E. Chipi*, G. Bellomo*, N. Salvadori, C. Montanucci, L. Gaetani, F. Paolini Paoletti, L. Parnetti
Center for Memory Disturbances, Lab of Clinical Neurochemistry, Section of Neurology, Department of Medicine and Surgery, University of Perugia, Italy; *equal contribution
Corresponding Author: Prof. Lucilla Parnetti, Center for Memory Disturbances, Lab of Clinical Neurochemistry, Section of Neurology, Department of Medicine and Surgery, University of Perugia, Italy, E-mail address: lucilla.parnetti@unipg.it, Telephone number: +390755783545, Fax number: +390755784229
J Prev Alz Dis 2023;3(10):523-529
Published online April 1, 2023, http://dx.doi.org/10.14283/jpad.2023.33
Abstract
BACKGROUND: In the perspective of novel treatments with disease-modifying drugs, a timely diagnosis of Alzheimer’s’ disease (AD) at preclinical phase represents a major issue. To this purpose, in clinical setting, there is the need to detect the earliest cognitive symptoms not yet fulfilling Mild Cognitive Impairment criteria, in order to proceed to biomarker assessment for diagnostic definition. In terms of cognitive performance, Subjective Cognitive Decline (SCD) is still a controversial entity, due to the difficulty of reliably measuring subtle deficits.
OBJECTIVE: To evaluate the possibility to predict the presence of AD-like CSF pattern in SCD individuals, according to their neuropsychological performance assessed by means of both traditional and computerized measures.
DESIGN: Retrospective study.
SETTING: Clinical setting (Centre for Memory Disturbances, Section of Neurology, University Hospital of Perugia, Italy).
PARTICIPANTS: 74 consecutive SCD subjects who underwent an in-depth (paper-pencil and computerized) neuropsychological assessment and CSF analysis for AD biomarkers (Aβ42/Aβ40 ratio, phospho-tau, total tau).
MEASUREMENTS: Neuropsychological assessment was composed of traditional tests assessing five cognitive domains (verbal memory, attention, executive functions, language, visuo-spatial abilities) and computerized tasks from CAmbridge Neuropsychological Test Automated Battery (CANTAB) (Pattern Recognition Memory, Paired Associates Learning and Spatial Working Memory). According to their performance at traditional tests, SCD individuals were categorized into cognitively normal (CN) and subtle impaired (SI); with respect to CANTAB, they were defined as CANTAB- in presence of normal performance, and CANTAB+ in presence of at least one pathological score. The subgroup with completely normal performance was defined as CN/CANTAB-, and the subgroup with impairment in both measures as SI/CANTAB+. Differences in prevalence of A/T/N profile according to cognitive profiles were assessed by Fisher’s exact text for count data.
RESULTS: None of CN/CANTAB- subjects showed A+/T+ status. SI/CANTAB+ subjects showed a significantly high prevalence of A+/T+ profile (14/35, 40%, p=0.03 vs CN/CANTAB-).
CONCLUSION: The neuropsychological profile may be of help in identifying SCD subjects requiring biomarker assessment. If confirmed in larger cohorts, the combination of traditional and computerized tests (namely, CANTAB) might represent a feasible strategy in clinical setting for carrying out biomarker assessment in individuals before the MCI stage. Detection of AD in these subjects would give them the highest chances to halt disease progression by means of disease modifying treatments.
Key words: Alzheimer’s Disease, subjective cognitive decline, neuropsychological assessment, CANTAB, cerebrospinal fluid biomarkers.
Introduction
Alzheimer’s disease (AD) pathology begins several years before the onset of clinical symptoms (1–5). The possibility to detect AD in vivo by means of pathophysiological biomarkers allows the diagnosis even before the prodromal stage, at the preclinical phase (6–9). A timely diagnosis of AD represents a major issue in view of the availability of novel treatments with disease-modifying drugs that may have the greatest efficacy when targeting individuals at the earliest stages of the disease.
Subjective Cognitive Decline (SCD) refers to a self-experienced decline in cognitive functioning with no impairment in daily functioning (10). Although it is still controversial if this condition might represent a preclinical stage of AD (8, 11) several studies report that SCD subjects showing PET or CSF amyloid positivity have an increased risk of cognitive decline (12–18) thus representing preclinical AD (19).
In view of the availability of effective disease modifying treatments, SCD individuals who seek for an objective diagnostic verification about the cognitive impairment they perceive, represent the ideal population deserving biomarkers assessment. In clinical setting, the major difficulty is represented by the lack of a clear definition of subtle cognitive impairment, i.e., the objective evidence of pathological scores not reaching MCI criteria. The main reasons for that are the large variability of neuropsychological measures used worldwide, and the lack of standardized procedures devoted to this specific aim. Some studies proposed to use “milder” cut-off as compared to MCI (i.e., impairment defined as more than 1 time but less than 1.5 times the normative standard deviation) (20, 21). Others focused on the number of altered tests in order to increase reliability (22). Another possible approach might be represented by the combination of a traditional domain-oriented assessment with a multi-componential approach, where the latter, represented by computerized tools, allows for the simultaneous evaluation of multiple sub-processes underlying cognitive functioning.
Cambridge Neuropsychological Test Automated Battery (CANTAB) is a computerized neuropsychological test battery based on touch-screen presentation of visual stimuli (23, 24). It has been used in a wide range of neurologic clinical and research settings, including studies with healthy elderly subjects (25). CANTAB has been developed on the basis of neuroanatomic-functional models of cognitive functioning derived from animal studies, then translated in human subjects with the assumption of higher sensitivity in detecting cognitive dysfunction due to multi-componential structure of its tasks. In fact, neuroimaging studies supported the hypothesis that CANTAB tasks allow the simultaneous assessment of multiple sub-processes underlying cognitive functioning in specific domains, relying on the activation of distinct key neural structure or networks (26). Thus, CANTAB could be suitable for assessing subtle cognitive deficits as compared with traditional domain-specific approach (27, 28). Of interest, CANTAB allows for the assessment of sub-components of cognitive functioning (namely attentional-executive processes, speed of information processing, working memory and associative learning) likely involved since the earliest AD phases (20, 29–31).
To this purpose, we retrospectively analysed a consecutive series of SCD subjects referring to our Centre for a comprehensive diagnostic assessment, including extensive neuropsychological evaluation and CSF biomarker assessment. Neuropsychological assessment included both traditional batteries, evaluating five cognitive domains (verbal memory, attention, executive functions, language, visuo-spatial abilities) and CANTAB evaluation assessing visual memory (Pattern Recognition Memory, PRM), working memory (Spatial Working Memory, SWM) and associative learning (Paired Associates Learning, PAL).
According to the performance at traditional battery, subjects showing no deficit were defined as cognitively normal (CN), as opposite to those showing subtle impairment (SI). Normal performance at CANTAB was defined as CANTAB-, while presence of impaired scores was defined as CANTAB+. Therefore, we created four subgroups: those who obtained normal performance at both measures (CN/CANTAB-), those with subtle cognitive deficits in both measures (SI/CANTAB+) and those who presented an “intermediate” cognitive profile (CN/CANTAB+ or SI/CANTAB-).
The aim of our study was to evaluate the association between CSF patterns and neuropsychological profiles.
Methods
Participants
A consecutive series of subjects who referred for subjective cognitive decline to Centre for Memory Disturbances in the period 2014-2022, having available traditional and computerized neuropsychological assessment, and CSF biomarkers, were retrospectively enrolled. The definition of subjective cognitive decline met SCD criteria (10). Since 2008, in our Centre CSF collection for early diagnosis of Alzheimer’s disease is performed, including cognitively unimpaired subjects with subjective memory complaints who express the will to know the possible cause of their symptoms (approved by Ethics Committee Umbria, 9 October 2008, protocol N.19369/08/AV, registry N. 1287/08).
Neuropsychological assessments
Traditional paper-pencil battery: it includes Mini Mental State Examination, MMSE (32), and HIV-Dementia Rating Scale, HDS (33), as screening tests for the assessment of global cognitive functioning, and a battery assessing five cognitive domains: attention [Trail Making Test-A (34); Digit Span forward (35)], executive functions [Trail Making Test-B (34); Digit Span backward (35)], verbal memory [Ray Auditory Verbal Learning Test, immediate and delayed recall (36); Story recall (37)], visuo-spatial abilities [Copy of drawing with and without landmarks from Mental Deterioration Battery (36)] and language [letter fluency (36) and phonemic fluency (38)]. Each neuropsychological score was converted to an Equivalent Score (ES) using published normative data for Italian cohorts. This ES correction method was developed by Capitani and colleagues (39) in an Italian multicentre study aimed at overcoming the standardization of neuropsychological scores after adjustment for age and education. This methodology makes it possible to establish the test subject’s position relative to a normative cohort, taking into account the influence of demographic variables. Specifically, the raw score is corrected and then converted into the corresponding ES on an ordinal scale ranging from 0 to 4 that maps percentile ranks of adjusted scores. ES= 0 corresponds to an insufficient score, below the 5th percentile; ES= 1 indicates a poor performance, between the 5th and 20th percentile; ES= 2-3 falls between the 20th and 50th percentile, showing a moderate performance; ES= 4 refers to a good performance, above the 50th percentile. Accordingly, in our study, subjects obtaining ES≥2 in all tests were defined as cognitively normal, CN; subtle impairment (SI) was defined in presence of ES=1 in at least one test.
CANTAB® (40): according to the available published data related to the sensitivity in detection of early AD, three tasks were proposed: Pattern Recognition Memory (PRM), Paired Associates Learning (PAL) and Spatial Working Memory (SWM). PRM is a measure of visual recognition memory in a 2-choice forced paradigm, and it has been considered particularly sensitive to temporal lobe dysfunction (41) and capable of distinguishing between MCI and normal controls (25). The analysed measure was “PRM-Immediate” That includes percentage of correct trials as outcome measure. PAL assesses visual memory and associative learning. It has been suggested that it might be used for the screening of AD-related memory impairment (26–28, 42). The outcome measure used is “PAL-total error” that evaluates the number of times the subject chose the incorrect box for a stimulus. SWM measures the ability to retain visuo-spatial information and manipulate it in working memory, which is impaired in early AD (20). It is considered a sensitive tool for discriminating MCI from normal ageing in combination with other measures of memory, attention and executive function (43). Outcome measure used is “SWM-between errors”, assessing the total number of times the subject returns on a box in which a token has previously been found.
Conventionally, insufficient performance at CANTAB is defined as ≥ 1.5 SD below normative means (44). Here, subtle impaired performance at CANTAB (CANTAB+) was defined as scores ≥1 SD below normative means in at least one task. We adopted a more inclusive criterion in order to include even subtle/minimal deficits at CANTAB.
CSF biomarker assessment
LP was performed according to international guidelines (45). Following a standardized procedure, 10–12 mL of CSF was collected in sterile polypropylene tubes and centrifuged at room temperature for 10 min (2000× g). Aliquots (0.5 mL) were frozen at −80 ◦C. In the CSF samples collected, Aβ40, Aβ42, total tau (t-tau) and tau phosphorylated at threonine 181 (p-tau) levels were measured by using Lumipulse G600-II (Lumipulse) β-Amyloid 1–40, Lumipulse β-Amyloid 1–42, Lumipulse Total Tau and Lumipulse p-Tau 181 assays (Fujirebio Europe, Gent, Belgium). Different production lots numbers were used to generate data for all Lumipulse assays. Standard quality controls and internal quality controls were assayed in each run. All analyses were performed by board-certified laboratory technicians, blinded to clinical data.
By applying the A/T/(N) classification scheme (7), A+/T+ profile was considered as CSF AD-like profile. According to the cut-off values calculated in our lab (46), “A+” corresponds to a CSF Aβ42/40 ratio<0.072, and “T+” corresponds to a CSF phospho-tau >50 pg/mL. Low CSF Aβ42/Aβ40 ratio and normal value of p-tau was defined as isolated amyloidosis (A+/T-). Normal CSF Aβ42/Aβ40 ratio and high p-tau was defined as non-AD pattern (A-/T+). Normal values of both CSF Aβ42/Aβ40 ratio and p-tau were defined as non-compatible with AD.
Statistical methodology
The data analysis was performed by using OriginPro 9 and R v4.2. Demographical and clinical features were summarized by using the mean ± standard deviation (SD). Multiple groups comparisons for continuous variables were performed by Kruskal-Wallis test. Dunn’s post-hoc test with Benjamini and Hochberg correction was applied to compare neuropsychological performances between groups. The statistical significance of differences in categorical variables (e.g., sex) among groups was assessed by chi-square test. The statistical significance of different prevalences among groups (i.e., prevalence of A+/T+ and A-T- CSF profiles in groups stratified according to cognitive scores) was assessed by Fisher’s exact text for count data. To understand which test was more efficient in discriminating A+/T+ and A-/T- CSF profiles, Least absolute shrinkage and selection operator (LASSO), a feature selection technique used for machine learning predictive modelling, was used (47). Penalty parameters λ corresponding to minimum binomial deviance (λmin) and to the minimum plus 1 standard error (λ1SE) were calculated by cross-validation. The glmnet R package was used for these purposes (48). Logistic regression (glm package) was then applied to further assess the significance of the association between LASSO-selected tests and A+/T+ status by assuming age, gender and education as covariates. Receiver Operating Characteristic (ROC) curve analysis was also performed by using the R package pROC (49) (see Supplementary material). The area under the curve (AUC) and AUC confidence intervals were calculated by using 2000 bootstrap replicates. A significance level of 0.05 was assumed in all the analyses.
Results
The details of SCD demographic details, A/T/N profiles and subcategories according to the neuropsychological performance (CN, SI, CANTAB+, CANTAB-, CN/CANTAB-, SI/CANTAB-; CN/CANTAB+; SI/CANTAB+) are reported in Table 1. With respect to CSF profile, approx. half of SCD subjects (35/74, 47%) were A-/T-, as opposite to one third of them (22/74, 30%) showing a CSF AD like profile (A+/T+). Out of 74 SCD individuals, only 7 (9%) showed a completely normal neuropsychological performance (CN/CANTAB-), while 35 (47%) showed some deficit in both measures (SI/CANTAB+). Raw scores (mean and SD) and number of impaired scores on traditional tests and CANTAB tasks are reported in Table 2.
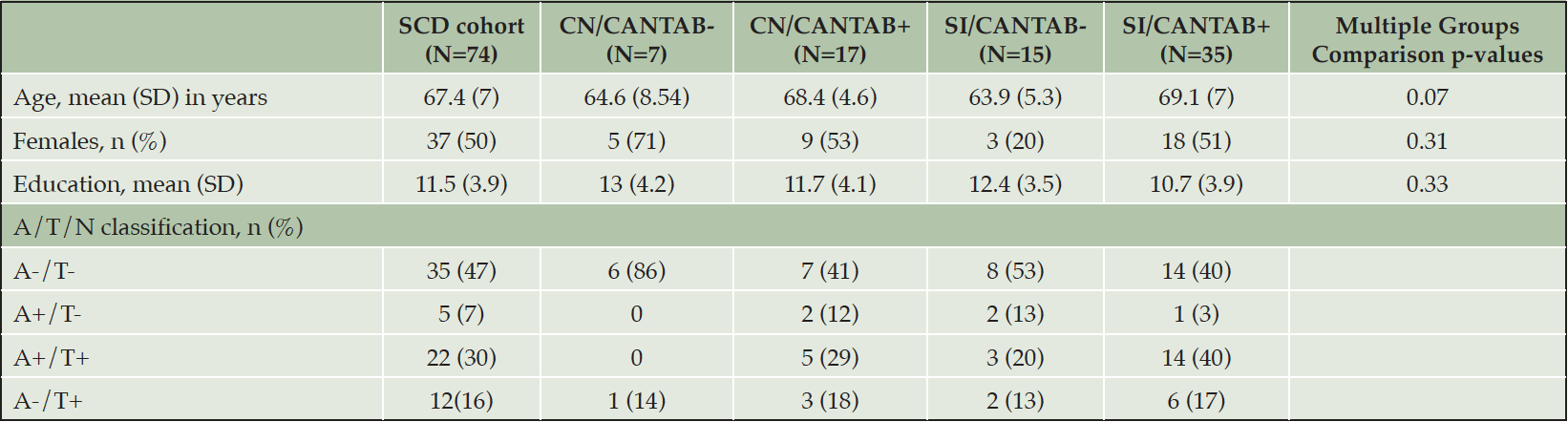
Table 1. Demographic and clinical characteristics of the SCD cohort and the 4 subgroups. Multiple groups comparison was performed by Kruskal-Wallis test for age and education. Multiple groups comparison on count data (gender) was performed by chi-square test
CANTAB: Cambridge Neuropsychological Test Automated Battery; CN: cognitively normal; SI: subtle impairment; SCD: subjective cognitive decline
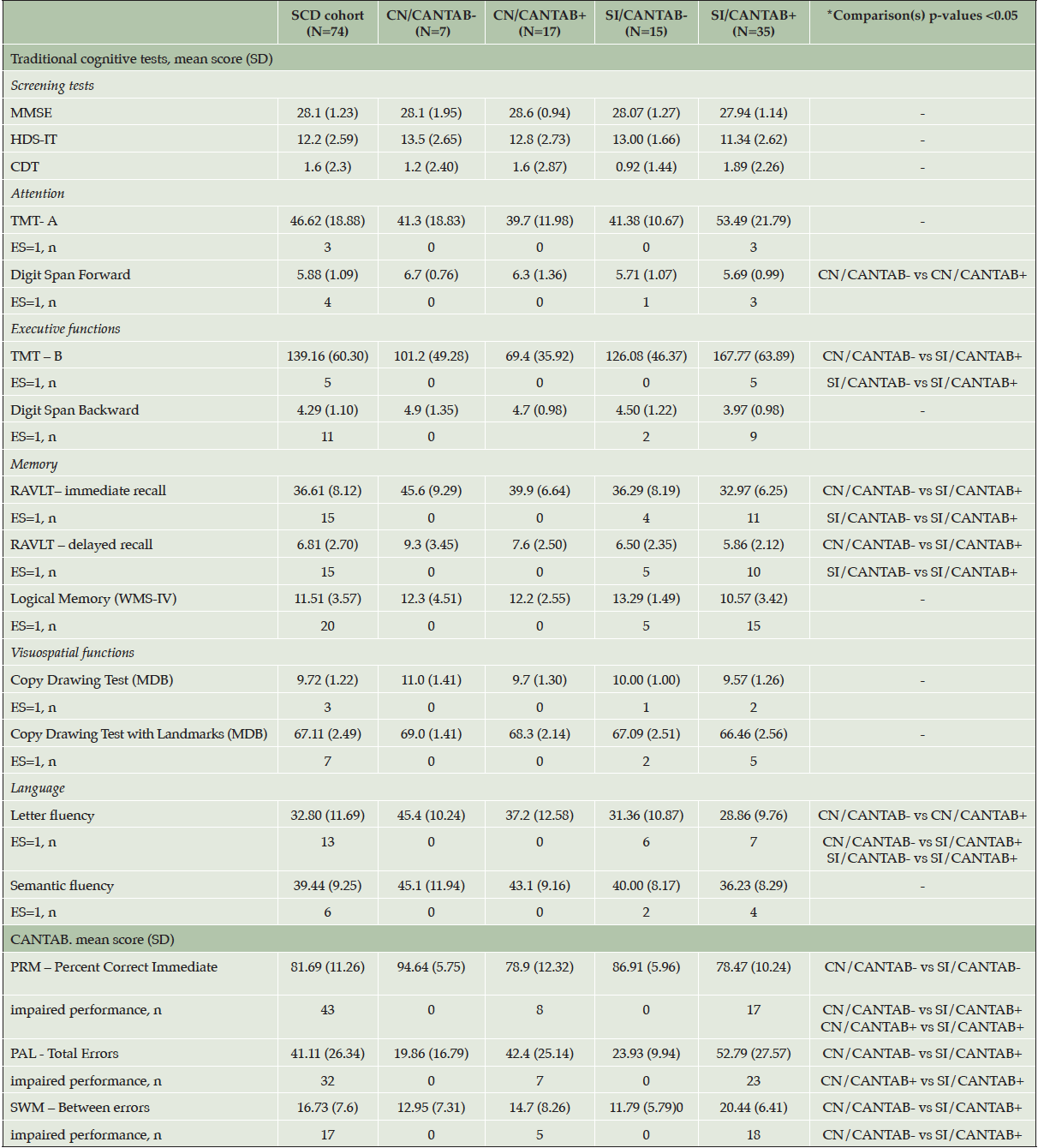
Table 2. Traditional tests and CANTAB scores in the SCD cohort and in the subgroups. Multiple groups comparison was performed by Kruskal-Wallis test with Dunn’s post-hoc test with Benjamini and Hochberg correction
CANTAB: Cambridge Neuropsychological Test Automated Battery; CDT: Clock Drawing Test; CN: cognitively normal; ES: Equivalent Scores (Capitani et al. 1987); HDS-IT: HIV Dementia Scale Italian Version; MDB: Mental Deterioration Battery; MMSE: Mini mental State Examination; PAL: Paired Associates Learning PRM: Pattern Recognition Memory; SWM: Spatial Working Memory; RAVLT: Rey’s Auditory Verbal Learning Test; SCD: subjective cognitive decline: SD: standard deviation; SI: subtle impairment; TMT: Trail Making Test; WMS-IV: Wechsler Memory Scale- Fourth Edition
The associations between CSF pattern and neuropsychological profiles are reported in Figure 1. CN/CANTAB- showed A-/T- CSF profile in 6 out of 7 subjects (86%). None of them showed A+/T- or A+/T+ profile. SI/CANTAB+ subjects showed the highest prevalence of A+/T+ profile (14/35, 40%). SI/CANTAB+ was significantly associated with A+/T+ (p=0.03), compared to CN/CANTAB- with A-/T- (Fisher exact test for count data).
CANTAB- = normal performance; CANTAB+ = at least one altered score
Successively, we wanted to explore which neuropsychological measure could best predict the presence of an AD-like CSF profile. Nineteen variables (age, education, traditional tests, n=13; CANTAB outcome measures, n=4) were included in the LASSO regression. After variables screening selection, two variables remained significant predictors of CSF A+/T+ profile: age and SWM-between error (Supplementary Material, Figure S1). Of interest, SWM-between error remained significant associated with A+/T+ by logistic regression, even by assuming age, gender and education as covariates.
Discussion
In front of the forthcoming availability of disease-modifying agents for treating Alzheimer’s Disease, the ideal subjects to be treated belong to the preclinical stage of AD, ie, functionally healthy individuals with no/minimal cognitive deficits. Unfortunately, in clinical practice, we are still unable to define how to screen and select these subjects, who could most benefit from these treatments.
To this aim, the first need is to find objective, repeatable and reliable measures of subtle cognitive impairment justifying the subsequent diagnostic work-up by means of biomarkers assessment.
So far, attempts to identify such minimal cognitive deficits by means of neuropsychological measures have provided inconsistent findings (50–52). Our approach is to combine two complementary neuropsychological measures, ie, the traditional, domain-oriented, batteries and CANTAB computerized battery, which gives the simultaneous assessment of multiple sub-processes underlying cognitive functioning.
In this retrospective study we observed that 40% of SCD subjects with subtle deficits in both traditional and computerized neuropsychological measures have a CSF AD-like profile (A+T+) while none of SCD cases with a completely normal neuropsychological profile at the two measures show a pathological CSF profile. This finding is in line with a recent paper by Jessen and colleagues (19). In it, a rate of ≈40% amyloid positivity, based on either CSF Aß42/Aß40 or amyloid-PET, was documented in SCD subjects referring to a Memory Clinics, and showing subtle deficits at neuropsychological assessment. These findings should prompt us to carefully consider, in Memory Clinics, the need of a systematic diagnostic approach to SCD individuals who want to know the cause of the disturbances they perceive.
As an exploratory analysis, we found that CANTAB SWM-between errors resulted the only measure able to predict CSF AD biomarkers positivity. Although statistically significant, we cannot generalize this finding. Single-measure approaches have been largely criticized for the assessment of Mild Cognitive Impairment since they may increase the risk of either under- or over-estimation of the magnitude of cognitive decline (one-test approach) (53). The assumption of a cognitive impairment being captured by a single measure, as well as the consequent use of a single test to assess a cognitive domain, should be even more avoided when we are dealing with subjects with SCD. A more complex neuropsychological approach that imply the combination of complementary cognitive measures is necessary for the detection of subtle but objective deficits in SCD subjects. In fact, as our data suggest, the assessment of neuropsychological profile by means of both traditional domain-oriented tests and computerized batteries, ie CANTAB, may further contribute to the accurate identification of subjects requiring biomarker assessment. This statement implies the need to have qualified neuropsychologists devoted to the assessment of subtle cognitive deficits.
As limitations of our explorative study, we must acknowledge the relative paucity of subjects and the single-center sample, which may have reduced the representativeness of SCD subjects. Replicating this work on larger and multi-centric cohorts are thus needed to validate the results of our observations.
In conclusion, a high percentage of subjects with subjective cognitive decline may have a CSF profile compatible with Alzheimer’s Disease. This finding poses the need to operationalize the neuropsychological approach to these subjects in Memory Clinics, in order to make it available for diagnostic purposes. The added value of combined traditional and computerized measures for objectifying subtle cognitive deficits needs to be confirmed by means of larger, longitudinal cohorts of SCD subjects characterized with CSF AD biomarkers.
Acknowledgements: G.B. is supported by the Postdoctoral Fellowship for Basic Scientists grant of the Parkinson’s Foundation (Award ID: PF-PRF-934916).
Funding: This research received no external funding. Open Access funding provided by Università degli Studi di Perugia within the CRUI-CARE Agreement.
Ethical standards: All the procedures were performed in accordance with Declaration of Helsinki and International Conference on Harmonization guidelines for Good Clinical Practice. Our study was approved by Ethics Committee Umbria, 9 October 2008, protocol N.19369/08/AV, registry N. 1287/08.
Conflict of interest: E.C., G.B., N.S., C.M., L.G., F.P.P., L.P. declare no conflict of interest.
Open Access: This article is distributed under the terms of the Creative Commons Attribution 4.0 International License (http://creativecommons.org/licenses/by/4.0/), which permits use, duplication, adaptation, distribution and reproduction in any medium or format, as long as you give appropriate credit to the original author(s) and the source, provide a link to the Creative Commons license and indicate if changes were made.
References
1. Price JL, Morris JC. Tangles and plaques in nondemented aging and preclinical Alzheimer’s disease. Ann Neurol. 1999;45(3):358–68. Doi: 10.1002/1531-8249(199903)45:3<358::aid-ana12>3.0.co;2-x.
2. Price JL, McKeel DW, Buckles VD, et al. Neuropathology of nondemented aging: Presumptive evidence for preclinical Alzheimer disease. Neurobiol Aging. 2009; 30(7):1026–36. doi: 10.1016/j.neurobiolaging.2009.04.002.
3. Bateman RJ, Xiong C, Benzinger TLS, et al. Clinical and Biomarker Changes in Dominantly Inherited Alzheimer’s Disease. N Engl J Med. 2012;367(9):795–804. doi: 10.1056/NEJMoa1202753.
4. Villemagne VL, Burnham S, Bourgeat P, et al. Amyloid β deposition, neurodegeneration, and cognitive decline in sporadic Alzheimer’s disease: a prospective cohort study. Lancet Neurol. 2013;12(4):357–67. doi: 10.1016/S1474-4422(13)70044-9.
5. Long JM, Coble DW, Xiong C, et al. Preclinical Alzheimer’s disease biomarkers accurately predict cognitive and neuropathological outcomes. Brain. 2022; 145(12):4506–18. doi: 10.1093/brain/awac250.
6. Jack CR, Knopman DS, Jagust WJ, et al. Tracking pathophysiological processes in Alzheimer’s disease: an updated hypothetical model of dynamic biomarkers. Lancet Neurol. 2013;12(2):207–16. doi: 10.1016/S1474-4422(12)70291-0.
7. Jack CR, Bennett DA, Blennow K, et al. NIA-AA Research Framework: Toward a biological definition of Alzheimer’s disease. Alzheimers Dement. 2018;14(4):535–62. doi: 10.1016/j.jalz.2018.02.018.
8. Dubois B, Hampel H, Feldman HH, et al. Preclinical Alzheimer’s disease: Definition, natural history, and diagnostic criteria. Alzheimers Dement. 2016;12(3):292-323. doi:10.1016/j.jalz.2016.02.002.
9. Parnetti L, Chipi E, Salvadori N, D’Andrea K, Eusebi P. Prevalence and risk of progression of preclinical Alzheimer’s disease stages: a systematic review and meta-analysis. Alzheimers Res Ther. 2019;11(1):7. doi:10.1186/s13195-018-0459-7.
10. Jessen F, Amariglio RE, van Boxtel M, et al. A conceptual framework for research on subjective cognitive decline in preclinical Alzheimer’s disease. Alzheimers Dement. 2014;10(6):844-852. doi:10.1016/j.jalz.2014.01.001.
11. Jessen F, Amariglio RE, Buckley RF, et al. The characterisation of subjective cognitive decline. Lancet Neurol. 2020;19(3):271-278. doi:10.1016/S1474-4422(19)30368-0.
12. Janssen O, Jansen WJ, Vos SJB, et al. Characteristics of subjective cognitive decline associated with amyloid positivity. Alzheimers Dement. 2022;18(10):1832-1845. doi:10.1002/alz.12512.
13. Jansen WJ, Janssen O, Tijms BM, et al. Prevalence Estimates of Amyloid Abnormality Across the Alzheimer Disease Clinical Spectrum. JAMA Neurol. 2022;79(3):228-243. doi:10.1001/jamaneurol.2021.5216.
14. Wolfsgruber S, Kleineidam L, Guski J, et al. Minor neuropsychological deficits in patients with subjective cognitive decline. Neurology. 2020;95(9):e1134-e1143. doi:10.1212/WNL.0000000000010142.
15. Ebenau JL, Timmers T, Wesselman LMP, et al. ATN classification and clinical progression in subjective cognitive decline: The SCIENCe project. Neurology. 2020;95(1):e46-e58. doi:10.1212/WNL.0000000000009724.
16. Eckerström M, Göthlin M, Rolstad S, et al. Longitudinal evaluation of criteria for subjective cognitive decline and preclinical Alzheimer’s disease in a memory clinic sample. Alzheimers Dement. 2017;8:96-107. doi:10.1016/j.dadm.2017.04.006.
17. Rostamzadeh A, Bohr L, Wagner M, Baethge C, Jessen F. Progression of Subjective Cognitive Decline to MCI or Dementia in Relation to Biomarkers for Alzheimer Disease: A Meta-analysis. Neurology. 2022;99(17):e1866-e1874. doi:10.1212/WNL.0000000000201072.
18. Slot RER, Sikkes SAM, Berkhof J, et al. Subjective cognitive decline and rates of incident Alzheimer’s disease and non-Alzheimer’s disease dementia. Alzheimers Dement. 2019;15(3):465-476. doi:10.1016/j.jalz.2018.10.003.
19. Jessen F, Wolfsgruber S, Kleineindam L, et al. Subjective cognitive decline and stage 2 of Alzheimer disease in patients from memory centers. Alzheimers Dement. 2022;10.1002/alz.12674. doi:10.1002/alz.12674.
20. Epelbaum S, Genthon R, Cavedo E, et al. Preclinical Alzheimer’s disease: A systematic review of the cohorts underlying the concept. Alzheimers Dement. 2017;13(4):454-467. doi:10.1016/j.jalz.2016.12.003.
21. Loewenstein DA, Greig MT, Schinka JA, et al. An investigation of PreMCI: subtypes and longitudinal outcomes. Alzheimers Dement. 2012;8(3):172-179. doi:10.1016/j.jalz.2011.03.002.
22. Edmonds EC, Delano-Wood L, Galasko DR, Salmon DP, Bondi MW; Alzheimer’s Disease Neuroimaging Initiative. Subtle Cognitive Decline and Biomarker Staging in Preclinical Alzheimer’s Disease. J Alzheimers Dis. 2015;47(1):231-242. doi:10.3233/JAD-150128.
23. Robbins TW, James M, Owen AM, Sahakian BJ, McInnes L, Rabbitt P. Cambridge Neuropsychological Test Automated Battery (CANTAB): a factor analytic study of a large sample of normal elderly volunteers. Dementia. 1994;5(5):266-281. doi:10.1159/000106735.
24. Sahakian BJ, Morris RG, Evenden JL, et al. A comparative study of visuospatial memory and learning in Alzheimer-type dementia and Parkinson’s disease. Brain. 1988;111 (Pt 3):695-718. doi:10.1093/brain/111.3.695.
25. Juncos-Rabadán O, Facal D, Pereiro AX, Lojo-Seoane C. Visual memory profiling with CANTAB in mild cognitive impairment (MCI) subtypes. Int J Geriatr Psychiatry. 2014;29(10):1040-1048. doi:10.1002/gps.4095.
26. de Rover M, Pironti VA, McCabe JA, et al. Hippocampal dysfunction in patients with mild cognitive impairment: a functional neuroimaging study of a visuospatial paired associates learning task. Neuropsychologia. 2011;49(7):2060-2070. doi:10.1016/j.neuropsychologia.2011.03.037.
27. Blackwell AD, Sahakian BJ, Vesey R, Semple JM, Robbins TW, Hodges JR. Detecting dementia: novel neuropsychological markers of preclinical Alzheimer’s disease. Dement Geriatr Cogn Disord. 2004;17(1-2):42-48. doi:10.1159/000074081.
28. Junkkila J, Oja S, Laine M, Karrasch M. Applicability of the CANTAB-PAL computerized memory test in identifying amnestic mild cognitive impairment and Alzheimer’s disease. Dement Geriatr Cogn Disord. 2012;34(2):83-89. doi:10.1159/000342116.
29. Duke Han S, Nguyen CP, Stricker NH, Nation DA. Detectable Neuropsychological Differences in Early Preclinical Alzheimer’s Disease: A Meta-Analysis. Neuropsychol Rev. 2017;27(4):305-325. doi:10.1007/s11065-017-9345-5.
30. Papp KV, Rentz DM, Mormino EC, et al. Cued memory decline in biomarker-defined preclinical Alzheimer disease. Neurology. 2017;88(15):1431-1438. doi:10.1212/WNL.0000000000003812.
31. Harrington MG, Chiang J, Pogoda JM, et al. Executive function changes before memory in preclinical Alzheimer’s pathology: a prospective, cross-sectional, case control study. PLoS One. 2013;8(11):e79378. doi:10.1371/journal.pone.0079378.
32. Magni E, Binetti G, Bianchetti A, Rozzini R, Trabucchi M. Mini-Mental State Examination: a normative study in Italian elderly population. Eur J Neurol. 1996;3(3):198-202. doi:10.1111/j.1468-1331.1996.tb00423.x.
33. Montanucci C, Chipi E, Salvadori N, Rinaldi R, Eusebi P, Parnetti L. HIV-Dementia Scale as a screening tool for the detection of subcortical cognitive deficits: validation of the Italian version. J Neurol. 2021;268(12):4789-4795. doi:10.1007/s00415-021-10592-9.
34. Giovagnoli AR, Del Pesce M, Mascheroni S, Simoncelli M, Laiacona M, Capitani E. Trail making test: normative values from 287 normal adult controls. Ital J Neurol Sci. 1996;17(4):305-309. doi:10.1007/BF01997792.
35. Monaco M, Costa A, Caltagirone C, Carlesimo GA. Forward and backward span for verbal and visuo-spatial data: standardization and normative data from an Italian adult population. Neurol Sci. 2013;34(5):749-754. doi:10.1007/s10072-012-1130-x.
36. Carlesimo GA, Caltagirone C, Gainotti G. The Mental Deterioration Battery: normative data, diagnostic reliability and qualitative analyses of cognitive impairment. The Group for the Standardization of the Mental Deterioration Battery. Eur Neurol. 1996;36(6):378-384. doi:10.1159/000117297.
37. Novelli G, Papagno C, Capitani E, Laiacona M, Vallar G, Cappa SF. Tre test clinici di ricerca e produzione lessicale. Taratura su soggetti normali. Arch Psicol Neurol Psichiatr. 1986; 47(4), 477–506.
38. Spinnler, H. Standardizzazione e taratura italiana di test neuropsicologici. Ital J Neurol Sci; 1987; 6,47-50.
39. Capitani E, Laiacona M. Aging and psychometric diagnosis of intellectual impairment: some considerations on test scores and their use. Dev Neuropsychol. 1988;4:325–30. doi:10.1080/87565648809540416.
40. CANTAB® [Cognitive assessment software]. Cambridge Cognition (2022). All rights reserved. www.cantab.com.
41. Owen AM. The role of the lateral frontal cortex in mnemonic processing: the contribution of functional neuroimaging. Exp Brain Res. 2000;133(1):33-43. doi:10.1007/s002210000398.
42. Summers MJ, Saunders NL. Neuropsychological measures predict decline to Alzheimer’s dementia from mild cognitive impairment. Neuropsychology. 2012;26(4):498-508. doi:10.1037/a0028576.
43. Klekociuk SZ, Summers MJ. The learning profile of persistent mild cognitive impairment (MCI): a potential diagnostic marker of persistent amnestic MCI. Eur J Neurol. 2014;21(3):470-e24. doi:10.1111/ene.12333.
44. Abbott RA, Skirrow C, Jokisch M, et al. Normative data from linear and nonlinear quantile regression in CANTAB: Cognition in mid-to-late life in an epidemiological sample. Alzheimers Dement (Amst). 2018;11:36-44. doi:10.1016/j.dadm.2018.10.007
45. Teunissen CE, Petzold A, Bennett JL, et al. A consensus protocol for the standardization of cerebrospinal fluid collection and biobanking. Neurology. 2009;73(22):1914-1922. doi:10.1212/WNL.0b013e3181c47cc2.
46. Bellomo G, Indaco A, Chiasserini D, et al. Machine Learning Driven Profiling of Cerebrospinal Fluid Core Biomarkers in Alzheimer’s Disease and Other Neurological Disorders. Front Neurosci. 2021;15:647783. doi:10.3389/fnins.2021.647783.
47. Gaetani L, Bellomo G, Parnetti L, Blennow K, Zetterberg H, Di Filippo M. Neuroinflammation and Alzheimer’s Disease: A Machine Learning Approach to CSF Proteomics. Cells. 2021;10(8):1930. doi:10.3390/cells10081930.
48. Friedman J, Hastie T, Tibshirani R. Regularization Paths for Generalized Linear Models via Coordinate Descent. J Stat Softw. 2010;33(1):1-22.
49. Robin X, Turck N, Hainard A, et al. pROC: an open-source package for R and S+ to analyze and compare ROC curves. BMC Bioinformatics. 2011;12:77. doi:10.1186/1471-2105-12-77.
50. Rentz DM, Parra Rodriguez MA, Amariglio R, Stern Y, Sperling R, Ferris S. Promising developments in neuropsychological approaches for the detection of preclinical Alzheimer’s disease: a selective review. Alzheimers Res Ther. 2013;5(6):58.. doi:10.1186/alzrt222.
51. Öhman F, Hassenstab J, Berron D, Schöll M, Papp KV. Current advances in digital cognitive assessment for preclinical Alzheimer’s disease. Alzheimers Dement. 2021;13(1):e12217. doi:10.1002/dad2.12217.
52. Chipi E, Salvadori N, Farotti L, Parnetti L. Biomarker-Based Signature of Alzheimer’s Disease in Pre-MCI Individuals. Brain Sci. 2019;9(9):213. doi:10.3390/brainsci9090213.
53. Bondi, M. W., Edmonds, E. C., Jak, A. J., et al. Neuropsychological criteria for mild cognitive impairment improves diagnostic precision, biomarker associations, and progression rates. J Alzheimers Dis. 2014; 42(1), 275–289. Doi:10.3233/JAD-140276
© The Authors 2023