheimer disease in patients with mild cognitive impairment. JAMA 2009;302:385–393. https://doi.org/10.1001/jama.2009.1064.
22. Hansson O, Seibyl J, Stomrud E, et al; Swedish BioFINDER study group; Alzheimer’s Disease Neuroimaging Initiative. CSF biomarkers of Alzheimer’s disease concord with amyloid-β PET and predict clinical progression: A study of fully automated immunoassays in BioFINDER and ADNI cohorts. Alzheimers Dement 2018;14:1470–1481. https://doi.org/10.1016/j.jalz.2018.01.010.
23. Morris JC, Ernesto C, Schafer K, et al. Clinical dementia rating training and reliability in multicenter studies: The Alzheimer’s Disease Cooperative Study experience. Neurology 1997;48:1508−10. https://doi.org/10.1212/wnl.48.6.1508.
24. Albert MS, DeKosky ST, Dickson D, et al. The diagnosis of mild cognitive impairment due to Alzheimer’s disease: Recommendations from the National Institute on Aging-Alzheimer’s Association workgroups on diagnostic guidelines for Alzheimer’s disease. Alzheimers Dement 2011;7:270−279. https://doi.org/10.1016/j.jalz.2011.03.008.
25. Gorno-Tempini ML, Hillis AE, Weintraub S, et al. Classification of primary progressive aphasia and its variants. Neurology 2011;76:1006−1014. https://doi.org/10.1212/WNL.0b013e31821103e6.
26. McKhann GM, Knopman DS, Chertkow H, et al. The diagnosis of dementia due to Alzheimer’s disease: Recommendations from the National Institute on Aging-Alzheimer’s Association workgroups on diagnostic guidelines for Alzheimer’s disease. Alzheimers Dement 2011;7:263–269. https://doi.org/10.1016/j.jalz.2011.03.005.
27. Rascovsky K, Hodges JR, Knopman D, et al. Sensitivity of revised diagnostic criteria for the behavioural variant of frontotemporal dementia. Brain 2011;134:2456−2477. https://doi.org/10.1093/brain/awr179
28. Teunissen CE, Petzold A, Bennett JL, et al. A consensus protocol for the standardization of cerebrospinal fluid collection and biobanking. Neurology 2009;73:1914−1922. https://doi.org/10.1212/WNL.0b013e3181c47cc2
29. Roche Diagnostics. Elecsys Phospho-Tau (181P) CSF. 2018. https://pim-eservices.roche.com/eLD/api/downloads/1b990a9b-e639-e811-f092-00215a9b3428?countryIsoCode=gb. Accessed 13 August 2021.
30. Roche Diagnostics. Elecsys Total-Tau CSF Method Sheet. 2018. https://pim-eservices.roche.com/eLD/api/downloads/b76c246c-e539-e811-f092-00215a9b3428?countryIsoCode=gb. Accessed 13 August 2021.
31. Roche Diagnostics. Elecsys β-Amyloid (1-42) CSF Method Sheet. 2020. https://pim-eservices.roche.com/eLD/api/downloads/2a206d32-5120-ea11-fa90-005056a772fd?countryIsoCode=gb. Accessed 13 August 2021.
32. Hanley JA, McNeil BJ. A method of comparing the areas under receiver operating characteristic curves derived from the same cases. Radiology 1983;148;839–843. https://doi.org/10.1148/radiology.148.3.6878708.
33. Nobili F, Arbizu J, Bouwman F, et al. European Association of Nuclear Medicine and European Academy of Neurology recommendations for the use of brain 18F fluorodeoxyglucose positron emission tomography in neurodegenerative cognitive impairment and dementia: Delphi consensus. Eur J Neurol 2018;25:1201–1217. https://doi.org/10.1111/ene.13728.
34. Gorno-Tempini ML, Pressman P. Introduction to primary progressive aphasia. In: Hickok G and Small SL (eds) Neurobiology of language. 2016. Elsevier, Amsterdam. pp 935–52. https://doi.org/10.1016/B978-0-12-407794-2.00075-4.
35. Gabelle A, Roche S, Gény C, et al. Decreased sAβPPβ, Aβ38, and Aβ40 cerebrospinal fluid levels in frontotemporal dementia. J Alzheimers Dis 2011;26:553−563. https://doi.org/10.3233/JAD-2011-110515.
36. Engelborghs S, Niemantsverdriet E, Struyfs H, et al. Consensus guidelines for lumbar puncture in patients with neurological diseases. Alzheimers Dement (Amst) 2017:8;111–126. https://doi.org/10.1016/j.dadm.2017.04.007.
37. Jack CR Jr, Bennett DA, Blennow K, et al. A/T/N: An unbiased descriptive classification scheme for Alzheimer disease biomarkers. Neurology 2016;87:539−547. https://doi.org/10.1212/WNL.0000000000002923.
38. Jack CR Jr, Bennett DA, Blennow K, Cet al. NIA-AA Research Framework: Toward a biological definition of Alzheimer’s disease. Alzheimers Dement 2018;14:535−562. https://doi.org/10.1016/j.jalz.2018.02.018.
39. Mattsson N, Andreasson U, Persson S, et al. The Alzheimer’s Association external quality control program for cerebrospinal fluid biomarkers. Alzheimers Dement 2011;7:386−395. https://doi.org/10.1016/j.jalz.2011.05.2243.
40. Drzezga A, Grimmer T, Henriksen G, et al. Imaging of amyloid plaques and cerebral glucose metabolism in semantic dementia and Alzheimer’s disease. Neuroimage 2008;39:619–633. https://doi.org/10.1016/j.neuroimage.2007.09.020.
41. Jansen WJ, Ossenkoppele R, Knol DL, et al; Amyloid Biomarker Study Group. Prevalence of cerebral amyloid pathology in persons without dementia: a meta-analysis. JAMA 2015;313:1924–1938. https://doi.org/10.1001/jama.2015.4668.