S. Roldan Munoz1, S.T. de Vries1,2, G. Lankester3, F. Pignatti4, B.C. van Munster5, I. Radford3, L. Guizzaro4,6, P.G.M. Mol1,2, H. Hillege2,7, D. Postmus4,7
1. University of Groningen, University Medical Center Groningen, Department of Clinical Pharmacy and Pharmacology, Groningen, The Netherlands; 2. Dutch Medicines Evaluation Board, Utrecht, The Netherlands; 3. Alzheimer’s Research UK, United Kingdom; 4. European Medicines Agency, Amsterdam, The Netherlands; 5. University of Groningen, University Medical Center Groningen, Groningen, Department of Internal Medicine and Geriatrics, The Netherlands; 6. Statistica Medica, Università Della Campania Luigi Vanvitelli, Napoli, Italy; 7. University of Groningen, University Medical Center Groningen, Department of Epidemiology, Groningen, The Netherlands.
Corresponding Author: Sonia Roldan Munoz, University Medical Center Groningen, Department of Clinical Pharmacy and Pharmacology. Hanzeplein 1, 9713 GZ Groningen; Building 50, entrance 45, 1st floor, Room 50.1.C.003. Department zip code AP50, mailbox 30.001. 9700 RB Groningen, s.roldan.munoz@umcg.nl
J Prev Alz Dis 2023;4(10):756-764
Published online June 30, 2023, http://dx.doi.org/10.14283/jpad.2023.84
Abstract
BACKGROUND: Treatments aiming at slowing down the progression of Alzheimer’s disease (AD) may soon become available. However, information about the risks that people are willing to accept in order to delay the progression of the disease is limited.
OBJECTIVE: To determine the trade-offs that individuals are willing to make between the benefits and risks of hypothetical treatments for AD, and the extent to which these trade-offs depend on individuals’ characteristics and beliefs about medicines.
DESIGN: Online, cross-sectional survey study.
SETTING: Population in the UK. Public link to the survey available at the websites of Alzheimer’s Research UK and Join Dementia Research.
PARTICIPANTS: Everyone self-reported ≥18 years old was eligible to participate. A total of 4384 people entered the survey and 3658 completed it.
MEASUREMENTS: The maximum acceptable risks (MARs) of participants for moderate and severe adverse events in exchange for a 2-year delay in disease progression. The risks were expressed on ordinal scales, from <10% to ≥50%, above a pre-existing risk of 30% for moderate adverse events and 10% for severe adverse events. We obtained the population median MARs using log-normal survival models and quantified the effects of individuals’ characteristics and beliefs about medicines in terms of acceleration factors.
RESULTS: For the moderate adverse events, 26% of the participants had a MAR ≥50%, followed by 25% of the participants with a MAR of 10 to <20%, giving an estimated median MAR of 25.4% (95% confidence interval [CI] 24.5 to 26.3). For the severe adverse events, 43% of the participants had a MAR <10%, followed by 25% of the participants with a MAR of 10 to <20%, resulting in an estimated median MAR of 12.1% (95%CI 11.6 to 12.5). Factors that were associated with the individuals’ MARs for one or both adverse events were age, gender, educational level, living alone, and beliefs about medicines. Whether or not individuals were living with memory problems or had experience as a caregiver had no effect on the MARs for any of the adverse events.
CONCLUSION: Trade-offs between benefits and risks of AD treatments are heterogeneous and influenced by individuals’ characteristics and beliefs about medicines. This heterogeneity should be acknowledged during the medicinal product decision-making in order to fulfil the needs of the various subpopulations.
Key words: Alzheimer’s disease, stated preferences, benefit-risk trade-offs, threshold technique, maximum acceptable risk.
Introduction
Currently, the management of AD is limited to a combination of pharmaceutical and non-pharmaceutical treatments to alleviate symptoms. However, with the development of new compounds targeting the pathophysiology of the disease, treatments aimed at slowing down disease progression may also become available (1). The first such potentially disease-modifying treatment on the market is aducanumab, approved by the United States (US) Food and Drug Administration (FDA) in 2021 (2). Other regulatory agencies, such as the European Medicines Agency (EMA), however, did not approve this treatment due to inconclusive therapeutic benefits and observed serious adverse events, most commonly amyloid-related imaging abnormalities (2-5).
To support the medical product decision-making for novel treatments for AD, better understanding is needed of how preferences over possible treatment outcomes are distributed in the population, and the extent to which these preferences differ between subgroups of the population (6). Previous studies have collected quantitative data on the maximum risk that individuals are willing to accept to delay the progression of AD (7-9). However, these studies were performed in unimpaired older adults and provided no information about the views of those living with memory problems. Exploring the preferences of this group is challenging but pertinent, since previous studies focusing on chronic diseases have shown differences in preferences between people living with and without the disease (10-14) or between people living with the disease and caregivers (15-17). Besides sociodemographic characteristics and aspects related to symptoms and disease experience, preferences for future AD treatments may also be influenced by psychological factors, such as individuals’ beliefs about medicines (7, 18). This psychological factor has been previously found to be associated with medication use for chronic diseases and preventive treatments, such as diabetes, asthma, and stroke (19-21). The effect of such beliefs on stated preferences for hypothetical treatments for AD is however not known.
The aims of this study were to determine the trade-offs that people are willing to make between the benefits and risks of hypothetical treatments for AD and to assess the extent to which these trade-offs depend on the individuals’ characteristics and beliefs about medicines.
Methods
Study design & participants
We conducted an online, cross-sectional survey study among people in the UK. An open link to the survey was published on the websites of Alzheimer’s Research UK (ARUK) (https://www.alzheimersresearchuk.org/) and Join Dementia Research (JDR) (https://www.joindementiaresearch.nihr.ac.uk/). The survey was open from 17th January 2022 to 7th March 2022 and responses were collected anonymously. The information letter provided to the participants and The Checklist for Reporting Results of Internet E-Surveys (CHERRIES checklist) are available in the supplementary appendix text 1 and supplementary appendix table 1.
Survey
The survey was in English and was created in the web application Research Electronic Data Capture 10.0.23 (REDCap – www.projectredcap.org) (supplementary appendix text 2). It consisted of three instruments: questions about individuals´ characteristics, the Beliefs about Medicines questionnaire (BMQ), and a preference elicitation exercise about hypothetical treatments for AD.
Individuals’ characteristics
These included sociodemographic characteristics and health-related questions. The sociodemographic characteristics were gender (female/male/other), age (continuous), educational level (grouped as low, middle, and higher education), experience as a caregiver for AD dementia (yes/no), and living alone (yes/no).
The health-related questions included whether they had ever consulted a doctor about their brain health (yes/no), self-reported brain health (visual analogue scale [VAS] ranging from 0 to 100), help needed with daily activities (e.g. cooking, eating, or keeping finances [yes/no]), help needed to fill out the survey (yes/no), and a short memory exercise. The memory exercise was based on a telephone screener for mild cognitive impairment (MCI) (22) and consisted of recalling three words in a free text entry two times (immediate recall and delayed recall: we used the questions about their beliefs about medicines as an intermediate task). Based on the question “Have you ever consulted a doctor about your brain health?”, participants were classified into people living with memory problems and people living without memory problems. The other health-related questions were utilized to confirm whether there were differences in cognitive capabilities between these two groups and they were all statistically different (supplementary appendix table 2).
Beliefs about medicines
Information about individuals’ beliefs about medicines was collected through the BMQ-General (23). The BMQ-General includes three subscales, i.e. benefits (e.g. “Medicines help many people to live longer”), harms (e.g. “All medicines are poisons”), and overuse (e.g. “Doctors place too much trust on medicines”). Each of these subscales contains four items to be answered on a five-point Likert scale. Therefore, the scores of each subscale can range between four (strongly disagree) and 20 (strongly agree) (23). For each BMQ subscale, missing values were imputed using the participant’s mean value of the subscale when no more than two items per subscale were missing. Otherwise, the whole subscale was considered missing.
Preference elicitation exercise about hypothetical AD treatments
The preference elicitation exercise was introduced by an initial vignette description asking participants to imagine they were experiencing memory and thinking problems. Participants then performed two exercises based on the threshold technique, where they had to choose between treatment A and B, defined by two attributes (i.e. a therapeutic benefit and an adverse event). The threshold technique is an adaptative approach to collect data on an individual’s maximum acceptable risk (MAR) (24). The MAR is defined by Hauber et al. (2009) as the “maximum treatment-related risk, above any pre-existing risk, that subjects are willing to accept in exchange for a given therapeutic benefit.” (7). In our survey, the therapeutic benefit was a delay in AD progression, defined as the number of years until more care and support is needed. The treatment-related risks were (i) the risk of experiencing moderate adverse events, defined as the number of people who experience periods of discomfort, and (ii) the risk of experiencing severe adverse events, defined as the number of people who experience a side effect that could cause long-term or permanent disability. The two adverse events were assessed in separate thresholding exercises. The question trees that we used to identify the participants’ MARs for the two treatment-related risks are schematically represented in figure 1. In short, both exercises started with an initial choice between two hypothetical treatments that was the same to all participants. Depending on a participant’s response to this first question, the risk of adverse events associated with the second treatment was increased or decreased until the end of that branch of the question tree was reached. The selection and definition of the attributes was based on previous literature (7, 8, 25-31) and the input of clinical doctors, researchers, and medicines regulators with expertise in AD dementia. The levels of the attributes were based on previous literature (7, 8, 32) and the input of people living with MCI. Further details about the selection of attributes, their definitions and levels are provided in supplementary appendix text 3.
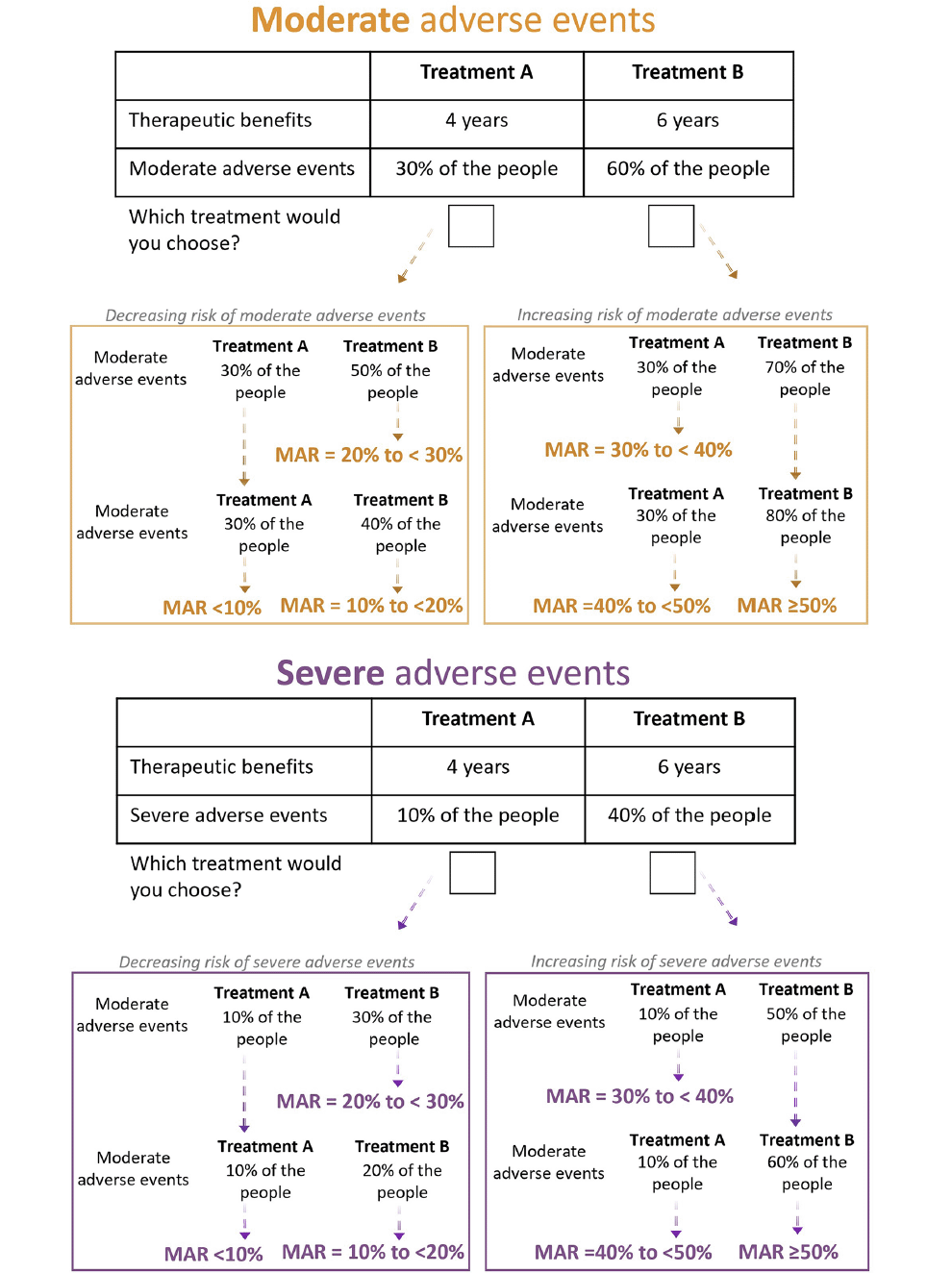
Figure 1. Question trees used to identify the participants’ MARs for the moderate and the severe adverse events in exchange for a 2-year delay in disease progression
MAR=Maximum acceptable risk
Patient involvement
We included the input of people living with MCI during the development of the survey through a focus group. The focus group consisted of eight participants, all of them with a self-reported diagnosis of MCI ranging from 18 months to 5 years. Participants completed an early version of the survey before the start of the focus group to test technical aspects, ease of use, and functionality. During the focus group, we discussed various topics, including difficulty of the memory exercise, capability and emotional impact of imagining future stages of their disease, definitions of therapeutic benefits and moderate and severe adverse events, and language, length, and general difficulty of the survey. We adapted the survey based on their feedback (e.g. we changed the definition of the adverse events, and we increased the levels of the attributes). The final survey is available in the supplementary appendix text 2.
Statistical analysis
We included in the statistical analyses all participants who completed the two thresholding exercises and at least one of the questions about individuals’ characteristics.
We summarized the MAR distributions for each of the two adverse events graphically using histograms and survival curves estimated using Turnbull’s non-parametric estimator for interval-censored data. We fitted interval-censored log-normal survival models to estimate the median MAR per adverse event. Subsequently, we extended these log-normal survival models with covariates to estimate the effects of the individuals’ characteristics and beliefs about medicines. Both unadjusted and mutually adjusted models with all covariates that were significant in the unadjusted models were fitted. We selected the log-normal distribution because it had the lowest value of Akaike information criterion (AIC) out of several accelerated failure time models fitted to the data (i.e. log logistic, log-normal, and Weibull). We presented the covariate effects in terms of acceleration factors, which show how the median MAR changes in response to changes in the covariate values (33). We considered p-values <0.05 as statistically significant. We performed the analyses in R version 4.0.2 (R Foundation for Statistical Computing, Vienna, Austria. URL https://www.R-project.org/). This study followed the STROBE recommendations.
Results
Out of the 4384 times the survey link was opened, there were 3658 responses that fulfilled the criteria to be included in the analyses (supplementary appendix figure 1). The median age of the participants was 64 years (interquartile range [IQR] 55 ‒ 70). Most frequently, participants were female (74%), highly educated (70%), and not living alone (78%). Out of the 3658 participants, 2416 (66%) reported to have experience as caregivers for people living with AD and 554 (15%) reported to be living with memory problems (these were not mutually exclusive responses). Participants agreed most with the beliefs about benefits of medicines (median score 16, IQR 15 – 17), followed by the beliefs about overuse of medicines (median score 12, IQR 10 – 13), and the beliefs about harm of medicines (median score 8, IQR 7 – 14) (table 1).
Missing responses: Age = 12 (0.00%), gender = 5 (0.00%), educational level = 30 (0.01%), living alone = 58 (0.01%). *Educational level was grouped as low (no formal qualifications), middle (secondary school, A level, or equivalent), and high (degree, postgraduate degree, or equivalent). $ Beliefs about medicines were measured with the Beliefs about Medicines Questionnaire General (BMQ-General). The BMQ-General is copyrighted (©Professor Robert Horne).
The histograms of the MAR distributions for the moderate and severe adverse events are shown in figure 2. For the moderate adverse events, the largest group of participants (n=952, 26%) had a MAR ≥50%, followed by 25% of the participants (n=904) having a MAR of 10 to <20%. For the severe adverse events, 43% of the participants (n=1573) had a MAR <10%, followed by 25% of the participants (n=923) with a MAR of 10 to <20%. The estimated survival curves for the MARs for the moderate and severe adverse events are shown in figure 3. Based on the fitted log-normal survival models, the estimated median MARs for the moderate and severe adverse events were 25.4% (95% confidence interval [CI] 24.5 to 26.3) and 12.1% (95% CI 11.6 to 12.5), respectively.
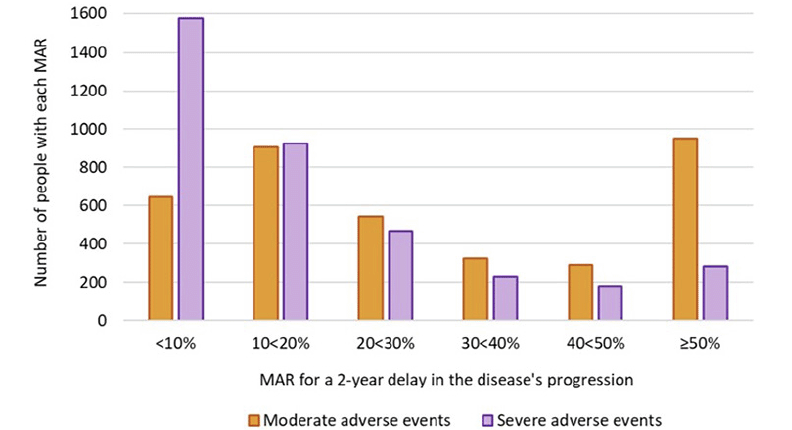
Figure 2. Histograms showing the distribution of MARs for the moderate and severe adverse events in exchange for a 2-year delay in disease progression
MAR=Maximum acceptable risk
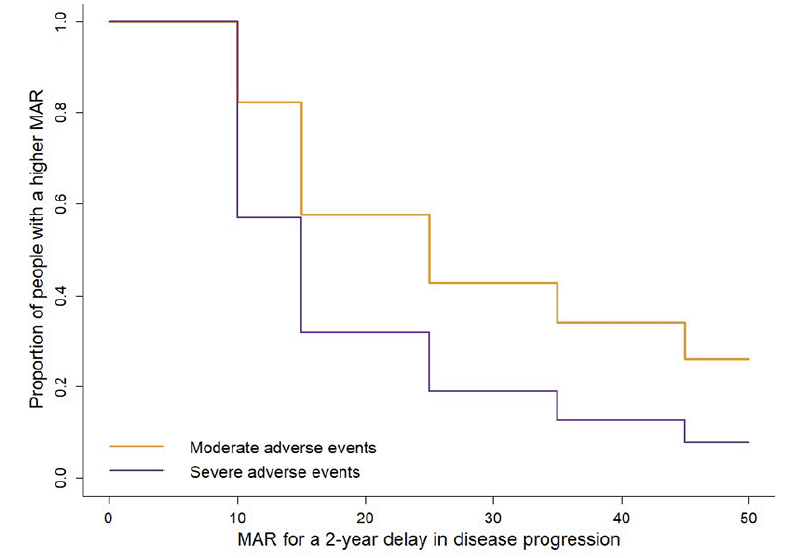
Figure 3. Survival curves showing the remaining proportion of participants at the various MARs for moderate and severe adverse events in exchange for a 2-year delay in disease progression
MAR=Maximum acceptable risk
The results of the unadjusted and adjusted log-normal regression analyses are shown in table 2 and supplementary appendix table 3, respectively. In the unadjusted models, several individuals’ characteristics were associated with the MAR for the moderate adverse events, with median MAR values being higher in people who were younger (p<0.001), male (p<0.001), highly educated (p<0.001), and living alone (p<0.001). Age (p<0.001), gender (p<0.001), and living alone (p=0.017) had a similar effect on the median MAR values for the severe adverse events. Educational level was, however, not associated with the MAR for the severe adverse events (p=0.250). The median MARs for the moderate and severe adverse events were not influenced by having experience as a caregiver (p=0.880 and p=0.180, respectively) or being a person living with memory problems (p=0.790 and p=0.420, respectively). Finally, for the beliefs about medicines, higher scores on the beliefs about the benefits of medicines were associated with higher median MAR values for the moderate (p<0.001) and severe (p=0.007) adverse events. For the other subscales, higher scores on the beliefs about overuse of medicines were associated with lower median MAR values for both the moderate (p<0.001) and the severe adverse events (p=0.003), while higher scores on the beliefs about harm of medicines were only associated with lower median MAR values (p<0.001) for the moderate adverse events. In the mutually adjusted models, the same associations were found for the median MAR values for the moderate adverse events. For the median MAR values for the severe adverse events, living alone and the beliefs about medicines were no longer statistically significant (supplementary appendix table 3).
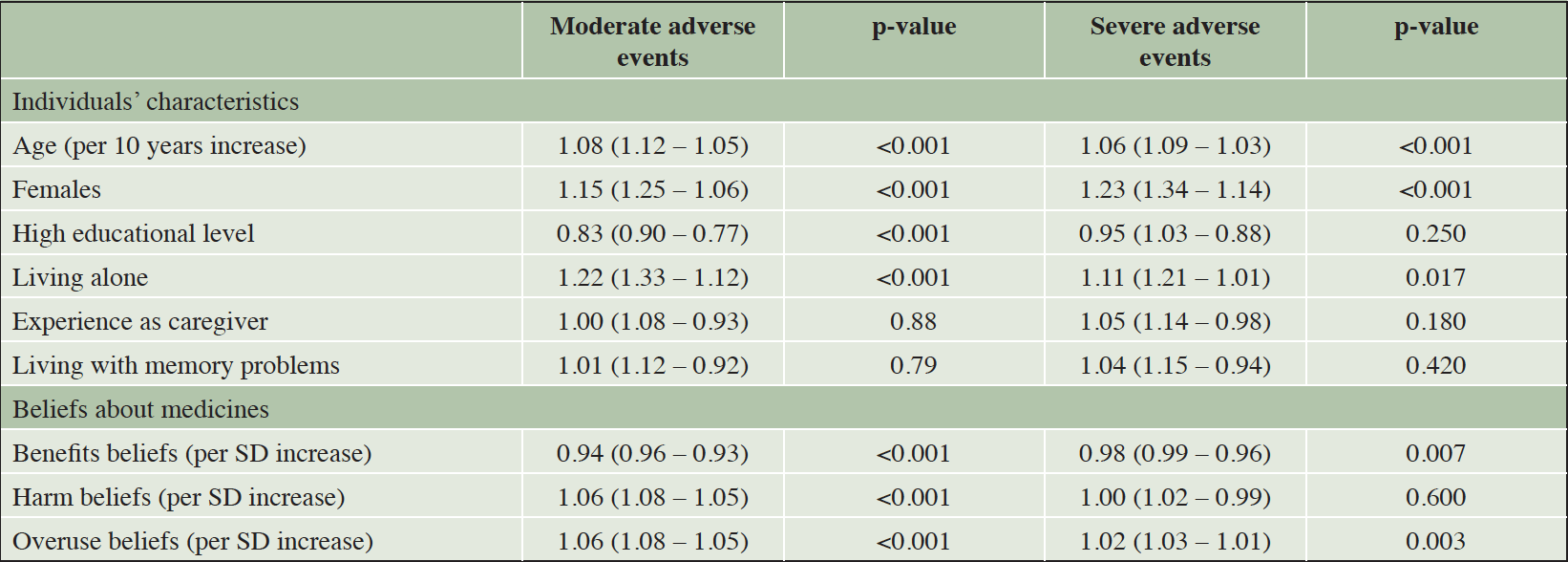
Table 2. Results of the unadjusted models showing the effect of the individuals’ characteristics and beliefs about medicines on the MAR for the moderate and the severe adverse events. Effects are expressed in terms of acceleration factors
Numbers below 1 indicate increase in the MAR and numbers above 1 indicate decrease in the MAR. For categorical variables, the reference groups were males, low/middle educational level, and not living alone. SD = standard deviation.
Discussion
Using an online, cross-sectional survey, we elicited the MARs of people living in the UK for moderate and severe adverse events in exchange for a 2-year delay in the time until AD progresses to a more severe stage. The median MAR was 25.4% for the moderate adverse events and 12.1% for the severe adverse events. For both adverse events, the MAR was heterogeneous and influenced by age, gender, and individuals’ beliefs about medicines. The MAR for the moderate adverse events was additionally influenced by educational level and living alone. Living with memory problems or having experience as a caregiver had no effect on either of the two MARs.
In a previously performed stated preference study in an elderly population with no symptoms of cognitive impairment, participants were willing to accept an 11% increase in the risk of stroke and a 13% increase in the risk of death in exchange for one additional year with normal memory (8). Another study performed in a similar population found that the mean MAR of treatment-related death or permanent severe disability due to stroke for a 2-year improvement in the time until progression to moderate AD was 22% (7). Compared to these previous studies, the MAR for severe adverse events was lower in our study. A possible explanation for this may lay in differences in the definitions of the attributes (e.g. therapeutic benefits: a fixed life expectancy with treatment resulting in spending a longer time in a less severe AD stage versus a delay in disease progression without specifying the survival time). Our study was conducted in the UK, while the other two studies were conducted in the US: heterogeneity in patient preferences between countries has previously been described (34, 35).
As well as eliciting the MAR for severe adverse events, we assessed trade-offs between delaying disease progression and the risk of experiencing moderate adverse events, defined as having periods of discomfort that affect the ability to carry out daily activities. We chose adverse events such as dizziness, nausea, or diarrhoea which have been shown in previous qualitative and quantitative studies in chronic diseases other than AD to be significant factors in decreasing patients’ health-related quality of life. In these studies, patients needed large benefits in order to accept an increase in nausea and would accept additional costs in order to prevent such adverse events from occurring (36-38). Our study, however, showed that up to 26% of the respondents were willing to accept a MAR ≥50% for moderate adverse events in exchange for a 2-year delay in disease progression. A possible explanation for this high MAR might be the irreversible consequences of the progression of AD, which will lead to less independence and self-sufficiency, and poorer quality of life (39). Furthermore, AD also affects the quality of life of the caregiver, which has previously been shown to be a point of concern for the cared-for individual (40).
With respect to individuals’ characteristics, age and gender influenced the MARs for both adverse events, with younger people and males having higher median MARs. This result is consistent with previous studies showing that older people accept lower MARs and are less keen to add preventive therapy, seemingly being more focused on quality of life rather than life extension or disease progression (7, 8, 41). With regard to gender, males have been shown to perceive various situations as less risky, and they generally accept higher risks than females (42, 43). Our study shows that the same tendency applies to accepting risks for AD treatments.
Our study showed no differences in MARs between people living with and without memory problems or between those with and without experience as caregivers. This finding differs from previous qualitative and quantitative studies in which caregivers and individuals living with MCI, dementia, or AD, showed differences in care values (17, 44). However, these studies were broader, applying to all aspects of care (e.g. the importance of autonomy), and did not show specific definitions of treatment options and range effects, which might have contributed to the differences in results. Another possible explanation could reside in the way we defined people living with memory problems, which allowed for a certain level of interpretation. Nonetheless, the other health-related questions included in our survey showed that there were significant differences in cognitive capabilities between the two groups, showing that our categorization was effective in identifying individuals with early signs of cognitive impairment. An alternative approach could have been to group individuals based on whether or not they had a confirmed diagnosis of early AD dementia. However, because diagnosis of AD usually happens in a later stage of the disease (45), this would risk excluding most of the individuals with early signs of cognitive impairment.
Alongside individuals’ characteristics, we assessed the effect of beliefs about medicines on the MAR. These results showed higher MARs in people with higher beliefs about the benefits of medicines and lower MARs in people with higher beliefs about the harm and overuse of medicines. Beliefs about medicines have mostly been studied in the context of medication adherence or medication use and, to our knowledge, this is the first study in which the impact of the beliefs about medicines on treatment preferences in hypothetical trade-off situations is evaluated. Nevertheless, our findings follow the direction of these previous studies, where positive beliefs led to higher medication use and adherence compared to negative beliefs (20, 21). However, the effect of beliefs about medicines on the MAR was lower than the effect of individuals’ characteristics and its clinical relevance needs to be further studied.
Strengths and limitations
A strength of our study is the large number of participants, which shows the engagement and willingness of people to help in AD research. Respondents were, however, recruited through websites mostly visited by people familiar with or interested in AD. It is possible, therefore, that the results are not representative of the overall population. An indicator of possible selection bias is the large percentage of highly educated individuals (70%), which differs from the general population in the UK (46). Representativeness with regard to other characteristics is unknown.
The study was performed in a broad population, including people living with memory problems. It is essential to understand the preferences of those individuals in an early stage of cognitive impairment since it provides a better understanding of their needs and wishes, and shows the (dis)similarities with those who are not living with the disease and who, at certain stage, might need to take healthcare decisions for them (47).
A limitation inherent to preference studies is that the definition of the attributes and the attribute levels can impact the results. In order to minimise this effect, we specified the severity and duration of the attributes and were guided by previous literature and regulatory guidelines to assess adverse events (e.g. need or not of medical assistance). For the levels of the attributes, we used previous literature (7, 8, 25-30, 32) and information from the pilot study. Still, given the large number of individuals falling within the lowest MAR category for the severe adverse events, it seems reasonable to use lower MAR increments in future stated preference studies related to AD. Another limitation of this type of study is that participants respond to hypothetical questions with limited information. However, their perspectives could be different when making decisions in the real world.
There are different methods to elicit treatment preferences. In this study, we used the threshold technique, which is, compared to other commonly applied preference elicitation techniques, cognitively less demanding. We therefore considered the threshold technique to be the most suitable method for our broad research population, which included people living with memory problems. A downside of the use of thresholding is that it results in MAR values that are interval-censored, thereby complicating the statistical analysis. In previous applications of the threshold technique, interval-censored regression models with Gaussian errors have been used to handle the interval-censored data (48). Assuming a normal distribution for the population MAR values was, however, not appropriate for the preference information collected in our study. To ensure the use of an appropriate distribution for our data, we fitted multiple parametric regression models and selected the one with the best fit based on the AIC. This resulted in the use of log-normal distributions of the MAR for both the moderate and severe adverse events.
Implications and future research
A significant implication of the study is the observed heterogeneity in MAR, which is a relevant factor to consider at the various stages of medical product decision-making. For example, the results of the study may be applicable to regulatory discussions, since more than half of the respondents were willing to accept very high risks for severe adverse events. This may result in differences between the trade-offs of regulators and patients. These results, however, should be interpreted with caution since the information provided to the responders was limited and hypothetical. For example, the clinical therapeutic benefit in our study was clearly defined, was relatively large, and did not elaborate on the possibility of lack of effect, which are common circumstances that regulators need to consider when evaluating a treatment (49-51). The existence of new treatments provides an opportunity for upcoming research to define the attributes in a less hypothetical manner, using more realistic therapeutic benefits and adverse events. The resultant preferences may be directly applicable to regulatory and medical situations.
Another implication relates to the ethical issues in dementia research that previous publications have raised, including the exclusion of people living with AD in research because of, for example, difficulties in obtaining their consent to participate (52). Understanding the views and preferences of these people while they maintain the cognitive capabilities to express themselves may provide insights about their preferences in future stages. The similarities in MAR between those living with and without memory problems observed in our study support current practices, where family members or caregivers take medical decisions on behalf of people living with severe cognitive impairment.
Finally, future studies on preferences for AD medication should also explore the effect of other individuals’ characteristics and psychological factors on the MAR, such as prior knowledge and experience with medicines, risk perception, or expected benefits, to better understand which factors influence trade-offs and the heterogeneity in preferences.
Conclusion
Our study shows heterogeneity in MARs for moderate and severe adverse events in order to delay the progression of AD, which is partly explained by individuals’ characteristics and beliefs about medicines. The observed differences in MARs should be taken into account during medical product decision-making since certain subgroups of the population seem willing to accept what might be considered high MARs in order to delay the progression of AD.
Contributors: SRM, STdV, GL, PM, HH, and DP contributed to the formulation of the research question. All authors contributed to the development of the survey. SRM, GL, DP collected the data. SRM and DP analysed the data. All authors contributed to the interpretation of the data. SRM and DP drafted the manuscript. All authors reviewed and edited the manuscript. All authors read and approved the final version of the manuscript. The corresponding author attests that all listed authors meet authorship criteria and that no others meeting the criteria have been omitted.
Funding: This project was funded by Alzheimer’s Research UK (ARUK). The work of SRM is supported by the PROMINENT project, which has received funding from the European Union’s Horizon 2020 research and innovation program under the Marie Skłodowska-Curie grant agreement number 754425.
Ethics approval: The Medical Ethics Review Board of the University Medical Center Groningen (METc UMCG) concluded that an ethical approval was not needed for this study (reference number M21.286183).
Data sharing: The datasets generated or analysed during the current study are available from the corresponding author upon reasonable request.
Disclaimer: The views expressed in this article are the personal views of the authors and may not be understood or quoted as being made on behalf of or reflecting the position of the Dutch Medicines Evaluation Board, the European Medicines Agency, or any of their committees.
Conflict of interest: All authors have completed the ICMJE uniform disclosure form at www.icmje.org/disclosure-of-interest/ and have no conflicts of interest relevant to the contents of this study.
Open Access: This article is distributed under the terms of the Creative Commons Attribution 4.0 International License (http://creativecommons.org/licenses/by/4.0/), which permits use, duplication, adaptation, distribution and reproduction in any medium or format, as long as you give appropriate credit to the original author(s) and the source, provide a link to the Creative Commons license and indicate if changes were made.
References
1. Grossberg GT, Tong G, Burke AD, Tariot PN. Present Algorithms and Future Treatments for Alzheimer’s Disease. J Alzheimers Dis. 2019; 67(4): 1157-1171. DOI: https://doi.org/10.3233/JAD-180903.
2. FDA Aducanumab label BLA 761178. 2020. Available from: https://www.accessdata.fda.gov/drugsatfda_docs/nda/2021/761178Orig1s000TOC.cfm.
3. de la Torre JC, Gonzalez-Lima F. The FDA Approves Aducanumab for Alzheimer’s Disease, Raising Important Scientific Questions. J Alzheimers Dis. 2021; 82(3): 881-882. DOI: https://doi.org/10.3233/JAD-210736.
4. Dhillon S. Aducanumab: First Approval. Drugs. 2021; 81(12): 1437-1443. DOI: https://doi.org/10.1007/s40265-021-01569-z.
5. European Medicines Agency. Withdrawal of application for the marketing authorisation of Aduhelm (aducanumab) EMA/225498/2022; EMEA/H/C/005558. 2022. Available from: https://www.ema.europa.eu/en/documents/medicine-qa/questions-answers-withdrawal-application-marketing-authorisation-aduhelm-aducanumab_en.pdf.
6. de Bekker-Grob EW, Berlin C, Levitan B, et al. Giving Patients’ Preferences a Voice in Medical Treatment Life Cycle: The PREFER Public-Private Project. Patient-Patient Centered Outcomes Research. 2017; 10(3): 263-266. DOI: https://doi.org/10.1007/s40271-017-0222-3.
7. Hauber AB, Johnson FR, Fillit H, et al. Older Americans’ Risk-benefit Preferences for Modifying the Course of Alzheimer Disease. Alz Dis Assoc Dis. 2009; 23(1): 23-32. DOI: https://doi.org/10.1097/WAD.0b013e318181e4c7.
8. Johnson FR, DiSantostefano RL, Yang JC, et al. Something Is Better Than Nothing: The Value of Active Intervention in Stated Preferences for Treatments to Delay Onset of Alzheimer’s Disease Symptoms. Value Health. 2019; 22(9): 1063-1069. DOI: https://doi.org/10.1016/j.jval.2019.03.022.
9. Lepper S, Radke A, Wehrmann H, et al. Preferences of Cognitively Impaired Patients and Patients Living with Dementia: A Systematic Review of Quantitative Patient Preference Studies. J Alzheimers Dis. 2020; 77(2): 885-901. DOI: https://doi.org/10.3233/JAD-191299.
10. Mansfield C, Bullok K, Fuhs JV, et al. The Patient Voice: Exploring Treatment Preferences in Participants with Mild Cognitive Concerns to Inform Regulatory Decision Making. Patient. 2022; 15(5): 551-564. DOI: https://doi.org/10.1007/s40271-022-00576-w.
11. Badia X, Herdman M, Kind P. The influence of ill-health experience on the valuation of health. Pharmacoeconomics. 1998; 13(6): 687-696. DOI: https://doi.org/Doi 10.2165/00019053-199813060-00005.
12. Ludwig K, Ramos-Goni JM, Oppe M, et al. To What Extent Do Patient Preferences Differ From General Population Preferences? Value Health. 2021; 24(9): 1343-1349. DOI: https://doi.org/10.1016/j.jval.2021.02.012.
13. Weernink MGM, van Til JA, Groothuis-Oudshoorn CGM, MJ IJ. Patient and Public Preferences for Treatment Attributes in Parkinson’s Disease. Patient. 2017; 10(6): 763-772. DOI: https://doi.org/10.1007/s40271-017-0247-7.
14. Ogorevc M, Murovec N, Fernandez NB, Rupel VP. Questioning the differences between general public vs. patient based preferences towards EQ-5D-5L defined hypothetical health states. Health Policy. 2019; 123(2): 166-172. DOI: https://doi.org/10.1016/j.healthpol.2017.03.011.
15. Ozdemir S, Ng S, Malhotra C, et al. Patient-Caregiver Treatment Preference Discordance and Its Association With Caregiving Burden and Esteem. Innov Aging. 2021; 5(3). DOI: https://doi.org/10.1093/geroni/igab020.
16. Shin DW, Cho J, Kim SY, et al. Discordance among patient preferences, caregiver preferences, and caregiver predictions of patient preferences regarding disclosure of terminal status and end-of-life choices. Psychooncology. 2015; 24(2): 212-9. DOI: https://doi.org/10.1002/pon.3631.
17. Reamy AM, Kim K, Zarit SH, Whitlatch CJ. Understanding discrepancy in perceptions of values: individuals with mild to moderate dementia and their family caregivers. Gerontologist. 2011; 51(4): 473-83. DOI: https://doi.org/10.1093/geront/gnr010.
18. Russo S, Jongerius C, Faccio F, et al. Understanding Patients’ Preferences: A Systematic Review of Psychological Instruments Used in Patients’ Preference and Decision Studies. Value Health. 2019; 22(4): 491-501. DOI: https://doi.org/10.1016/j.jval.2018.12.007.
19. Andersson Sundell K, Jonsson AK. Beliefs about medicines are strongly associated with medicine-use patterns among the general population. Int J Clin Pract. 2016; 70(3): 277-85. DOI: https://doi.org/10.1111/ijcp.12781.
20. Sjolander M, Eriksson M, Glader EL. The association between patients’ beliefs about medicines and adherence to drug treatment after stroke: a cross-sectional questionnaire survey. BMJ Open. 2013; 3(9): e003551. DOI: https://doi.org/10.1136/bmjopen-2013-003551.
21. Roldan Munoz S, Lupattelli A, de Vries ST, et al. Differences in medication beliefs between pregnant women using medication, or not, for chronic diseases: a cross-sectional, multinational, web-based study. BMJ Open. 2020; 10(2): e034529. DOI: https://doi.org/10.1136/bmjopen-2019-034529.
22. Fillit H, Mohs RC, Edelman Lewis B, et al. A Brief Telephonic Instrument to Screen for Cognitive Impairment in a Managed Care Population. JCOM. 2003; 10(8): 419-429.
23. Horne R, Weinman J, Hankins M. The beliefs about medicines questionnaire: The development and evaluation of a new method for assessing the cognitive representation of medication. Psychology & Health. 1999; 14(1): 1-24. DOI: https://doi.org/10.1080/08870449908407311.
24. Hauber B, Coulter J. Using the Threshold Technique to Elicit Patient Preferences: An Introduction to the Method and an Overview of Existing Empirical Applications. Appl Health Econ Health Policy. 2020; 18(1): 31-46. DOI: https://doi.org/10.1007/s40258-019-00521-3.
25. Hauber AB, Mohamed AF, Johnson FR, et al. Understanding the relative importance of preserving functional abilities in Alzheimer’s disease in the United States and Germany. Qual Life Res. 2014; 23(6): 1813-21. DOI: https://doi.org/10.1007/s11136-013-0620-5.
26. Watson J, Saunders S, Terrera GM, et al. What matters to people with memory problems, healthy volunteers and health and social care professionals in the context of developing treatment to prevent Alzheimer’s dementia? A qualitative study. Health Expect. 2019; 22(3): 504-517. DOI: https://doi.org/10.1111/hex.12876.
27. Guideline on the clinical investigation of medicines for the treatment of Alzheimer’s disease CPMP/EWP/553/95 Rev.2. 2018. Available from: https://www.ema.europa.eu/en/documents/scientific-guideline/guideline-clinical-investigation-medicines-treatment-alzheimers-disease-revision-2_en.pdf.
28. Jongen PJ, Sindic C, Sanders E, et al. Adverse events of interferon beta-1a: a prospective multi-centre international ICH-GCP-based CRO-supported external validation study in daily practice. PLoS One. 2011; 6(10): e26568. DOI: https://doi.org/10.1371/journal.pone.0026568.
29. Merck Sharp & Dohme Corp. asoMC, Inc., A Parallel-Group, Double Blind Long Term Safety Trial of MK-8931 in Subjects with Alzheimer’s Disease. (Protocol No. MK-8931-017-21) (also known as SCH 900931, P07738). 2016, Merck Sharp & Dohme Corp.: Merck Sharp & Dohme Corp. p. 140.
30. Guideline on good pharmacovigilance practices (GVP) – Annex I – Definitions (Rev 4) EMA/876333/2011 Rev 4*. 2017. Available from: https://www.ema.europa.eu/en/documents/scientific-guideline/guideline-good-pharmacovigilance-practices-annex-i-definitions-rev-4_en.pdf.
31. Huys I, de Bekker-Grob E, Levitan B, et al., D2.2 Processes, conditions and contextual factors that influence the utility and role of patient preference studies in PREFER Patient Preferences in Benefit-Risk Assessments during the Drug Life Cycle. 2017.
32. Vermunt L, Sikkes SAM, van den Hout A, et al. Duration of preclinical, prodromal, and dementia stages of Alzheimer’s disease in relation to age, sex, and APOE genotype. Alzheimers Dement. 2019; 15(7): 888-898. DOI: https://doi.org/10.1016/j.jalz.2019.04.001.
33. Klein JP, Moeschbergernd ML, Survival Analyses. 2003, Springer-Verlag New York: Springer-Verlag New York.
34. Mansfield C, Sikirica MV, Pugh A, et al. Patient Preferences for Attributes of Type 2 Diabetes Mellitus Medications in Germany and Spain: An Online Discrete-Choice Experiment Survey. Diabetes Ther. 2017; 8(6): 1365-1378. DOI: https://doi.org/10.1007/s13300-017-0326-8.
35. Roldan Munoz S, Postmus D, de Vries ST, et al. Differences in Importance Attached to Drug Effects Between Patients With Type 2 Diabetes From the Netherlands and Turkey: A Preference Study. Frontiers in Pharmacology. 2021; 11. DOI: https://doi.org/10.3389/fphar.2020.617409.
36. Janssens R, Arnou R, Schoefs E, et al. Key Determinants of Health-Related Quality of Life Among Advanced Lung Cancer Patients: A Qualitative Study in Belgium and Italy. Front Pharmacol. 2021; 12: 710518. DOI: https://doi.org/10.3389/fphar.2021.710518.
37. Bridges JFP, de la Cruz M, Pavilack M, et al. Patient preferences for attributes of tyrosine kinase inhibitor treatments for EGFR mutation-positive non-small-cell lung cancer. Future Oncol. 2019; 15(34): 3895-3907. DOI: https://doi.org/10.2217/fon-2019-0396.
38. Jendle J, Torffvit O, Ridderstrale M, et al. Willingness to pay for health improvements associated with anti-diabetes treatments for people with type 2 diabetes. Curr Med Res Opin. 2010; 26(4): 917-23. DOI: https://doi.org/10.1185/03007991003657867.
39. Edvardsson D, Winblad B, Sandman P. Person-centred care of people with severe Alzheimer’s disease: current status and ways forward. Lancet Neurol. 2008; 7(4): 362-367. DOI: https://doi.org/Doi 10.1016/S1474-4422(08)70063-2.
40. Wehrmann H, Michalowsky B, Lepper S, et al. Priorities and Preferences of People Living with Dementia or Cognitive Impairment – A Systematic Review. Patient Prefer Adherence. 2021; 15: 2793-2807. DOI: https://doi.org/10.2147/PPA.S333923.
41. de Vries ST, de Vries FM, Dekker T, et al. The Role of Patients’ Age on Their Preferences for Choosing Additional Blood Pressure-Lowering Drugs: A Discrete Choice Experiment in Patients with Diabetes. PLoS One. 2015; 10(10): e0139755. DOI: https://doi.org/10.1371/journal.pone.0139755.
42. Harris CR, Jenkins M. Gender Differences in Risk Assessment: Why do Women Take Fewer Risks than Men? Judgment and Decision Making. 2006; 1(1): 48-63.
43. Brown GD, Largey A, McMullan C. The impact of gender on risk perception: Implications for EU member states’ national risk assessment processes. Int J Disast Risk Re. 2021; 63. DOI: https://doi.org/10.1016/j.ijdrr.2021.102452.
44. Smith GE, Chandler M, Fields JA, et al. A Survey of Patient and Partner Outcome and Treatment Preferences in Mild Cognitive Impairment. Journal of Alzheimers Disease. 2018; 63(4): 1459-1468. DOI: https://doi.org/10.3233/Jad-171161.
45. Bradford A, Kunik ME, Schulz P, et al. Missed and Delayed Diagnosis of Dementia in Primary Care Prevalence and Contributing Factors. Alz Dis Assoc Dis. 2009; 23(4): 306-314. DOI: https://doi.org/10.1097/WAD.0b013e3181a6bebc.
46. GPS E. United Kingdom – Overview of the education system (EAG 2022). 2022 [cited 2022 12/10/2022]; Available from: https://gpseducation.oecd.org/CountryProfile?primaryCountry=GBR&treshold=10&topic=EO.
47. Mejia AM, Smith GE, Wicklund M, Armstrong MJ. Shared decision making in mild cognitive impairment. Neurology: Clinical Practice. 2019; 9(2): 160-164. DOI: https://doi.org/10.1212/cpj.0000000000000576.
48. Hauber B, Mange B, Zhou M, et al. Parkinson’s Patients’ Tolerance for Risk and Willingness to Wait for Potential Benefits of Novel Neurostimulation Devices: A Patient-Centered Threshold Technique Study. MDM Policy Pract. 2021; 6(1): 2381468320978407. DOI: https://doi.org/10.1177/2381468320978407.
49. Haeberlein SB, Aisen PS, Barkhof F, et al. Two Randomized Phase 3 Studies of Aducanumab in Early Alzheimer’s Disease. Jpad-J Prev Alzheim. 2022; 9(2): 197-210. DOI: https://doi.org/10.14283/jpad.2022.30.
50. Salloway S, Chalkias S, Barkhof F, et al. Amyloid-Related Imaging Abnormalities in 2 Phase 3 Studies Evaluating Aducanumab in Patients With Early Alzheimer Disease. Jama Neurol. 2022; 79(1): 13-21. DOI: https://doi.org/10.1001/jamaneurol.2021.4161.
51. Mt-Isa S, Ouwens M, Robert V, et al. Structured Benefit-risk assessment: a review of key publications and initiatives on frameworks and methodologies. Pharm Stat. 2016; 15(4): 324-332. DOI: https://doi.org/10.1002/pst.1690.
52. Gotzelmann TG, Strech D, Kahrass H. The full spectrum of ethical issues in dementia research: findings of a systematic qualitative review. BMC Med Ethics. 2021; 22(1): 32. DOI: https://doi.org/10.1186/s12910-020-00572-5.
© The Authors 2023