M.T. Ashford*,1,2, A. Aaronson*,1,3, W. Kwang1,3, J. Eichenbaum1,3, S. Gummadi1,3, C. Jin4, N. Cashdollar5,6, E. Thorp5, E. Wragg5, K.H. Zavitz6, F. Cormack5,7, T. Banh1,3, J.M. Neuhaus4, A. Ulbricht1,3, M.R. Camacho1,2, J. Fockler1,3, D. Flenniken1,2, D. Truran1,2, R.S. Mackin1,8, M.W. Weiner1,3,9,10, R.L. Nosheny1,8
1. VA Advanced Imaging Research Center, San Francisco Veteran’s Administration Medical Center, San Francisco, CA, USA; 2. Northern California Institute for Research and Education (NCIRE), Department of Veterans Affairs Medical Center, San Francisco, CA, USA; 3. University of California, San Francisco Department of Radiology and Biomedical Imaging, San Francisco, CA, USA; 4. University of California San Francisco Department of Epidemiology and Biostatistics San Francisco, CA, USA; 5. Cambridge Cognition, Cambridge, United Kingdom; 6. Cambridge Cognition, Cambridge, MA, USA; 7. Department of Psychiatry, University of Cambridge, Cambridge, United Kingdom; 8. Department of Psychiatry, University of California San Francisco, San Francisco, CA, USA; 9. Department of Neurology, University of California San Francisco, San Francisco, CA, USA; 10. Department of Medicine, University of California San Francisco, San Francisco, CA, USA; *These authors contributed equally
Corresponding Author: Miriam Ashford, 4150 Clement St, San Francisco, CA 94121, Email: Miriam.ashford@ucsf.edu, Phone: +16502089267
J Prev Alz Dis 2024;2(11):514-524
Published online September 25, 2023, http://dx.doi.org/10.14283/jpad.2023.117
Abstract
BACKGROUND: Unsupervised online cognitive assessments have demonstrated promise as an efficient and scalable approach for evaluating cognition in aging, and Alzheimer’s disease and related dementias.
OBJECTIVES: The aim of this study was to evaluate the feasibility, usability, and construct validity of the Paired Associates Learning task from the Cambridge Neuropsychological Test Automated Battery® in adults enrolled in the Brain Health Registry.
DESIGN, SETTING, PARTICIPANTS, MEASUREMENTS: The Paired Associates Learning task was administered to Brain Health Registry participants in a remote, unsupervised, online setting. In this cross-sectional analysis, we 1) evaluated construct validity by analyzing associations between Paired Associates Learning performance and additional participant registry data, including demographics, self- and study partner-reported subjective cognitive change (Everyday Cognition scale), self-reported memory concern, and depressive symptom severity (Patient Health Questionnaire-9) using multivariable linear regression models; 2) determined the predictive value of Paired Associates Learning and other registry variables for identifying participants who self-report Mild Cognitive Impairment by employing multivariable binomial logistic regressions and calculating the area under the receiver operator curve; 3) investigated feasibility by looking at task completion rates and statistically comparing characteristics of task completers and non-completers; and 4) evaluated usability in terms of participant requests for support from BHR related to the assessment.
RESULTS: In terms of construct validity, in participants who took the Paired Associates Learning for the first time (N=14,528), worse performance was associated with being older, being male, lower educational attainment, higher levels of self- and study partner-reported decline, more self-reported memory concerns, greater depressive symptom severity, and self-report of Mild Cognitive Impairment. Paired Associates Learning performance and Brain Health Registry variables together identified those with self-reported Mild Cognitive Impairment with moderate accuracy (areas under the curve: 0.66-0.68). In terms of feasibility, in a sub-sample of 29,176 participants who had the opportunity to complete Paired Associates Learning for the first time in the registry, 14,417 started the task. 11,647 (80.9% of those who started) completed the task. Compared to those who did not complete the task at their first opportunity, those who completed were older, had more years of education, more likely to self-identify as White, less likely to self-identify as Latino, less likely to have a subjective memory concern, and more likely to report a family history of Alzheimer’s disease. In terms of usability, out of 8,395 received requests for support from BHR staff via email, 4.4% (n=374) were related to PAL. Of those, 82% were related to technical difficulties.
CONCLUSIONS: Our findings support moderate feasibility, good usability, and construct validity of cross-sectional Paired Associates Learning in an unsupervised online registry, but also highlight the need to make the assessment more inclusive and accessible to individuals from ethnoculturally and socioeconomically diverse communities. A future, improved version could be a scalable, efficient method to assess cognition in many different settings, including clinical trials, observational studies, healthcare, and public health.
Key words: Brain health registry, unsupervised cognitive assessment, Paired Associates Learning, CANTAB, Mild Cognitive Impairment.
Introduction
Digital, unsupervised cognitive assessments demonstrate promise for efficiently evaluating cognition in brain diseases related to aging, including Alzheimer’s disease and related dementias. This approach may improve at-risk individuals’ access to care and research studies, minimize the time and costs involved in research participation, and enable more frequent assessment of diverse populations (1). These assessments have been particularly useful during the COVID-19 pandemic (2). Furthermore, there is evidence for the validity of unsupervised remote cognitive assessments in comparison with traditional supervised in-clinic assessments and Alzheimer’s disease and related dementias-related biomarkers (1). However, numerous challenges remain, for example, external factors (e.g., assessment environment, device, internet connection) could impact the quality of the data. Furthermore, lack of an assessor may impact participants’ understanding of the assessment, motivation, and engagement (3, 4).
The Paired Associates Learning (PAL) task from the Cambridge Neuropsychological Test Automated Battery (CANTAB®; Cambridge Cognition, 2022) is a digital cognitive assessment (5). The CANTAB PAL assesses visual learning and episodic memory. Previous studies have shown that those with Mild Cognitive Impairment and mild Alzheimer’s disease dementia performed more poorly on PAL than cognitively unimpaired individuals (6-10). Furthermore, in combination with other measures, PAL performance accurately predicts progression to dementia (6, 7, 11) and is associated with Alzheimer’s disease biomarkers (10, 12-14). PAL has also demonstrated comparable levels of performance and psychometric properties when administered in a remote, unsupervised setting on an individual’s personal computer at home when compared to an in- clinic, supervised environment (15-17) In March 2021, the PAL task was added to the Brain Health Registry (BHR), an online, remote research study run by the University of California, San Francisco ( N>100,000) that collects longitudinal, unsupervised data related to cognitive aging and dementias in adults (18).
The overall aim of this study was to evaluate the feasibility, usability, and construct validity of an online, unsupervised, at home version of the PAL task in Brain Health Registry participants. To assess construct validity of PAL, this analysis investigated cross-sectional association of PAL performance with BHR participant data, as well as the predictive value of PAL in identifying BHR participants who self-report Mild Cognitive Impairment. This analysis focused on Mild Cognitive Impairment since there is a critical, unmet need to identify adults for clinical treatment research at early stages on the disease spectrum (19). To assess feasibility, we examined PAL task completion rate, and differences between PAL completers and non-completers, and to assess usability, we summarized requests for BHR staff support regarding PAL and determined the proportion of participants passing the integrity criteria. We hypothesized that a decrease in PAL performance would be associated with older age, fewer years of education, self-reported memory concerns, a family history of Alzheimer’s disease, as well as with higher levels of subjective cognitive decline and depressive symptom severity.
Methods
Study design
This study reports cross-sectional CANTAB PAL data collected through the BHR study, an ongoing longitudinal study which collects data in an online unsupervised setting. BHR has over 100,000 consented and enrolled participants over the age of 18 who are invited to complete online assessments every six months, including self-report questionnaires (e.g., sociodemographic and health information) and different cognitive assessments (18). Participation in BHR is voluntary and not compensated. The BHR study is approved by the University of California, San Francisco institutional review board.
Measures
Paired Associates Learning assessment
In the CANTAB PAL task (Cambridge Cognition, 2022; cambridgecognition.com/cantab/), participants receive automated voiceover instructions to remember the location of abstract colorful patterns which are presented within a variety of possible locations on a computer screen (see Figure 1A). In BHR, PAL is called the “Pattern Location Challenge,” and is presented as the third task in the second section (labeled “Identifying Changes”) of the BHR task list (see Figure 1B for a screenshot). In the BHR version of PAL, registry participants have the opportunity to complete up to 5 stages of the assessment, which include learning two, four, six, eight or twelve pattern-location pairings. During the first part of the assessment, BHR participants are shown boxes, which are “opened” in a randomized order one-by-one, displaying either a pattern or an empty box which the participant needs to remember. Next, the patterns are displayed in the middle of the screen one at a time and the participant needs to indicate the box in which the pattern was located originally. In case of an error, the boxes re-open and the participant can try again to recall the correct pattern locations, up to a maximum of 4 attempts. From any given stage, a subject can advance to the next more difficult stage only if they correctly recall all of the patterns. If the participant fails to complete the stage after 4 attempts, then the task will end.
For the present analysis, the outcome variables were a) PAL First Attempt Memory Score and b) PAL Total Errors Adjusted. PAL First Attempt Memory Score is the number of times a participant chose the correct box on their first attempt when recalling the pattern locations. PAL Total Errors Adjusted accounts for adjusted errors for all 2-12 pattern levels regardless of whether the participant reached the 12-box level or not. These two PAL performance measures were chosen as they are relatively independent from one another and are also most commonly reported when using PAL (5). This analysis data collected between March 2021 and July 2022, focused on the first time PAL was taken to eliminate practice effects, and included participants with at least one completed PAL assessment and those who successfully completed the easiest stage of the PAL task (N=14,528).
Self-reported measures
Participants enrolled in BHR are invited to complete a variety of online self-report questionnaires every six months. For this analysis, the self-report measure data closest to the first PAL assessment was included.
Self-reported sociodemographic measures
For this analysis, we included information from the following participant characteristics: age at the time of assessment (continuous), gender (male, female, other, prefer not to say), educational attainment (grammar school, high school, some college, two-year degree, four-year degree, master’s degree, doctoral degree, professional degree), ethnicity (Latino, non-Latino, declined to state), and race (African American/ Black, Asian, Native American, Other, Pacific Islander, White decline to state). We converted the variable educational attainment into a continuous years of education measure.
Self-reported and medical history and memory measures
This analysis used subjective memory concern (“Are you concerned that you have a memory problem?”; yes, no, prefer not to say), family history of Alzheimer’s disease (“Do you have any biological parents, full siblings, or biological children who have been diagnosed with Alzheimer’s Disease?”; yes, no, I don’t know, prefer not to say), self-reported Mild Cognitive Impairment (“Please indicate whether you currently have or have had any of the following conditions in the past: Mild Cognitive Impairment”; yes, no, I don’t know, prefer not to say, and self-reported Alzheimer’s disease (“Please indicate whether you currently have or have had any of the following conditions in the past: Alzheimer’s disease”; yes, no, I don’t know, prefer not to say).
Everyday Cognition Scale
The 39-item Everyday Cognition Scale (ECog) measures change in instrumental activities of daily living compared to activity levels 10 years before as rated by the participant (self-rated) and the study partner (20). Activities included in the Everyday Cognition Scale map onto six domains of cognitive abilities. Scores range from 1-4. Higher scores indicate more reported decline. An online adaptation of the scale is used in the BHR (21).
PHQ-9
The Patient Health Questionnaire-9 (PHQ-9) is a brief (9 item), valid, and reliable self-administered questionnaire, which measures depressive symptom severity (22, 23). In the questionnaire, participants respond to each of the nine DSM-IV criteria on a scale ranging from not at all (0) – nearly every day (3). Higher scores indicate greater depressive symptom severity (normal range 0-27).
Feasibility and Usability Outcomes
We defined feasibility in terms of participant completion of the PAL task and usability in terms of participant requests for support related to PAL.
Feasibility – PAL completion
To assess feasibility, we looked at a subset of participants (N=29,176) at their first opportunity to complete the PAL task in BHR. An opportunity is defined as a participant logging into BHR and completing the first, required self-report questionnaire. After completing the required questionnaire, the PAL task appears in the participant’s task list. For this analysis we report on how many participants of this subset (i) did not attempt/start the PAL task; (ii) attempted but did not complete; (iii) attempted and completed. For those who attempted PAL but did not complete we are reporting reasons for lack of completion.
Usability
This study assesses usability by (1) participant contact with the BHR staff and (2) by determining the proportion of participants passing the integrity criteria (>2 patterns). Participants can contact BHR staff for support via email and an online web form. BHR staff manage participant support requests using Zendesk, a third-party customer support tool that uses an e-mail/message ticketing system to communicate with participants. Tickets are solved by designated BHR staff within 24-48 hours. For the present analysis, we examined total number of tickets received and sorted these tickets by general category.
Statistical analyses
The objectives of this statistical analysis was to 1) describe and compare demographic characteristics of BHR participants who took the PAL task and those who did not, 2) report descriptive statistics of PAL performance measures for PAL First Attempt Memory Score and PAL Total Errors Adjusted, 3) estimate magnitude of associations between participant reported information (age, gender, ethnicity, race, years of education, self- and study partner reported Everyday Cognition Scale scores, subjective memory concern, self-reported diagnosis of Mild Cognitive Impairment and Alzheimer’s disease, family history of Alzheimer’s disease, and Patient Health Questionnaire-9 score) and the two PAL performance outcome measures, and 4) assess the predictive value of the two PAL performance measures in distinguishing adults with or without self-report of Mild Cognitive Impairment.
We calculated descriptive statistics including frequencies and percentages for categorical data and mean and standard deviation (SD) for continuous data to assess participant information and PAL performance measures. For evaluating differences between those who completed PAL at their first opportunity and those who did not, we used Welch two sample t-test for continuous variables and Chi-square test for categorical variables, and calculated 95% confidence intervals (CI) and effect sizes (Cohen’s d for continuous variables; Cohen’s h for categorical variables). For assessing the associations between PAL and BHR variables, we employed multivariable linear regression models. Using ordinary least squares, we fit separate models for each of the two PAL performance measures and included the following predictors in each model: age, gender, education, self-Everyday Cognition Scale, study partner Everyday Cognition, subjective memory concern, self-reported Mild Cognitive Impairment, self-reported family history of Alzheimer’s disease, Patient Health Questionnaire-9. For the linear regressions we report regression coefficients and 95% confidence intervals (CI) for the models. We used multivariable binomial logistic regressions to assess the ability of PAL (predictor) to distinguish participants who self-reported Mild Cognitive Impairment from those who did not (outcome). We modeled the predictors PAL First Attempt Memory Score and PAL Total Errors Adjusted separately and in combination. Each predictor was modeled by itself and in combination with the following covariates: age, gender, and education. We assessed the predictive performance of the logistic model by calculating the area under the receiver operating characteristic curve. We calculated 10-fold cross-validated estimates of the AUCs to correct for optimism. We used SAS 9.4 (SAS Institute, Cary NC) and R (version 4.2.2) (24) for the statistical analyses.
Results
Sample characteristics of BHR first time PAL takers
The study sample consisted of 14,528 participants who completed the PAL for the first time with scores meeting the integrity criteria. Table 1 presents a summary of participant characteristics including sociodemographic and health information. Participants who completed PAL were on average 66.3 years old (SD=11.3), had an average of 16.6 years of education (SD=2.27), 10,526 (72.5%) identified as female, 13,487 (92.8%) as White and 922 (6.3%) as Latino. In terms of self-reported health- and cognition-related variables, 496 (3.4%) self-reported a Mild Cognitive Impairment diagnosis, and 54 (0.4%) self-reported an Alzheimer’s disease diagnosis. 6,162 (42.4%) had a subjective memory concern, and 5,038 (34.7%) had a family history of Alzheimer’s disease.
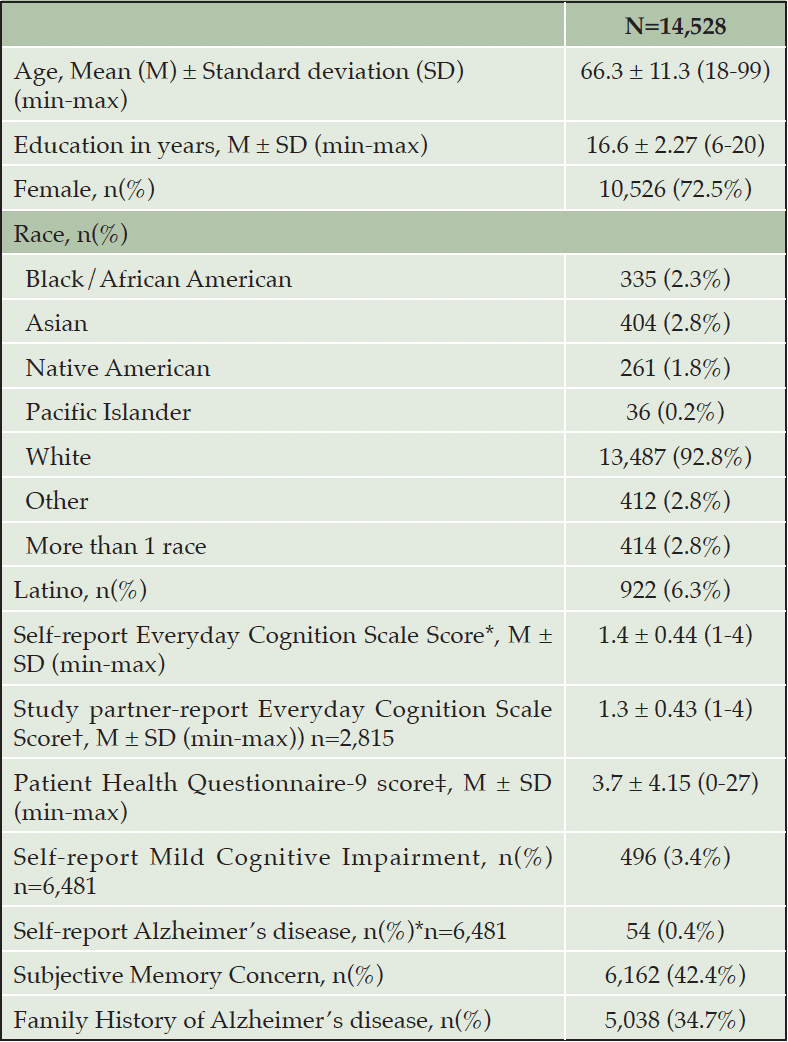
Table 1. Descriptive statistics of participant self-report variables who completed Paired Associates Learning (PAL) for the first time
Note. *Self-report Everyday Cognition Scale normal range: 1-4; †Study partner-report ECog normal range: 1-4; ‡Patient Health Questionnaire (PHQ-9) normal range: 0-27
PAL performance scores
The mean PAL First Attempt Memory Score was 12.1 (SD=4.25, min=0, max=20) and the mean PAL Total Errors Adjusted was 41.8 (SD=29.5, min=0, max=111). Figure 1C shows the distribution of the two PAL performance outcomes.
Note. A) During the first part of the PAL assessment, participants are shown boxes configured in a circle (A1). In the following part, the boxes are “opened” in a randomized order one-by-one, displaying either a pattern or an empty box which the participant needs to remember (A2). Next, the patterns are displayed in the middle of the screen one at a time and the participant needs to indicate the box in which the pattern was located originally (A3). B) Screenshot of the BHR task list showing the location of PAL named “Pattern Location Challenge”. C) PAL First Attempt Memory Score and PAL Total Errors Adjusted distributions. PAL First Attempt Memory Score is the number of times a participant chose the correct box on their first attempt when recalling the pattern locations. PAL Total Errors Adjusted accounts for adjusted errors for all 2-12 pattern levels regardless of whether the participant reached the 12-box level or not.
PAL construct validity – Associations between participant information and PAL performance scores
Lower PAL First Attempt Memory Score (worse) performance was associated with being older, having fewer years of education, identifying as male, higher self- and study partner reported Everyday Cognition Scale scores, self-reporting a memory concern, self-report of Mild Cognitive Impairment, a family history of Alzheimer’s disease, and higher depressive symptom severity (see Table 2). Specifically, a 1-year increase in age is significantly associated with a -0.14 decrease in PAL First Attempt Memory Score (PAL FAMS). A 1-year increase in years education is significantly associated with a .12 increase in PAL FAMS. Compared to male participants, female participants have a .81 increase in PAL FAMS. A 1-unit increase in Self-report Everyday Cognition score is significantly associated with a -.96 decrease in PAL FAMS. A 1-unit increase in Study Partner-report Everyday Cognition score is significantly associated with a -1.40 decrease in PAL FAMS. Having a subjective memory concern is significantly associated with a -.68 decrease in PAL FAMS. Self-reported Mild Cognitive Impairment is significantly associated with a -1.74 decrease in PAL FAMS. A 1-unit increase in Patient Health Questionaire-9 score is significantly associated with a -.06 decrease in PAL FAMS.
Higher PAL Total Errors Adjusted reflecting worse performance was also associated with being older, having less years of education, identifying as male, higher self- and study partner reported Everyday Cognition Scale scores, self-reporting a memory concern, self-report of Mild Cognitive Impairment, a family history of Alzheimer’s disease, and higher depressive symptom severity. Specifically, a 1-year increase in age is significantly associated with a 1.02 increase in PAL total errors. A 1-year increase in years of education is significantly associated with a -0.88 decrease in PAL total errors. Compared to male participants, female participants significantly have 7.14 fewer PAL total errors. A 1-unit increase in Self-report Everyday Cognition score is significantly associated with a 6.37 increase in PAL total errors. A 1-unit increase in Study Partner-report Everyday Cognition score is significantly associated with a 9.08 increase in PAL total errors. Having a subjective memory concern is significantly associated with 4.64 more PAL total errors. Self-reported MCI is significantly associated with a 12.04 increase in PAL Total Errors. A 1-unit increase in Patient Health Questionaire-9 score is significantly associated with a .39 increase in PAL Total Errors.
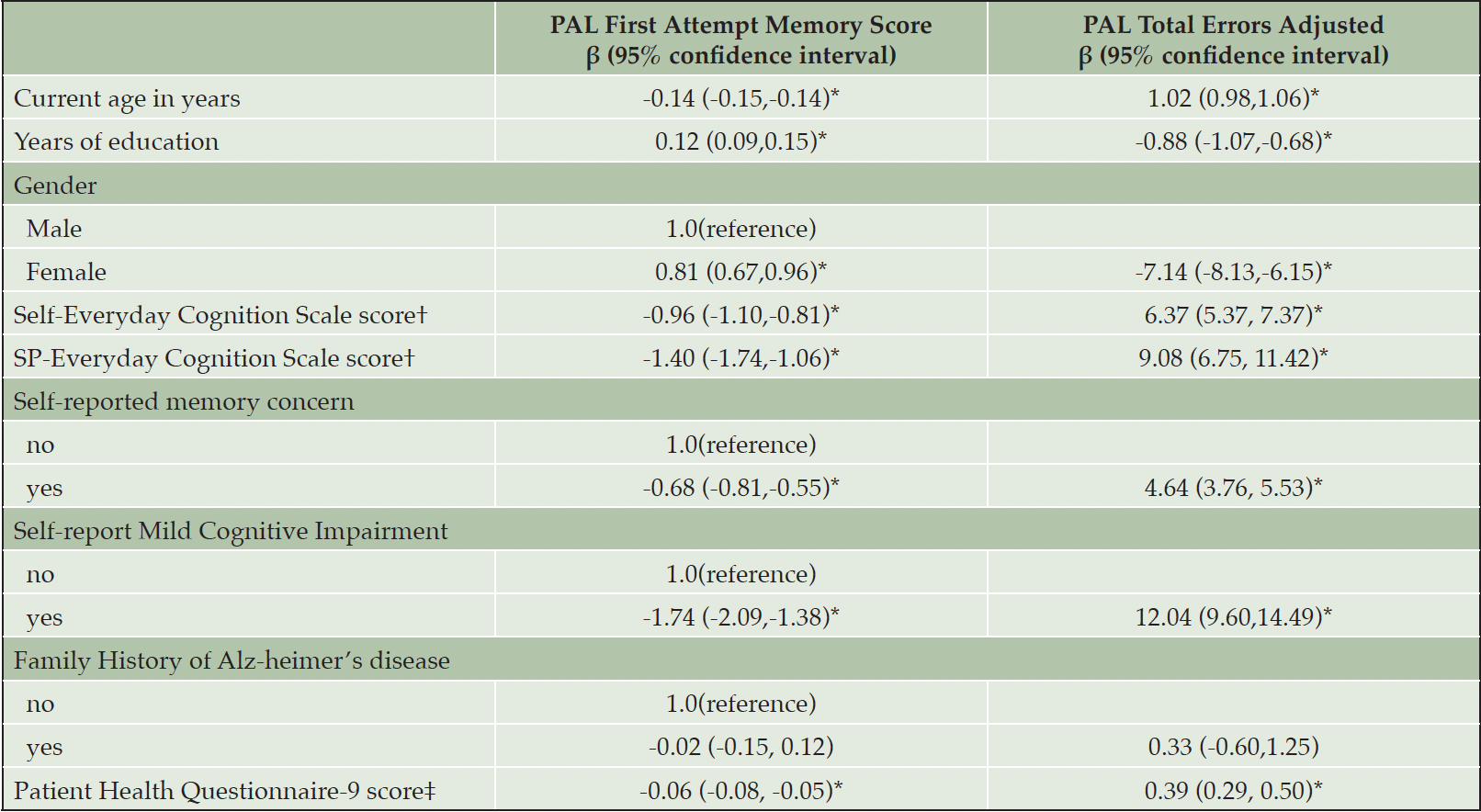
Table 2. Estimated regression coefficients and 95% confidence intervals from linear regression models fit to PAL First Attempt Memory Score and PAL Total Errors Adjusted outcomes
Note. *<.05, †Everyday Cognition Scale normal range: 1-4; ‡Patient Health Questionnaire (PHQ-9) normal range: 0-27
PAL performance measures to predict self-reported Mild Cognitive Impairment in BHR participants
PAL First Attempt Memory Score predicted self-reported Mild Cognitive Impairment with a cross-validated area under the curve of 0.66. Adding demographic information (age, gender, education) slightly improved the cross-validated area under the curve to 0.68. PAL Total Errors Adjusted scores predicted self-reported Mild Cognitive Impairment with a cross-validated area under the curve of 0.67. Adding demographic information to PAL Total Errors Adjusted slightly increased the cross-validated area under the curve to 0.68. PAL First Attempt Memory Score and PAL Total Errors Adjusted combined predicted self-reported Mild Cognitive Impairment with a cross-validated area under the curve of 0.67. Adding demographic information to this model slightly increased the cross-validated area under the curve to 0.68. See Figure 2 for a summary of multivariate logistic regressions and receiver operating characteristic curves.
Feasibility – PAL completion
Finally, we looked at a subset of participants who had their first opportunity to complete PAL (defined as being presented with PAL in their BHR task list; N=29,176). Of those, 14,759 (50.6%) did not attempt PAL and 14,417 (49.4%) attempted (started) the PAL task. Of those who attempted, 2,770 (19.2%) did not complete PAL and 11,647 (80.8%) completed PAL. When comparing those who completed PAL (N=11,647) to those who either did not attempt or attempted but did not complete; N=17,529, there were statistically significant differences in self-reported age, gender, education, race, memory concern, and family history of Alzheimer’s disease (see Table 3). BHR participants who completed PAL (N=11,647) were older with more years of education, less likely to report female gender, less likely to self-report identifying with being Black/African American, Native American, Pacific Islander, and Latino ethnicity, and more likely to be White, or Asian, less likely to report a subjective memory concern, and more likely to report a family history of Alzheimer’s disease. Participants who attempted but did not complete PAL (N=2,770) were on average 64.1 years old (SD=11.2), had an average of 15.5 years of education (SD=2.5), 76.3% (N=2,114) identified as female, 92.8% (N=2,425) as White and 488 (17.6%) as Latino. A total of 1429 (51.6%) self-reported a memory concern, and 912 (32.9%) had a family history of Alzheimer’s disease. The two most common reasons for starting but not completing PAL were that the device was not supported (N=2,071) followed by technical difficulties (N=468).
PAL usability – participant support
Out of 8,395 received requests for support from BHR staff via email during the specified time period, 4.4% (n=374) were related to PAL. These emails fell under 3 different themes: technical difficulties (i.e., difficulty loading the test, incompatible device (n=305, 81.6%)), user experience (i.e., confusion with study instructions, issues with audio) (n=65, 17.4%)), and participant feedback/suggestions for improvement (n=4, <1%). Out of 14,650 participants who completed PAL for the first time, only 32 (0.2%) did not pass the integrity criteria.
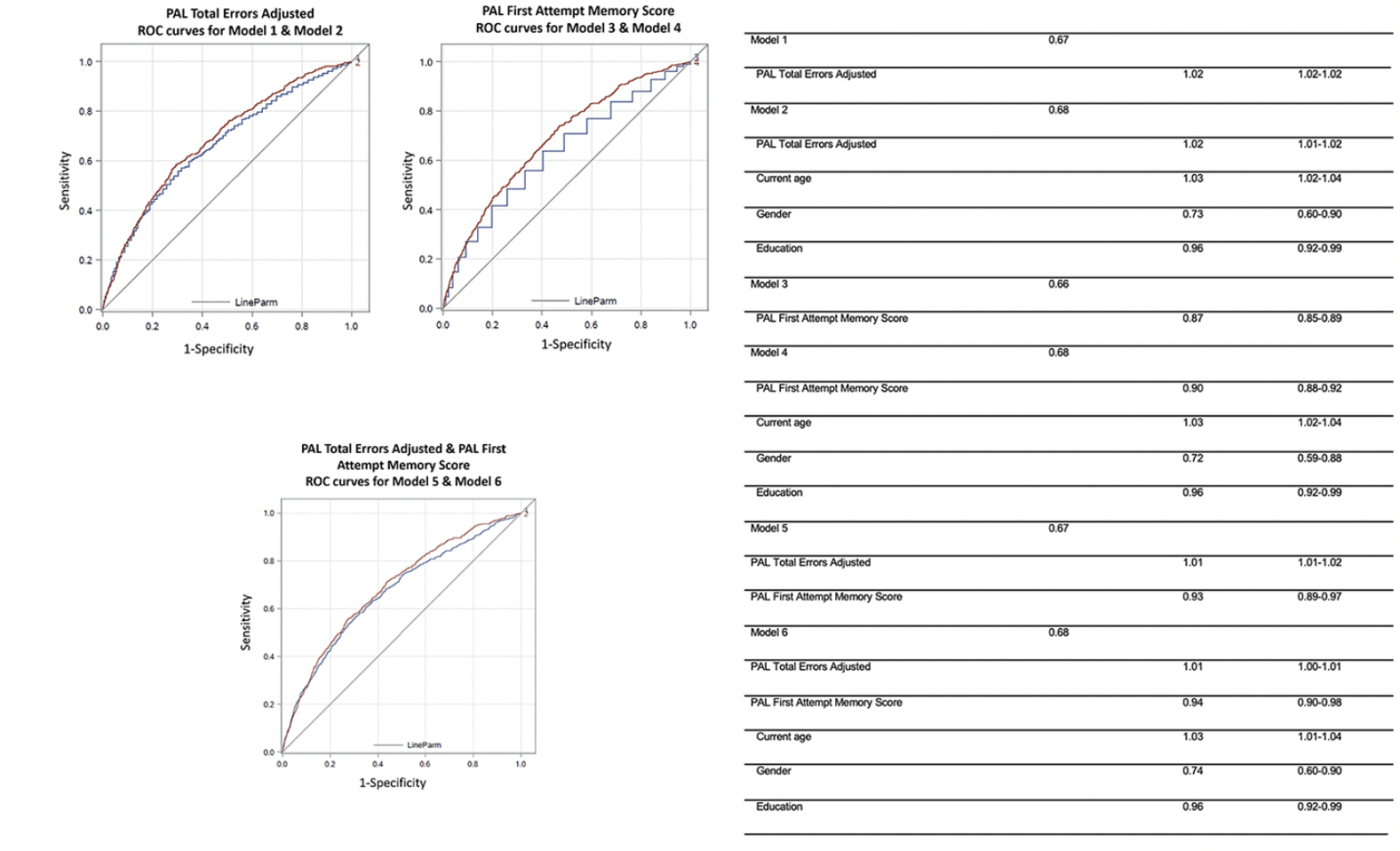
Figure 2. Predictive performance of multivariable logistic regression models to distinguish participants who self-report Mild Cognitive Impairment from those who do not. Areas under receiver operating characteristic curves (ROC) for predictive ability of Paired Associates Learning (PAL) First Attempt Memory Score and PAL
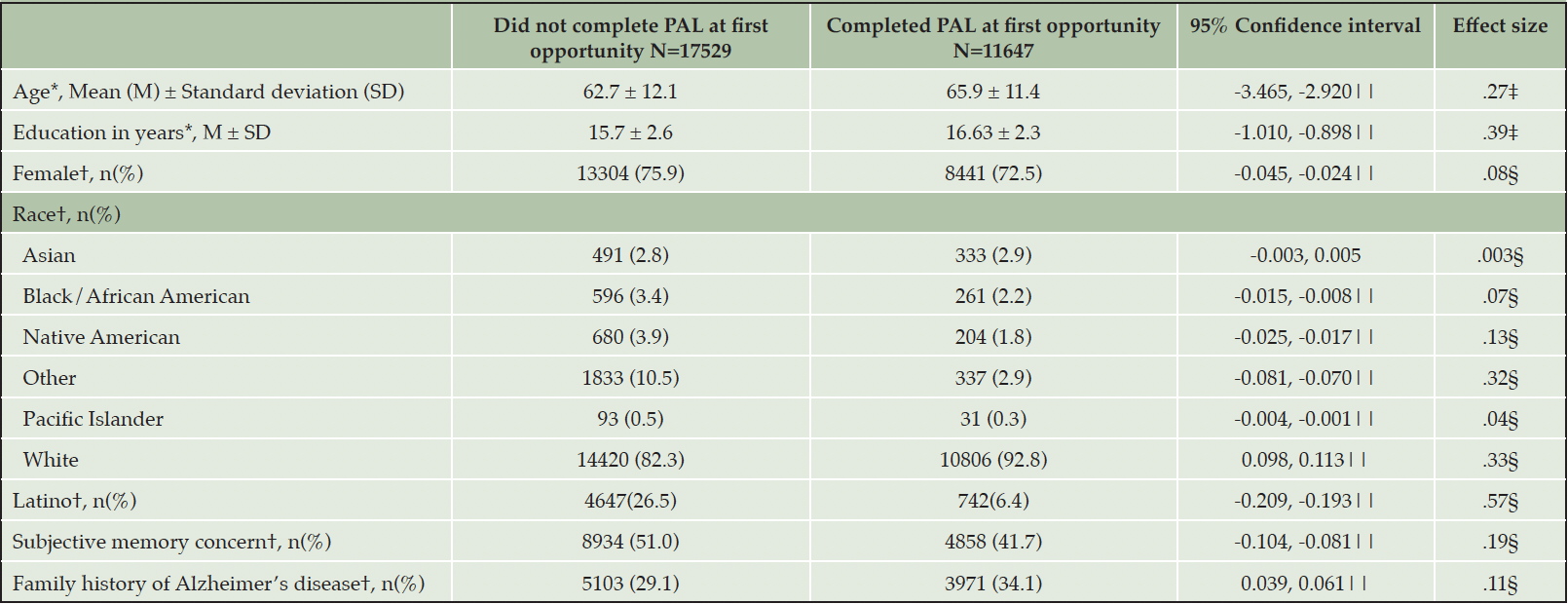
Table 3. Participant characteristics of those who completed the Paired Associates Learning (PAL) task at their first opportunity compared to those who did not
Note. * Welch two sample t-test; †=Chi-square test; ‡= Cohen’s d; §= Cohen’s h; ||= p<.05
Discussion
The major findings were that (1) PAL has good usability, but only moderate feasibility, and lacks accessibility for diverse ethnocultural an socioeconomic communities (2) associations of PAL with demographic and cognitive BHR variables support construct validity and (3) PAL performance differentiates participants with a self-reported diagnosis of Mild Cognitive Impairment and participants who do not report a diagnosis of Mild Cognitive Impairment with modest accuracy. Online, unsupervised cognitive assessments like PAL hold promise as an efficient and scalable approach for evaluating cognition related to aging and brain health, including Alzheimer’s disease and related dementias. This may improve access to care and research studies.
This first major finding was that our results demonstrate good usability of the PAL task in a large sample of >11,000 participants who took the task in an unsupervised online setting. For those who completed the task, there was minimal need for support and only 0.2% of PAL completed tests did not meet the necessary integrity criteria. Only 4.5% (375/8,395) of all requests for support in BHR during the same time period were about PAL. In comparison, during the same time period, BHR staff received 8,395 support requests for the overall BHR study and of those, there was a total 868 (10.3%) requests for other cognitive assessments in BHR. The most common reason for contacting BHR with a request for support with PAL was related to unsupported devices. Currently, PAL in BHR does not support the use of smartphones and tablet. This raises feasibility and accessibility concerns, especially since, for example, Black/African American and Latino adults are less likely to own a traditional computer compared to White adults (25).
In terms of feasibility, a moderate percentage of participants completed the PAL assessment when the task was first added to their task list (39%) and 19% or approximately 1 out of 5 attempted the PAL task but did not complete it. It is possible that the order of the PAL task within the BHR task list, as well as task choice overload may have affected completion of the PAL task. Future research could include an analysis of participants’ overall BHR task usage to identify whether certain participants actively avoid the PAL task. In addition, since PAL is administered in an unsupervised setting, completion might be affected by external factors such as internet connection and distractions in the participant’s environment.
Participants who were older, had more years of education, male, self-identified as White, non-Latino, had fewer memory concerns, and who were more likely to self-report a family history of Alzheimer’s disease had a higher probability of completing PAL. This is in line with results from a previous analysis of BHR task completion data which highlighted the registry’s failure to engage non-White and non-Latino participants in terms of completion of cognitive assessments (26). In addition, in comparison with a recent analysis of the BHR participant cohort (n=90,650), those who completed PAL had a higher percentage of individuals identifying as White (92.8% versus 79.1%) and a lower percentage identifying as Latino (6.3% versus 13.2%). The PAL completer group had a lower percentage of individuals with a subjective memory concern (42.4% versus 49.9%) and higher percentage of individuals with a family history of Alzheimer’s disease (34.7% versus 22.3%). These selection biases are important to consider when evaluating the generalizability of the results.
The second major finding was that mean PAL scores were associated with age, gender, education, self-report of memory concerns, self-report of Mild Cognitive Impairment, depressive symptoms severity, but not family history of Alzheimer’s disease. These findings provide evidence supporting the construct validity of PAL in an online unsupervised setting. The data showed associations of decreasing PAL performance with increasing age, decreasing educational attainment, increasing self- and study partner reported cognitive change (Everyday Cognition Scale), a self-reported memory concern, increasing depressive symptoms severity, as well as with a self-reported diagnosis of Mild Cognitive Impairment. These findings are consistent with previous studies of cognition in online unsupervised settings (21, 27-29) and of PAL (5, 12). Our data also revealed that male gender was associated with decreasing PAL performance on both measures, but there was a larger effect for PAL first attempt memory score. Previous findings from analyses of PAL have been mixed in terms of gender effects, with some research showing gender differences in a general population of older men and women (30), which aligns with the results reported here. Other research reports no sex difference in a large sample looking at normative performance (31). Also, in contrast, previous research has reported that older male participants showed higher performance for visual memory tests (32). One potential factor influencing our findings might be a female self-selection bias (73% of PAL completers were female). In addition, the effects of education, as well as social and mental activity may play a role in the identified gender difference. This is an area which requires further investigation. We also found no association of family history of Alzheimer’s disease with either of the PAL performance outcomes. This finding stands in contrast to previous studies which have identified relationships between family history of Alzheimer’s disease and poorer cognitive assessment performance in older adults (33). However, our finding is consistent with a study evaluating a different unsupervised online cognitive assessment in BHR (27, 28).
The third major finding was that PAL performance measures, with and without demographic information, identified those with self-reported Mild Cognitive Impairment with moderate accuracy (area under the curve: 0.66-0.68 in Figure 2). This is in line with a study of PAL in a supervised setting, which showed high sensitivity and specificity in differentiating between participants with Mild Cognitive Impairment and cognitively unimpaired participants (5, 34) and comparable to other computerized cognitive assessments (35). Even though the accuracy is considered low to moderate these results suggest that PAL may be useful as a highly scalable first step in a multi-stage screening process, to identify those at higher risk for Mild Cognitive Impairment for subsequent screening. It is necessary to further improve the ability of unsupervised remote cognitive assessments, such as PAL, so they may accurately detect Mild Cognitive Impairment. Future strategies could include testing the assessments in cohorts with clinically confirmed Mild Cognitive Impairment, longitudinal testing to establish the stability and or/progression of the observed impairment, as well as analysis over multiple assessments across domains. It should also be noted that the Mild Cognitive Impairment diagnosis used in this study was self-reported, rather than clinically confirmed, and that participants may not understand what it means to be told that they have Mild Cognitive Impairment. Nonetheless, this is a highly scalable approach which could be extended to many research settings and clinical care, such as facilitating clinical trial and observational study screening and assessment.
This study is not without limitations. Due to the overall design of the BHR, this study is subject to multiple selection biases. BHR is a voluntary registry which requires access to the internet and a computer, high literacy, and motivation to enroll and complete the BHR tasks. In addition, internet and a computer literacy and proficiency are not measured in this study, but this would be important information to collect in future studies. Further, only recently (July 2021) did BHR become available in Spanish, so the vast majority of participants are English-speaking. Like other studies, BHR underrepresents participants from the Latino, Asian, Black, other non-White communities, and male participants, as well as those with fewer than 16 years of education. These selection biases were amplified when considering only those who completed PAL. This impacts the interpretation and generalizability of the presented findings. Therefore, BHR PAL should be made accessible to individuals from ethnoculturally and socioeconomically diverse communities. Further, PAL usability was only assessed via digital support requests, which relies on the technology proficiency and cognitive ability of participants, and by determining the number and proportion of participants whose scores did not meet data integrity criteria. Future studies could include a formal assessment of usability, for example, using a validated usability scale like the System Usability Scale (36) and/or in-depth interviews with both participants who complete and not complete PAL. In addition, the support request data was not linked to participants’ demographic data, which would be informative for future studies to better understand reasons for non-completion. Lastly, this analysis focused on cross-sectional data of BHR participants who took PAL for the first time. Future analyses will investigate longitudinal PAL performance in BHR and expand to look at the associations with in-clinic assessments and biomarkers.
Taken together our findings show that collecting PAL data from a large sample (n>11,000) in an online unsupervised setting shows good usability and moderate feasibility. The minimal need for support provides evidence for the usability of PAL in this format. The findings also provide evidence about the construct validity of cross-sectional BHR PAL and that BHR PAL may facilitate efficient screening of older adults for Mild Cognitive Impairment. However, our findings also highlight the need to make this version of PAL more inclusive and accessible to individuals from diverse ethnocultural and socioeconomic communities. Unsupervised, online administration of PAL is a potentially highly scalable approach that could facilitate clinical research and clinical care in many settings, especially if proven to be accessible and inclusive.
Acknowledgments: Our deep gratitude to all BHR study participants and study partners, Cambridge Cognition, as well as current and former BHR staff who expertly run the registry.
Funding: The sponsors had no role in the design and conduct of the study; in the collection, analysis, and interpretation of data; in the preparation of the manuscript; or in the review or approval of the manuscript.
Conflict of interest disclosure: Anna Aaronson, Winnie Kwang, Joseph Eichenbaum, Shilpa Gummadi, Chengshi Jin, Timothy Banh, Aaron Ulbricht, Monica R. Camacho, Juliet Fockler, Derek Flenniken, Nathan Cashdollar, Emily Thorp, Elizabeth Wragg, Francesca Cormack, Kenton H. Zavitz, and Diana Truran report no conflict of interest. Miriam T. Ashford is supported by the National Institutes of Health’s National Institute on Aging, grant F32AG072730-01. This content is solely the responsibility of the authors and does not necessarily represent the official views of the National Institutes of Health’s National Institute on Aging. John Neuhaus reports grants from NIH, during the conduct of the study. R. Scott Mackin reports grants from National Institute of Mental Health, grants from Janssen Research and Development LLC, grants from National Institute of Aging, outside the submitted work. Michael W. Weiner reports grants from National Institutes of Health (NIH), grants from Department of Defense (DOD), grants from Patient-Centered Outcomes Research Institute (PCORI), grants from California Department of Public Health (CDPH), grants from University of Michigan, grants from Siemens, grants from Biogen, grants from Hillblom Foundation, grants from Alzheimer’s Association, grants from The State of California, grants from Johnson & Johnson, grants from Kevin and Connie Shanahan, grants from GE, grants from VUmc, grants from Australian Catholic University (HBI-BHR), grants from The Stroke Foundation, grants from Veterans Administration, personal fees from Acumen Pharmaceutical, personal fees from Cerecin, personal fees from Dolby Family Ventures, personal fees from Eli Lilly, personal fees from Merck Sharp & Dohme Corp., personal fees from National Institute on Aging (NIA), personal fees from Nestle/Nestec, personal fees from PCORI/PPRN, personal fees from Roche, personal fees from University of Southern California (USC), personal fees from NervGen, personal fees from Baird Equity Capital, personal fees from BioClinica, personal fees from Cytox, personal fees from Duke University, personal fees from Eisai, personal fees from FUJIFILM-Toyama Chemical (Japan), personal fees from Garfield Weston, personal fees from Genentech, personal fees from Guidepoint Global, personal fees from Indiana University, personal fees from Japanese Organization for Medical Device Development, Inc. (JOMDD), personal fees from Medscape, personal fees from Peerview Internal Medicine, personal fees from Roche, personal fees from T3D Therapeutics, personal fees from WebMD, personal fees from Vida Ventures, personal fees from The Buck Institute for Research on Aging, personal fees from China Association for Alzheimer’s Disease (CAAD), personal fees from Japan Society for Dementia Research, personal fees from Korean Dementia Society, outside the submitted work; and I hold stocks or options with Alzheon Inc., Alzeca, and Anven. Rachel Nosheny reports grants from NIH, grants from Genentech, Inc., and grants from California Department of Public Health outside the submitted work.
Ethical standards: The UCSF Brain Health Registry is approved by the UCSF Institutional Review Board.
Open Access: This article is distributed under the terms of the Creative Commons Attribution 4.0 International License (http://creativecommons.org/licenses/by/4.0/), which permits use, duplication, adaptation, distribution and reproduction in any medium or format, as long as you give appropriate credit to the original author(s) and the source, provide a link to the Creative Commons license and indicate if changes were made.
References
1. Öhman, F., et al., Current advances in digital cognitive assessment for preclinical Alzheimer’s disease. Alzheimer’s & Dementia: Diagnosis, Assessment & Disease Monitoring, 2021. 13(1): p. e12217 DOI: https://doi.org/10.1002/dad2.12217.
2. Owens, A.P., et al., Implementing remote memory clinics to enhance clinical care during and after COVID-19. Frontiers in psychiatry, 2020: p. 990 DOI: https://doi.org/10.3389/fpsyt.2020.579934.
3. Feenstra, H.E., et al., Online cognition: factors facilitating reliable online neuropsychological test results. The Clinical Neuropsychologist, 2017. 31(1): p. 59-84 DOI: https://doi.org/10.1080/13854046.2016.1190405.
4. Robillard, J.M., et al., Scientific and ethical features of English-language online tests for Alzheimer’s disease. Alzheimer’s & Dementia: Diagnosis, Assessment & Disease Monitoring, 2015. 1(3): p. 281-288 DOI: https://doi.org/10.1016/j.dadm.2015.03.004.
5. Barnett, J.H., et al., The paired associates learning (PAL) test: 30 years of CANTAB translational neuroscience from laboratory to bedside in dementia research. Translational neuropsychopharmacology, 2015: p. 449-474 DOI: https://doi.org/10.1007/7854_2015_5001.
6. Blackwell, A.D., et al., Detecting dementia: novel neuropsychological markers of preclinical Alzheimer’s disease. Dementia and geriatric cognitive disorders, 2004. 17(1-2): p. 42-48 DOI: https://doi.org/10.1159/000074081.
7. Fowler, K.S., et al., Paired associate performance in the early detection of DAT. Journal of the International Neuropsychological Society, 2002. 8(1): p. 58-71 DOI: https://doi.org/10.1017/S1355617701020069.
8. Égerházi, A., et al., Automated Neuropsychological Test Battery (CANTAB) in mild cognitive impairment and in Alzheimer’s disease. Progress in Neuro-Psychopharmacology and Biological Psychiatry, 2007. 31(3): p. 746-751 DOI: https://doi.org/10.1016/j.pnpbp.2007.01.011.
9. Junkkila, J., et al., Applicability of the CANTAB-PAL computerized memory test in identifying amnestic mild cognitive impairment and Alzheimer’s disease. Dementia and geriatric cognitive disorders, 2012. 34(2): p. 83-89 DOI: https://doi.org/10.1159/000342116.
10. Reijs, B.L., et al., Memory correlates of Alzheimer’s disease cerebrospinal fluid markers: A longitudinal cohort study. Journal of Alzheimer’s Disease, 2017. 60(3): p. 1119-1128 DOI: https://doi.org/10.3233/JAD-160766.
11. Mitchell, J., et al., Outcome in subgroups of mild cognitive impairment (MCI) is highly predictable using a simple algorithm. Journal of neurology, 2009. 256: p. 1500-1509 DOI: https://doi.org/10.1007/s00415-009-5152-0.
12. Pettigrew, C., et al., Computerized paired associate learning performance and imaging biomarkers in older adults without dementia. Brain imaging and behavior, 2022. 16(2): p. 921-929 DOI: https://doi.org/10.1007/s11682-021-00583-9.
13. Soldan, A., et al., Computerized cognitive tests are associated with biomarkers of Alzheimer’s disease in cognitively normal individuals 10 years prior. Journal of the International Neuropsychological Society, 2016. 22(10): p. 968-977 DOI: https://doi.org/10.1017/S1355617716000722.
14. Nathan, P.J., et al., Association between CSF biomarkers, hippocampal volume and cognitive function in patients with amnestic mild cognitive impairment (MCI). Neurobiology of aging, 2017. 53: p. 1-10 DOI: https://doi.org/10.1016/j.neurobiolaging.2017.01.013.
15. Rodrigo, A., et al., Identification of undiagnosed dementia cases using a web-based pre-screening tool: The MOPEAD project. Alzheimer’s & Dementia, 2021. 17(8): p. 1307-1316 DOI: https://doi.org/10.1002/alz.12297.
16. Boada, M., et al., Complementary pre-screening strategies to uncover hidden prodromal and mild Alzheimer’s disease: Results from the MOPEAD project. Alzheimer’s & Dementia, 2022. 18(6): p. 1119-1127 DOI: https://doi.org/10.1002/alz.12441.
17. Backx, R., et al., Comparing web-based and lab-based cognitive assessment using the Cambridge Neuropsychological Test Automated Battery: A within-subjects counterbalanced study. Journal of Medical Internet Research, 2020. 22(8): p. e16792 DOI: https://doi.org/10.2196/16792.
18. Weiner, M.W., et al., The Brain Health Registry: An internet-based platform for recruitment, assessment, and longitudinal monitoring of participants for neuroscience studies. Alzheimer’s & Dementia, 2018. 14(8): p. 1063-1076 DOI: https://doi.org/10.1016/j.jalz.2018.02.021.
19. Watson, J.L., et al., Obstacles and opportunities in Alzheimer’s clinical trial recruitment. Health Affairs, 2014. 33(4): p. 574-579 DOI: https://doi.org/10.1377/hlthaff.2013.1314.
20. Farias, S.T., et al., The measurement of everyday cognition (ECog): scale development and psychometric properties. Neuropsychology, 2008. 22(4): p. 531 DOI: https://doi.org/10.1037/0894-4105.22.4.531.
21. Nosheny, R.L., et al., Online study partner-reported cognitive decline in the Brain Health Registry. Alzheimer’s & Dementia: Translational Research & Clinical Interventions, 2018. 4: p. 565-574.
22. Kroenke, K. and R.L. Spitzer, The PHQ-9: a new depression diagnostic and severity measure. 2002, SLACK Incorporated Thorofare, NJ. p. 509-515 DOI: https://doi.org/10.3928/0048-5713-20020901-06.
23. Kroenke, K., R.L. Spitzer, and J.B. Williams, The PHQ-9: validity of a brief depression severity measure. Journal of general internal medicine, 2001. 16(9): p. 606-613 DOI: https://doi.org/10.1046/j.1525-1497.2001.016009606.x.
24. Team, R.C., R: language and environment for statistical computing. 2017, R Foundation for Statistical Computing: Vienna, Austria.
25. Atske, S. and A. Perrin, Home broadband adoption, computer ownership vary by race, ethnicity in the U.S. 2021, Pew Research Center.
26. Ashford, M.T., et al., Effects of sex, race, ethnicity, and education on online aging research participation. Alzheimer’s & Dementia: Translational Research & Clinical Interventions, 2020. 6(1): p. e12028 DOI: https://doi.org/10.1002/trc2.12028.
27. Mackin, R.S., et al., Unsupervised online neuropsychological test performance for individuals with mild cognitive impairment and dementia: Results from the Brain Health Registry. Alzheimers Dement (Amst), 2018. 10: p. 573-582 DOI: https://doi.org/10.1016/j.dadm.2018.05.005.
28. Banh, T., et al., Unsupervised Performance of the CogState Brief Battery in the Brain Health Registry: Implications for Detecting Cognitive Decline. The journal of prevention of Alzheimer’s disease, 2022: p. 1-7 DOI: https://doi.org/10.14283/jpad.2021.68.
29. Nosheny, R.L., et al., Validation of online functional measures in cognitively impaired older adults. Alzheimer’s & Dementia, 2020 DOI: https://doi.org/10.1002/alz.12138.
30. Hayat, S.A., et al., Cognitive function in a general population of men and women: a cross sectional study in the European Investigation of Cancer–Norfolk cohort (EPIC-Norfolk). BMC geriatrics, 2014. 14: p. 1-16.
31. Abbott, R.A., et al., Normative data from linear and nonlinear quantile regression in CANTAB: Cognition in mid-to-late life in an epidemiological sample. Alzheimer’s & Dementia: Diagnosis, Assessment & Disease Monitoring, 2019. 11: p. 36-44 DOI: https://doi.org/10.1016/j.dadm.2018.10.007.
32. Pauls, F., F. Petermann, and A.C. Lepach, Gender differences in episodic memory and visual working memory including the effects of age. Memory, 2013. 21(7): p. 857-874 DOI: https://doi.org/10.1080/09658211.2013.765892.
33. Morrow, L.A., et al., High medical co-morbidity and family history of dementia is associated with lower cognitive function in older patients. Family Practice, 2009. 26(5): p. 339-343 DOI: https://doi.org/10.1093/fampra/cmp047.
34. Chandler, J.M., et al., P3-111: Cognitive assessment: Discrimination of impairment and detection of decline in Alzheimer’s disease and mild cognitive impairment. Alzheimer’s & Dementia, 2008. 4: p. T551-T552 DOI: https://doi.org/10.1016/j.jalz.2008.05.1676.
35. Stricker, N.H., et al., Diagnostic and prognostic accuracy of the cogstate brief battery and auditory verbal learning test in preclinical Alzheimer’s disease and incident mild cognitive impairment: implications for defining subtle objective cognitive impairment. Journal of Alzheimer’s Disease, 2020. 76(1): p. 261-274 DOI: https://doi.org/10.3233/JAD-200087.
36. Lewis, J.R., The system usability scale: past, present, and future. International Journal of Human–Computer Interaction, 2018. 34(7): p. 577-590 DOI: https://doi.org/10.1080/10447318.2018.1455307.
© The Authors 2023